EUNNet: Efficient UN-Normalized Convolution Layer for Stable Training of Deep Residual Networks Without Batch Normalization Layer
IEEE Access(2023)
摘要
Batch Normalization (BN) is an essential component of the Deep Neural Networks (DNNs) architectures. It helps improve stability, convergence, and generalization. However, studies are showing that BN might introduce several concerns. Although there are methods for training DNNs without BN using proper weight initialization, they require several learnable scalars or accurate fine-tuning to the training hyperparameters. As a result, in this study, we aim to stabilize the training process of un-normalized networks without using proper weight initialization and to minimize the hyperparameters fine-tuning step. We propose EUNConv, an Efficient UN-normalized Convolutional layer, which helps train un-normalized Deep Residual Networks (ResNets) by using hyperparameters of the normalized networks. Furthermore, we introduce Efficient UN-normalized Neural Network (EUNNet), which replaces all of the conventional convolutional layers of ResNets with our proposed EUNConv. Experimental results show that the proposed EUNNet achieves the same or even better performance than previous methods in various tasks: image recognition, object detection, and segmentation. In particular, EUNNet requires less fine-tuning and less sensitivity to hyperparameters than previous methods.
更多查看译文
关键词
Deep neural networks,batch normalization,overfitting,computer vision,image classification,object detection
AI 理解论文
溯源树
样例
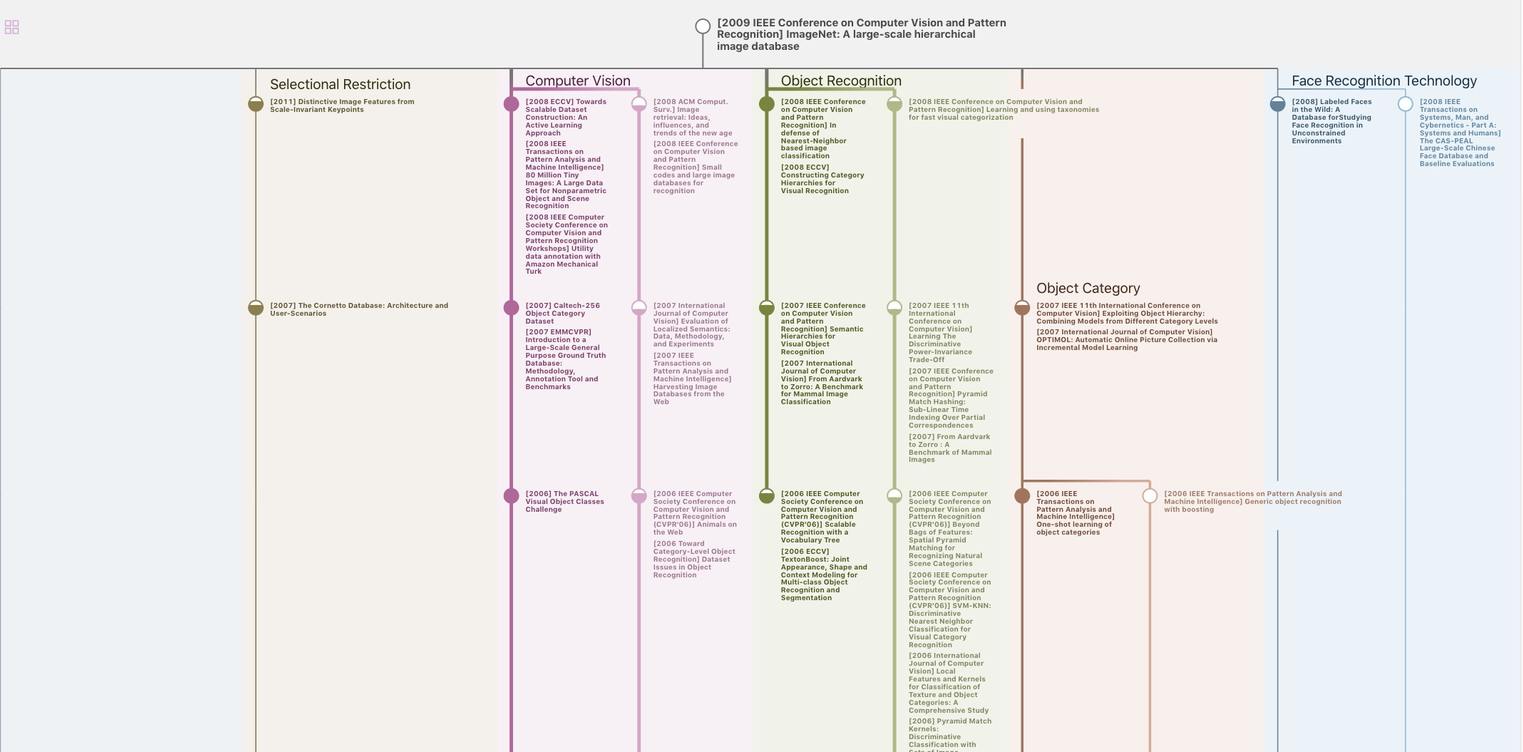
生成溯源树,研究论文发展脉络
Chat Paper
正在生成论文摘要