Blockchain-Based Federated Learning: A Systematic Survey
IEEE NETWORK(2023)
摘要
Federated Learning (FL) is a distributed machine learning paradigm that trains models across multiple devices without exchanging users ' data, thereby providing stronger data privacy guarantees. However, some research reveals that FL may face security and privacy issues, such as single point of failure, model poisoning, and parameter privacy disclosure. Recently, the field of combining blockchain and FL has a trend to become a hot research topic. More and more researchers attempt to use blockchain to decentralize and secure FL frameworks. To further understand recent advances in blockchain- based FL (BFL) systems, this article aims to provide a systematic survey to deconstruct BFL systems. We propose a taxonomy of BFL systems following the lifecycle of FL tasks and divide them into three layers, i. e., the blockchain layer, the training layer, and the aggregation layer. We review and summarize representative work in each layer. We also discuss several open challenges for designing more secure and efficient BFL systems.
更多查看译文
AI 理解论文
溯源树
样例
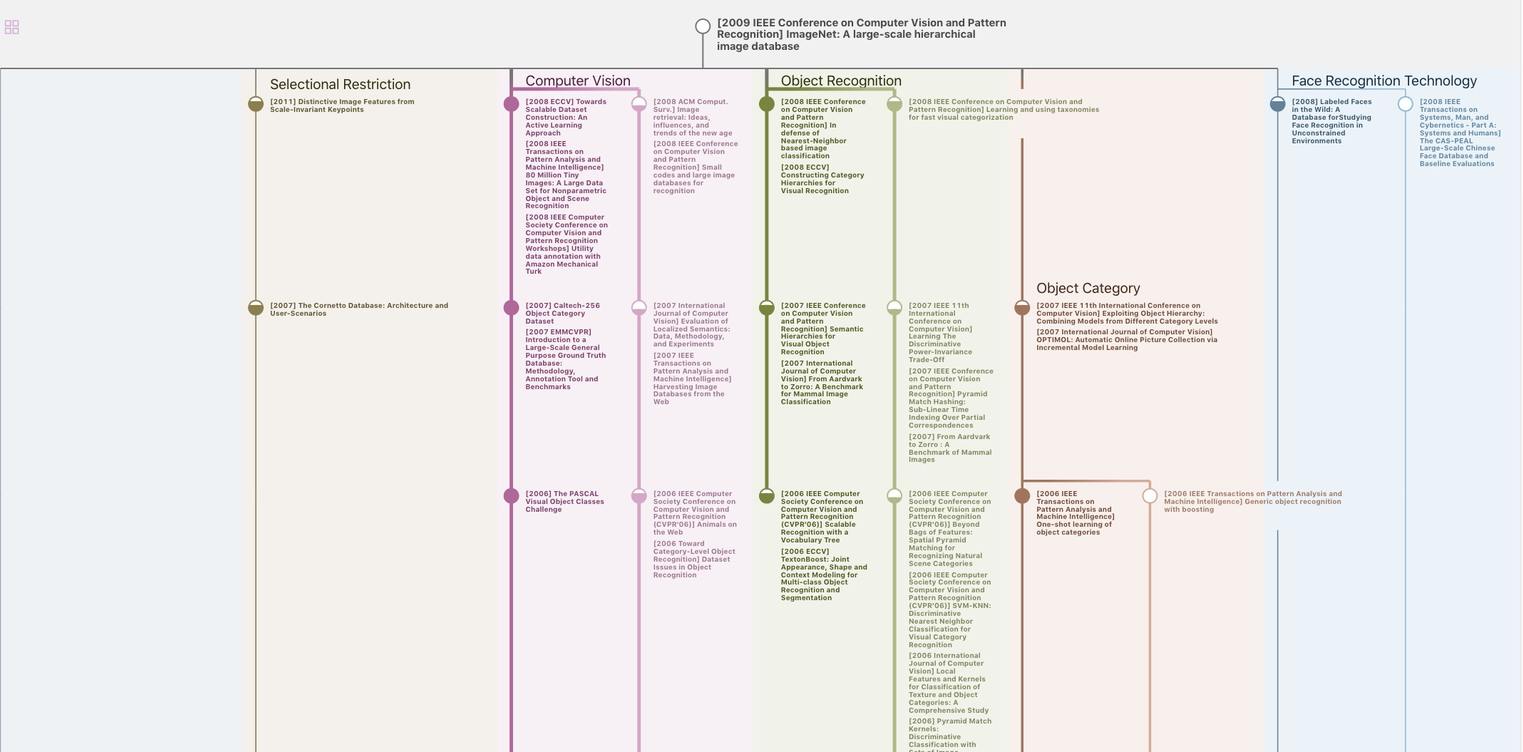
生成溯源树,研究论文发展脉络
Chat Paper
正在生成论文摘要