Time-variant gain in noise but not signal in drift-diffusion model leads to slower error decisions
crossref(2023)
摘要
Sequential sampling based mathematical models of decision-making involve evidence accumulation over time during decision formation and have been successful in capturing choice behaviour. A popular model is the drift-diffusion model (DDM). To capture finer aspects of choice behaviour, such as differences in choice reaction times, time-variant gain features that imitate urgency signals have been implemented in standard DDM. In particular, a previous DDM with a time-variant gain can replicate slower error decisions than correct decisions. However, the time-variant gain of the model, and other similar time-variant gain-based models, often implement time-variant gain on both signal and noise features, with the assumption that increasing gain on the drift rate (due to urgency) is similar to DDM with collapsing decision bounds. Hence, it is unclear whether the gain effects on just the signal or noise feature can lead to different choice behaviour. Here, using computational simulations and theoretical approximations, we used a previous time-variant gain DDM and systemically showed that time-variant gain only on the DDM’s noise suffices to produce slower error choices while time-variant gain only on the drift rate speeds up error choices. In addition, the former was associated with more skewness and kurtosis on correct RT distributions while the latter led to more skewness and kurtosis in error RT distributions. Thus, time-variant gain in DDM’s different components can lead to different choice outcomes, which can potentially be used for more systematic data fitting.
更多查看译文
AI 理解论文
溯源树
样例
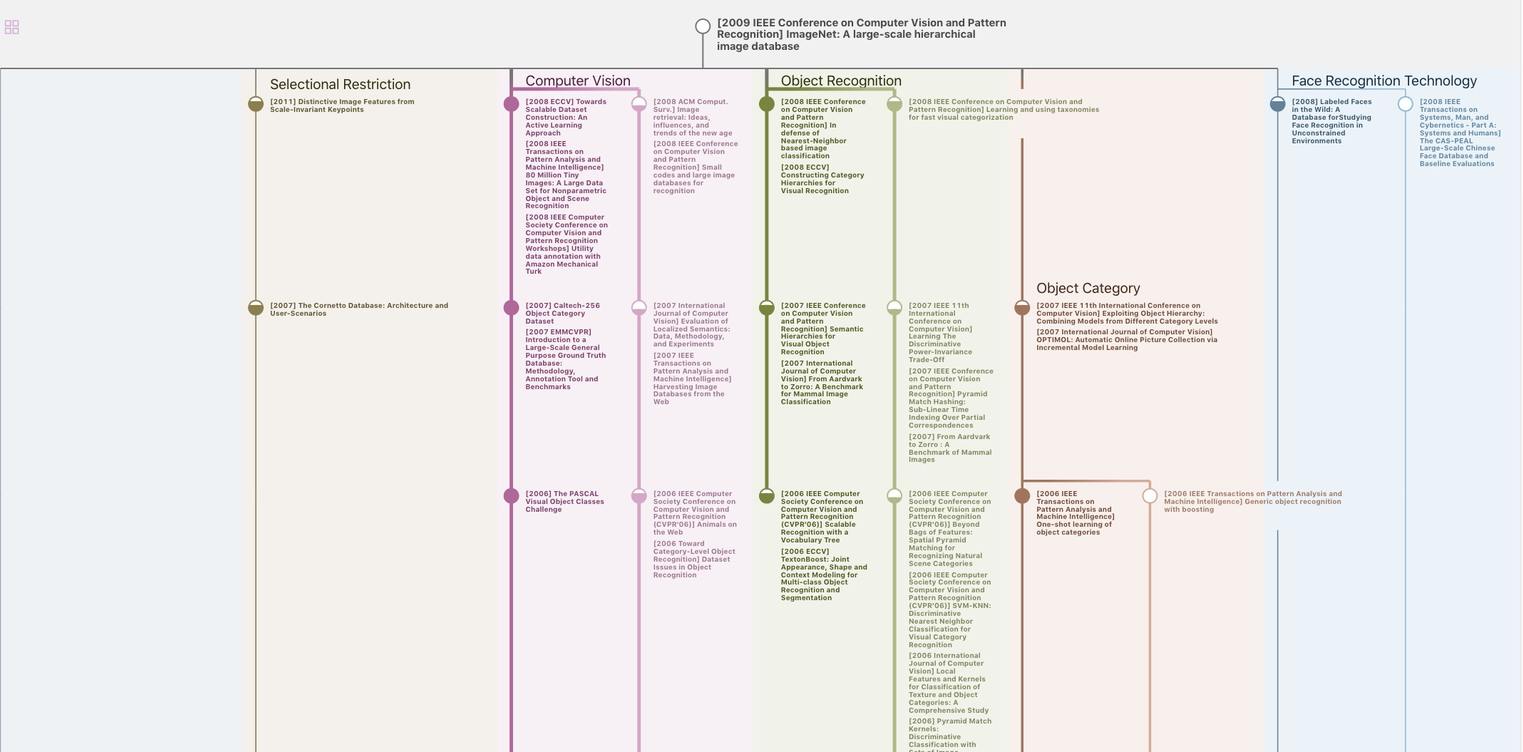
生成溯源树,研究论文发展脉络
Chat Paper
正在生成论文摘要