Hybrid Forecasting for Functional Time Series of Dissolved Oxygen Profiles
Data Science in Science(2023)
摘要
Hybridizing machine learning (ML) and traditional statistical modeling is an active area of research, with evidence that integrating the two approaches may improve model performance. In lake ecology, exploring such models is necessary because recent research shows that traditional hydrodynamic models often produce poor short-term forecasts. Thus, in this paper, we compare a selection of hybrid, ML, and statistical models in functional forecasting of dissolved oxygen (DO) profiles in a lake. Functional data have a unique structure wherein the observations are functions, and several ML models for functional data have been recently proposed. The hybrid models in this paper first obtain functional principal components (FPCs) to reduce the dimension, and FPC scores are then forecast using a feed-forward neural network (NN), a recurrent NN, or a random forest (RF). Purely ML NN and RF models forecast each measurement in the functions independently. A functional-statistical model and the persistence model are provided for reference. The forecast performance of these seven models is compared, and prediction bands are built using a subset of the training data to estimate the prediction uncertainty. The RF-based models forecast the best, and the prediction bands of all models provide good average coverage.
更多查看译文
关键词
dissolved oxygen profiles,hybrid forecasting,functional time series
AI 理解论文
溯源树
样例
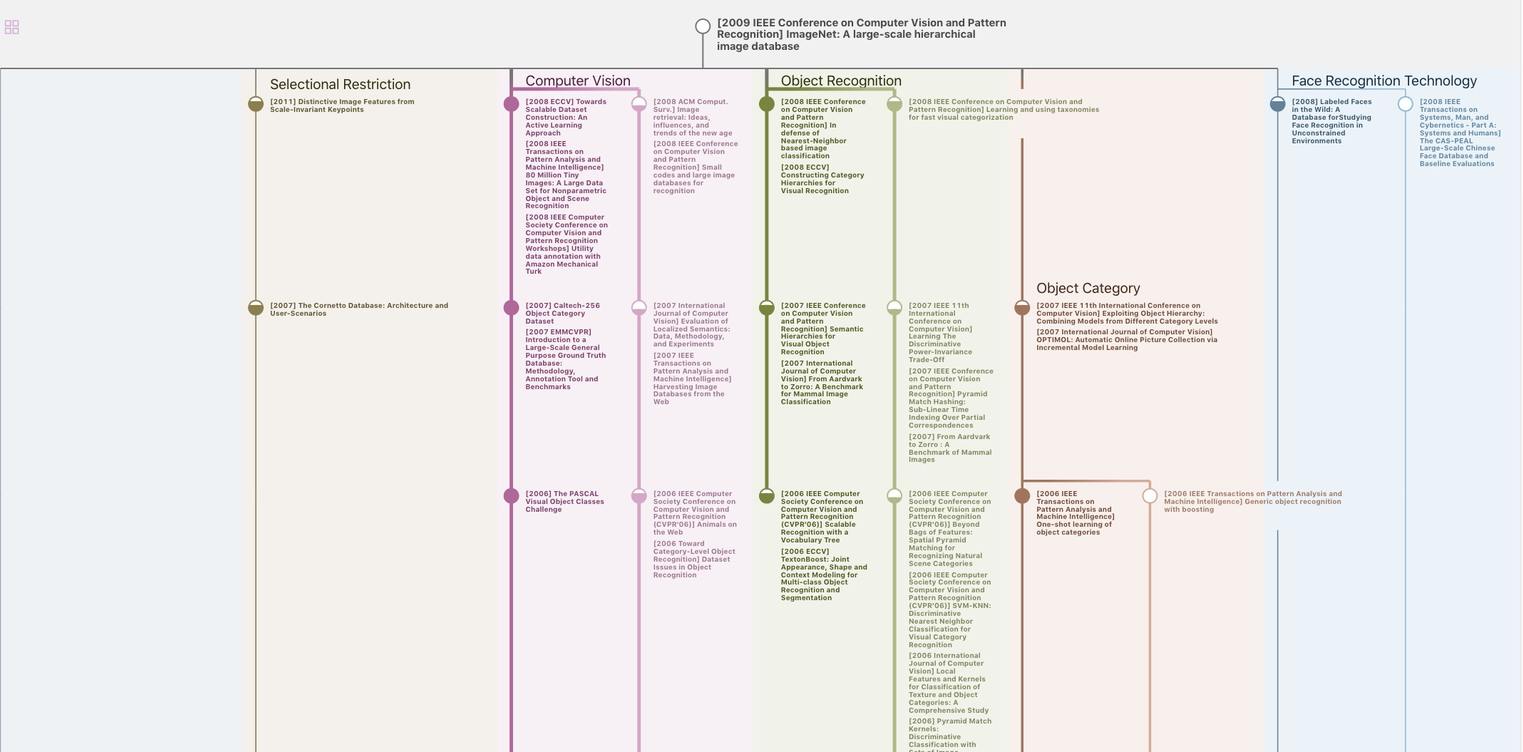
生成溯源树,研究论文发展脉络
Chat Paper
正在生成论文摘要