Target Detection Based on Two-Stream Convolution Neural Network With Self-Powered Sensors Information
IEEE Sensors Journal(2023)
摘要
With the rapid development of artificial intelligence, a neural network is widely used in various fields. The target detection algorithm is mainly based on a neural network, but the accuracy of the target detection algorithm is greatly related to the complexity of the scene and texture. A target detection algorithm based on the RGB-D image from the perspective of the lightweight of the target detection network model and the integration of the depth map to overcome the weak environmental illumination with self-powered sensors information is proposed. This article analyzes the network model structure of YOLOv4 and MobileNet, compares the variation of parameter numbers between depthwise separable convolution and convolutional neural networks, and combines the advantages of the YOLOv4 network and the MobileNetv3 network. The main network of three effective feature layers in YOLOv4 is replaced by the MobileNetv3 network for initial feature layer extraction to strengthen the feature extraction network. At the same time, the standard convolution models in the network are replaced by depthwise separable convolution. The proposed method is compared with YOLOv4 and YOLOv4-MobileNetv3 in this article, and the experimental results show that the proposed network retains its original advantages in accuracy, but the size of the network model is about 23% of that of the YOLOv4 network model, the processing speed is about 42% higher than that of the YOLOv4 network model, and the detection accuracy can still reach 83% in the environment with poor lighting conditions.
更多查看译文
关键词
Depthwise separable convolution,MobileNet,self-powered sensors information,target detection,two-stream convolutional neural network (CNN),YOLOv4
AI 理解论文
溯源树
样例
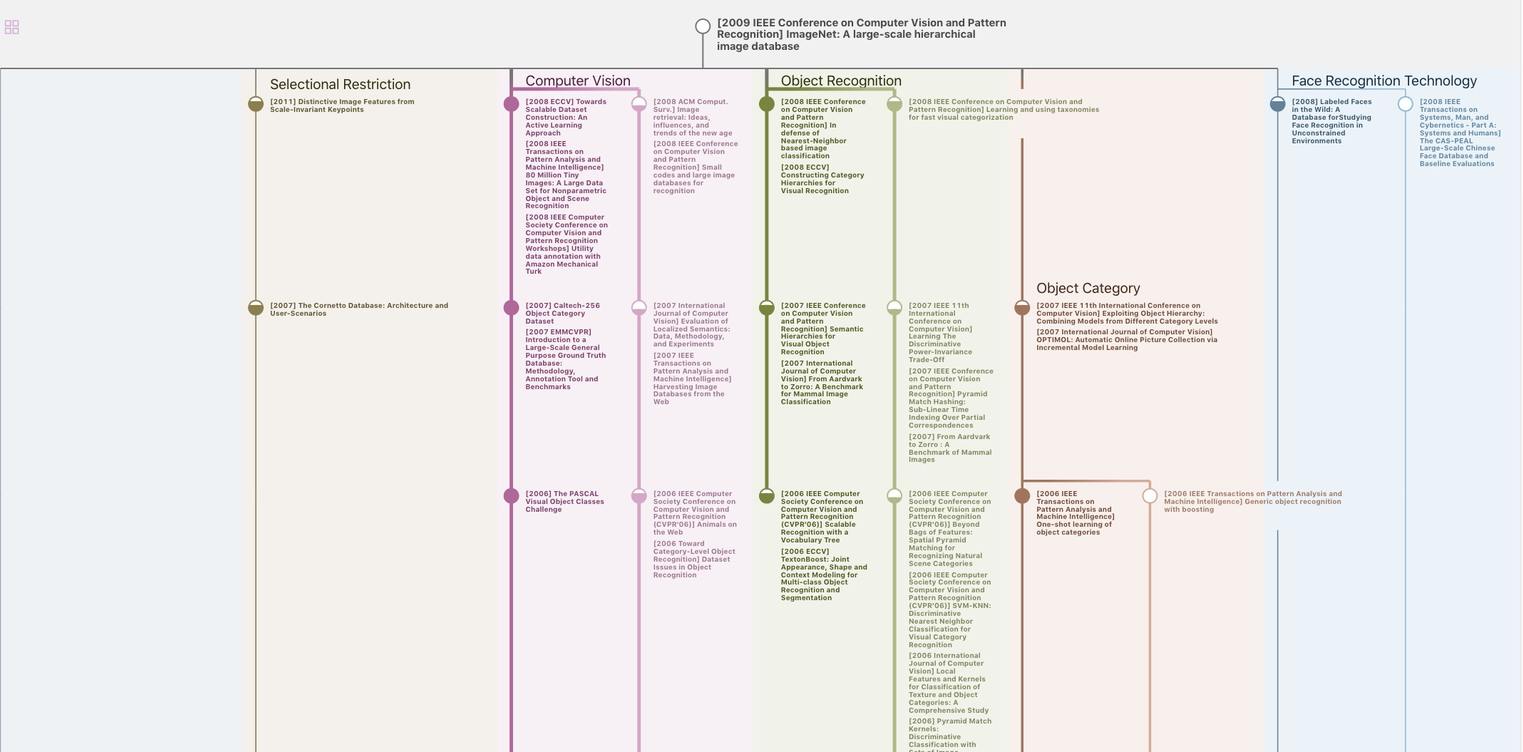
生成溯源树,研究论文发展脉络
Chat Paper
正在生成论文摘要