Precise Real-Time Doppler Positioning Using a Stand-Alone GNSS Receiver
Proceedings of the 2023 International Technical Meeting of The Institute of NavigationThe International Technical Meeting of the The Institute of Navigation(2023)
摘要
Doppler shift is an essential measurement within a GNSS receiver, which reflects the relative velocity between the transmitter and the receiver. Therefore, the Doppler measurement provides an alternative approach to the Position, Velocity, Timing (PVT) solution, which is commonly termed Doppler positioning. In this way, Doppler positioning can serve as a complement to conventional pseudorange positioning. Besides, Doppler positioning is also an essential component of LEO satellite navigation and anti-spoofing schemes. Therefore, researches for precise, real-time, and wide applicability Doppler positioning algorithms have been gradually attached importance. However, the practicality of existing methods is limited, due to the shortcomings in terms of accuracy performance and real-time use. To this end, a precise real-time Doppler positioning technique is investigated in this paper, using a stand-alone GNSS receiver. Inspired by the superiority of real-time use, modifying the conventional Least Squares (LS) based approach is the basic idea of our work to improve the Doppler positioning performance. Both the position and velocity results can be derived from the LS-based Doppler positioning technique, meanwhile, the velocity solution has much higher accuracy than the position, which will be demonstrated in this paper. Thereby, the improvement on positioning accuracy is achieved by exploiting the velocity result to smooth the original position result, which is directly computed from the LS-based approach. In particular, a Position-Domain (PD) filter is implemented for the fusion of position and velocity. Compared with a Kalman Filter (KF), the PD filter is capable of freeing the user from the inconvenience of selecting an appropriate model and initial value, as the choice of which will dramatically influence the performance of a KF. A basic demonstration of the validity and enhancement of our work is conducted with two field tests. Compared with the LS-based approach, the Root-Mean-Square Error (RMSE) is reduced from 5km to 28.96m in the dynamic scenario, and from 4km to 21.82m in the static scenario.
更多查看译文
关键词
positioning,real-time,stand-alone
AI 理解论文
溯源树
样例
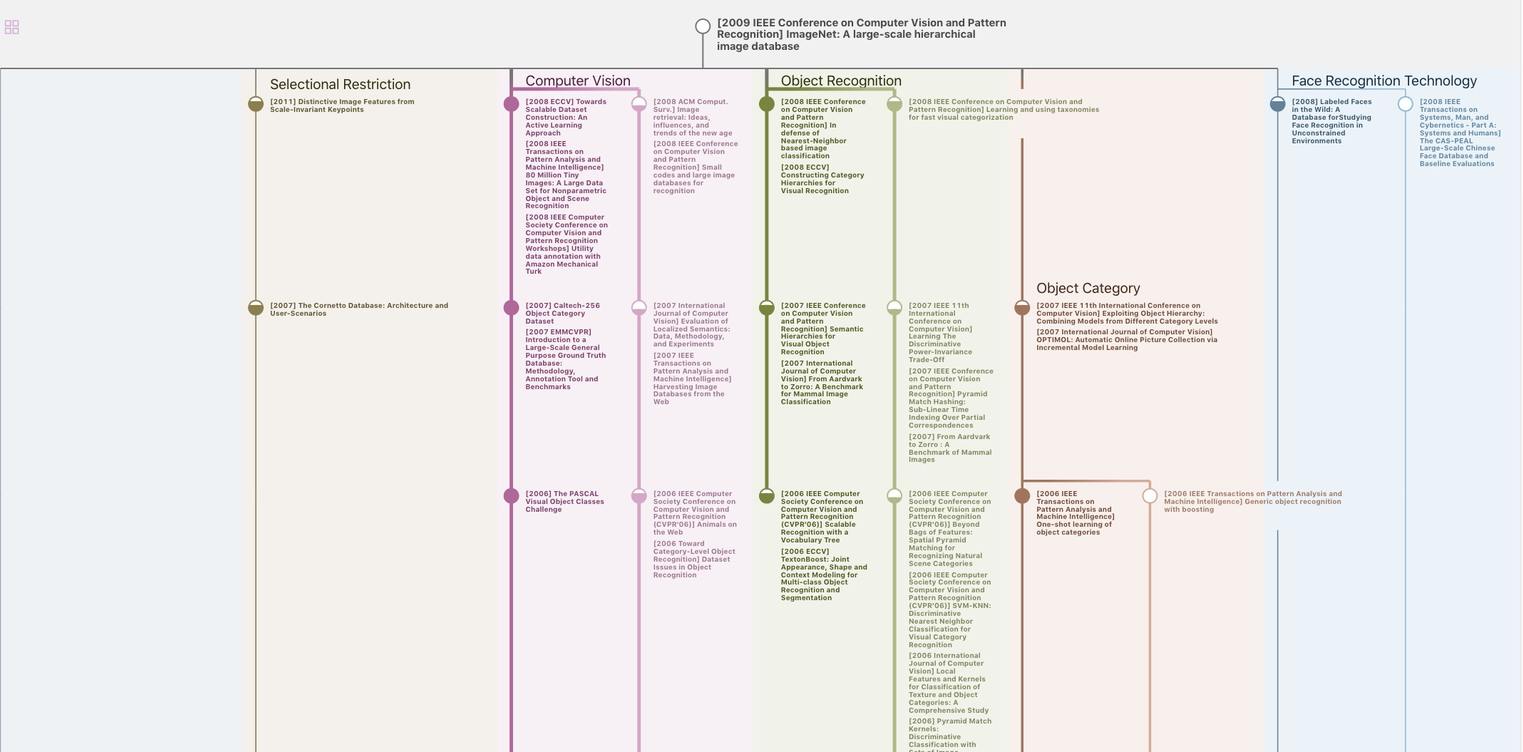
生成溯源树,研究论文发展脉络
Chat Paper
正在生成论文摘要