Statistical learning across passive listening adjusts perceptual weights of speech input dimensions
Cognition(2023)
摘要
Statistical learning across passive exposure has been theoretically situated with unsupervised learning. However, when input statistics accumulate over established representations – like speech syllables, for example – there is the possibility that prediction derived from activation of rich, existing representations may support error-driven learning. Here, across five experiments, we present evidence for error-driven learning across passive speech listening. Young adults passively listened to a string of eight beer - pier speech tokens with distributional regularities following either a canonical American-English acoustic dimension correlation or a correlation reversed to create an accent. A sequence-final test stimulus assayed the perceptual weight – the effectiveness – of the secondary dimension in signaling category membership as a function of preceding sequence regularities. Perceptual weight flexibly adjusted according to the passively experienced regularities even when the preceding regularities shifted on a trial-by-trial basis. The findings align with a theoretical view that activation of established internal representations can support learning across statistical regularities via error-driven learning. At the broadest level, this suggests that not all statistical learning need be unsupervised. Moreover, these findings help to account for how cognitive systems may accommodate competing demands for flexibility and stability: instead of overwriting existing representations when short-term input distributions depart from the norms, the mapping from input to category representations may be dynamically – and rapidly – adjusted via error-driven learning from predictions derived from internal representations.
更多查看译文
关键词
Statistical learning,Perceptual weight,Speech categorization,Dimension-based statistical learning
AI 理解论文
溯源树
样例
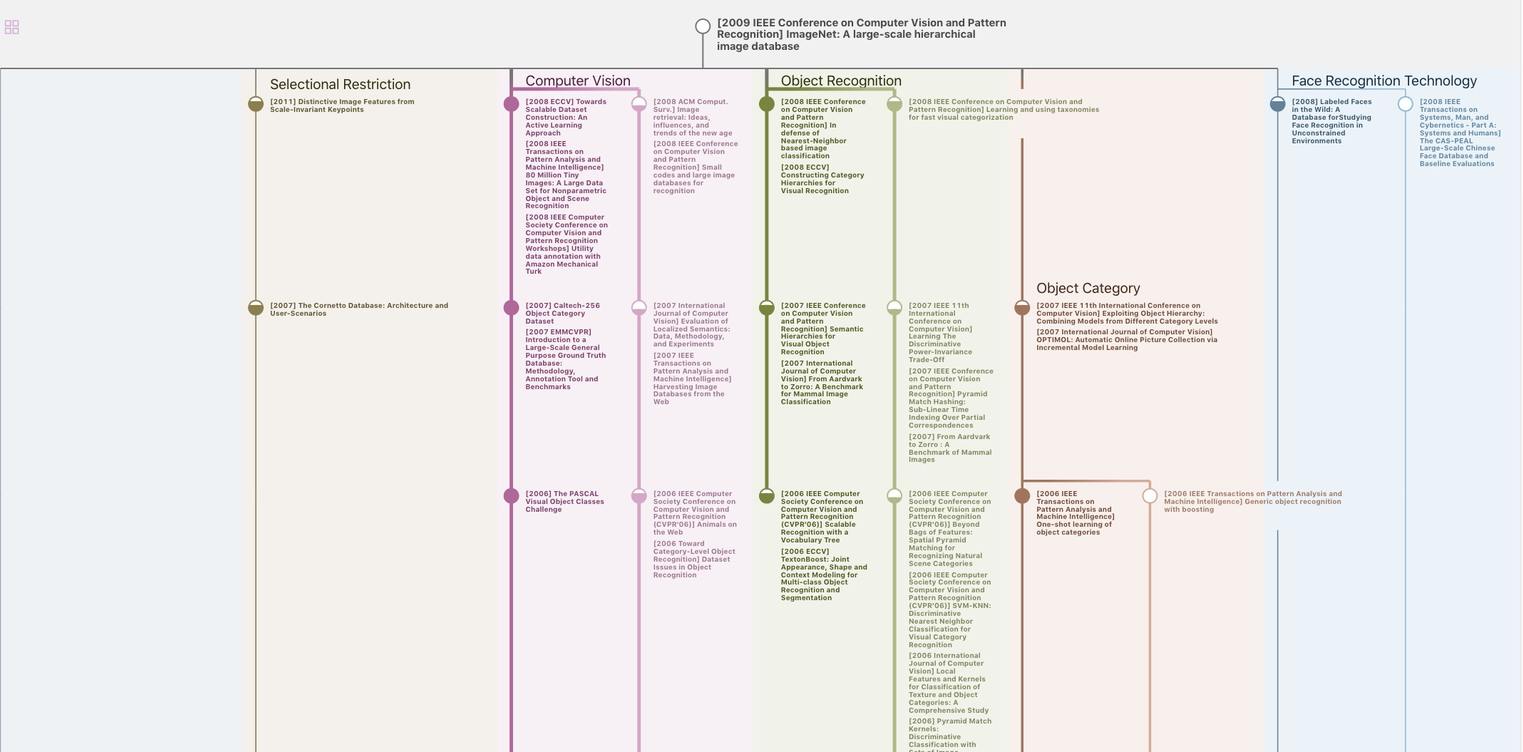
生成溯源树,研究论文发展脉络
Chat Paper
正在生成论文摘要