Social Image-Text Sentiment Classification With Cross-Modal Consistency and Knowledge Distillation
IEEE TRANSACTIONS ON AFFECTIVE COMPUTING(2023)
摘要
Social media sentiment analysis, which aims to evaluate the attitudes of online users based on their posts, has attracted significant research attention due to its successful application in the field of social media monitoring. It is a beneficial way to utilize multimodal information uploaded by users in order to improve sentiment classification ability. However, existing multimodal fusion-based approaches continue to face difficulties due to the issues of between-modality semantic inconsistency and missing modality. To address these issues, we propose a cross-modal consistency modeling-based knowledge distillation framework for image-text sentiment classification of social media data. Specifically, we design a hybrid curriculum learning strategy to measure the semantic consistency of multimodal data, then gradually train all image-text pairs from easy to hard, which can effectively handle the massive amounts of noise caused by inconsistencies between image and text data on social media. Moreover, in order to alleviate the problem of missing images in unimodal posts, we propose a privileged feature distillation method, in which the teacher model additionally considers images as privileged features, to transfer the visual knowledge to the student model, thereby enhancing the accuracy for text sentiment classification. Extensive experiments conducted over three real-world social media datasets demonstrate the effectiveness and superiority of the proposed multimodal sentiment analysis model.
更多查看译文
关键词
Multimodal sentiment classification,cross-modal consistency,curriculum learning,privileged feature,knowledge distillation
AI 理解论文
溯源树
样例
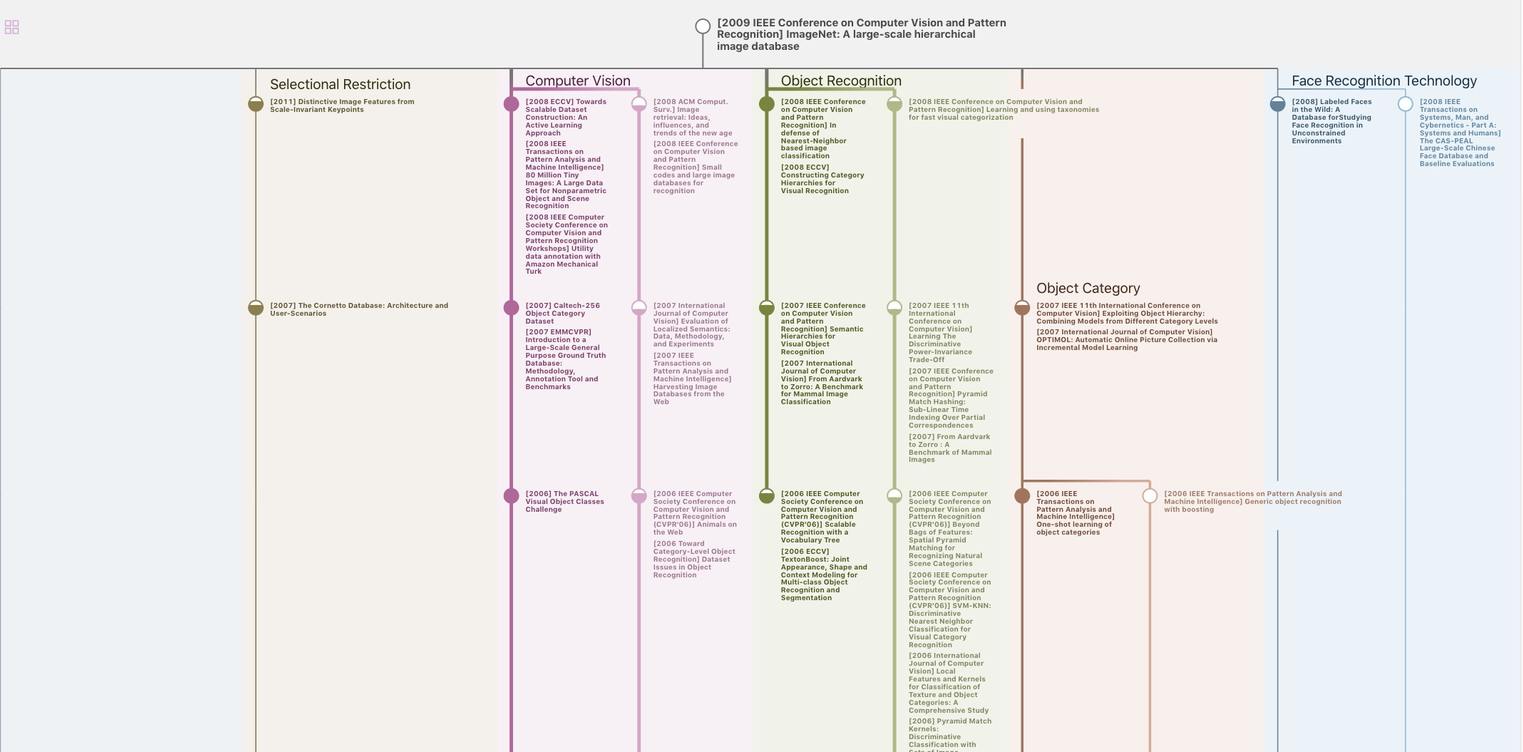
生成溯源树,研究论文发展脉络
Chat Paper
正在生成论文摘要