Machine learning-guided discovery of ionic polymer electrolytes for lithium metal batteries (vol 14, 2789, 2023)
Research Square (Research Square)(2023)
摘要
Abstract Development of ionic polymer electrolytes (IPEs) without flammable organics is a critical strategy to enable safe and high-energy lithium metal batteries (LMBs). As critical components in IPEs, ionic liquids (ILs) with high ionic conductivity and wide electrochemical window are promising candidates to enable LMBs. Here, we describe a fast and robust machine learning workflow embedded with quantum chemistry calculation and graph convolutional neural network to discover promising ILs for IPEs. By selecting subsets of the recommended ILs, combining with a rigid-rod polyelectrolyte and a predetermined lithium salt, we develop a class of large area and mechanically strong IPE membranes with thickness ~ 50 μm. The symmetric cells exhibit stable cycling performance at 1 mA cm-1 (0.5 mAh cm-2) up to 800 h at room temperature (RT) and excellent reversibility at 6 mA cm-2 (3 mAh cm-2) at 80 °C. With LiFePO4 loading ~ 10.3 mg cm-2, the full cells deliver outstanding capacity retention for > 350 cycles (> 96% with 0.5 C at RT; > 80% with 2 C at 50 °C), fast charge/discharge capability (146 mAh g-1 with 5 C at 80 °C) and ultrahigh coulombic efficiency (> 99.92%). This performance is rarely reported by any single-layer polymer electrolytes without any organic plasticizers/oligomers for LMBs.
更多查看译文
关键词
ionic polymer,lithium,electrolytes,discovery,learning-guided
AI 理解论文
溯源树
样例
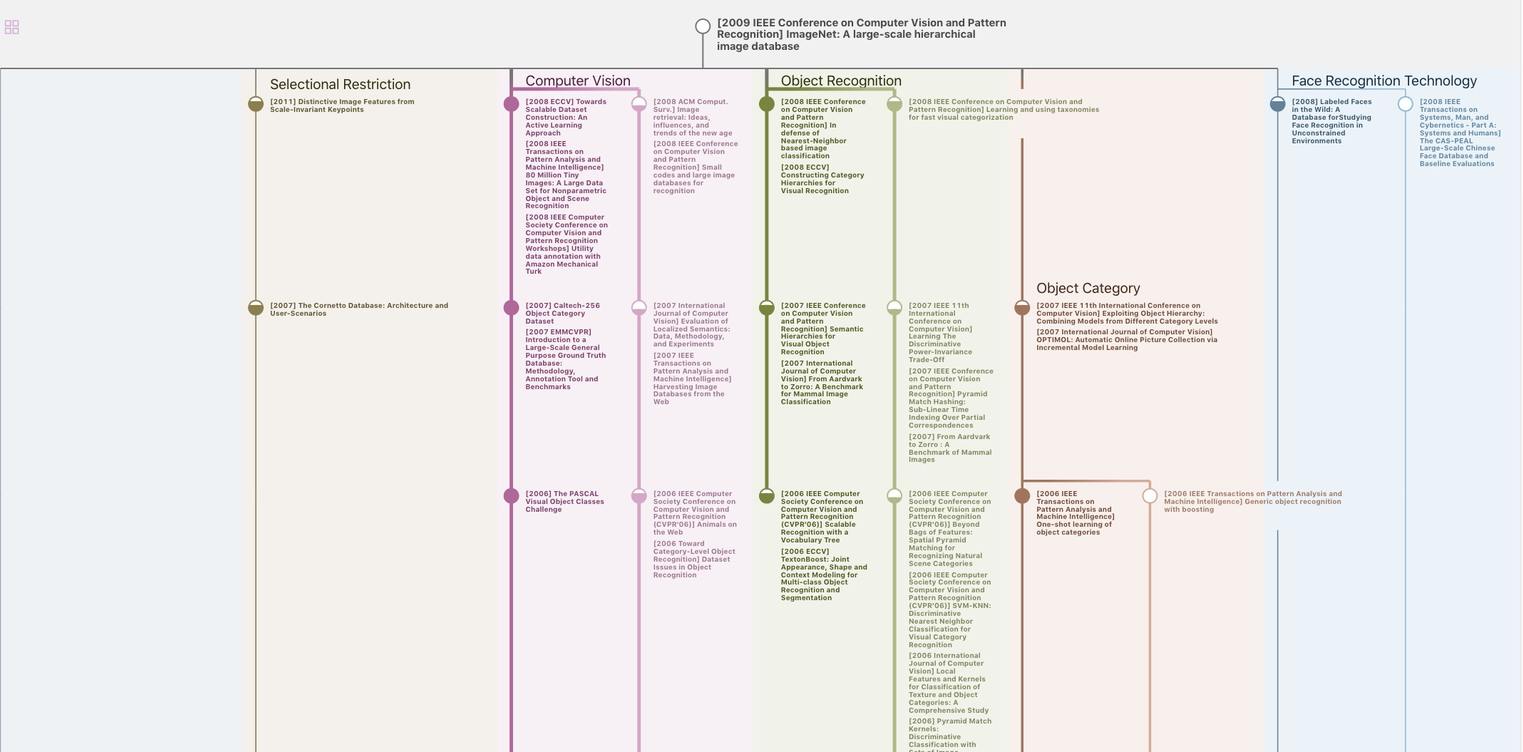
生成溯源树,研究论文发展脉络
Chat Paper
正在生成论文摘要