Analyzing and forecasting pediatric fever clinic visits in high frequency using ensemble time series methods during the COVID-19 pandemic: A case study in Hangzhou, China (Preprint)
crossref(2023)
摘要
BACKGROUND Since the outbreak of COVID-19, Chinese hospitals have widely established independent 24-hour fever clinics to face challenges in nosocomial infections. Forecasting outpatient’s hourly visits can help hospital managers make resource scheduling and planning preliminarily. However, due to the particularity of children's treatment behavior in epidemic context, the fever clinic hourly visits of children's hospital which manifests as a long sequence time series in high-frequency, requires a model suitable for its data properties to implement forecasting rather than simply applying existing methods. OBJECTIVE Our aim is to select models fitting the patterns and statistical characteristics of the time series while having good generalization and robustness in pediatric fever clinic hourly visits forecasting. METHODS We collected hourly data on fever clinic visits from 2020.1.23 to 2022.9.23 in a large public children's hospital in China. Exploratory analysis was firstly conducted on the time series data to reveal the multi-seasonality and nonlinearity of it. Then, single and hybrid models were respectively built and validated on the retrospective data to generate hourly visits forecast. Based on the analysis results, SARIMA, STLF and NNAR were preselected as single models, and the multiple model combinations were made up as hybrid forecasting models. For evaluation, RMSE, MAE, MASE and RMSSE were used as accuracy measurements. And cross-validation of rolling forecasting origin was implemented for performance comparison among models over different forecast horizons. RESULTS A novel model combination -- the Hybrid NNAR-STLF, was founded and proved effective in our forecast task. In 15-days forecast, the model achieved the best performance in all accuracy metrics (with RMSE=17.4, MAE=12.1, MASE=1.43, RMSSE=1.43). It also shows an overall advantage on all the forecast horizons (from 1-day ahead to 30-days ahead). CONCLUSIONS Our exploratory analysis fully reveals the underlying characteristics of time series data, which is of significant help in the succeeding model selection step. Meanwhile, it also verifies methodological validity of our case study. Although forecast accuracy of time series tends to decline with the forecast horizon increases, the Hybrid NNAR-STLF model is applicable in short-, medium- and long-term forecasts due to its fitting ability of multi-seasonality captured by the STLF component and nonlinear characteristics captured by the NNAR component. In future work, external variables characterizing moving events and climate factors can be introduced to acquire better accuracy.
更多查看译文
AI 理解论文
溯源树
样例
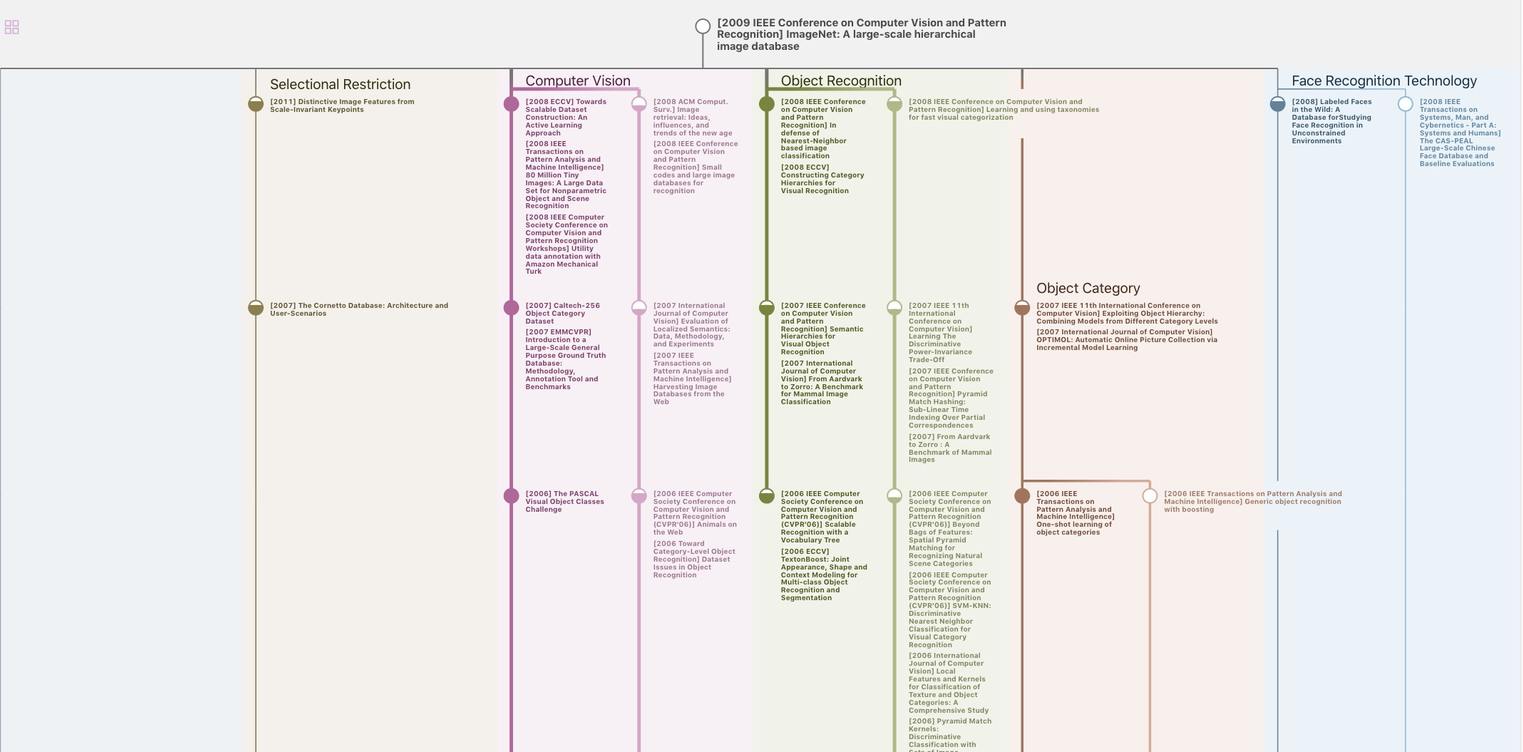
生成溯源树,研究论文发展脉络
Chat Paper
正在生成论文摘要