IA-FaceS: A bidirectional method for semantic face editing
Neural Networks(2023)
摘要
Semantic face editing has achieved substantial progress in recent years. However, existing face editing methods, which often encode the entire image into a single code, still have difficulty in enabling flexible editing while keeping high-fidelity reconstruction. The one-code scheme also brings entangled face manipulations and limited flexibility in editing face components. In this paper, we present IA-FaceS, a bidirectional method for disentangled face attribute manipulation as well as flexible, controllable component editing. We propose to embed images onto two branches: one branch computes high-dimensional component-invariant content embedding for capturing face details, and the other provides low-dimensional component-specific embeddings for component manipulations. The two-branch scheme naturally enables high-quality facial component-level editing while keeping faithful reconstruction with details. Moreover, we devise a component adaptive modulation (CAM) module, which integrates component-specific guidance into the decoder and successfully disentangles highly-correlated face components. The single-eye editing is developed for the first time without editing face masks or sketches. According to the experimental results, IA-FaceS establishes a good balance between maintaining image details and performing flexible face manipulation. Both quanti-tative and qualitative results indicate that the proposed method outperforms the existing methods in reconstruction, face attribute manipulation, and component transfer. We release the code and weights at: https://github.com/CMACH508/IA-FaceS.(c) 2022 Elsevier Ltd. All rights reserved.
更多查看译文
关键词
Deep bidirectional method,Disentangled attribute manipulation,Facial component editing
AI 理解论文
溯源树
样例
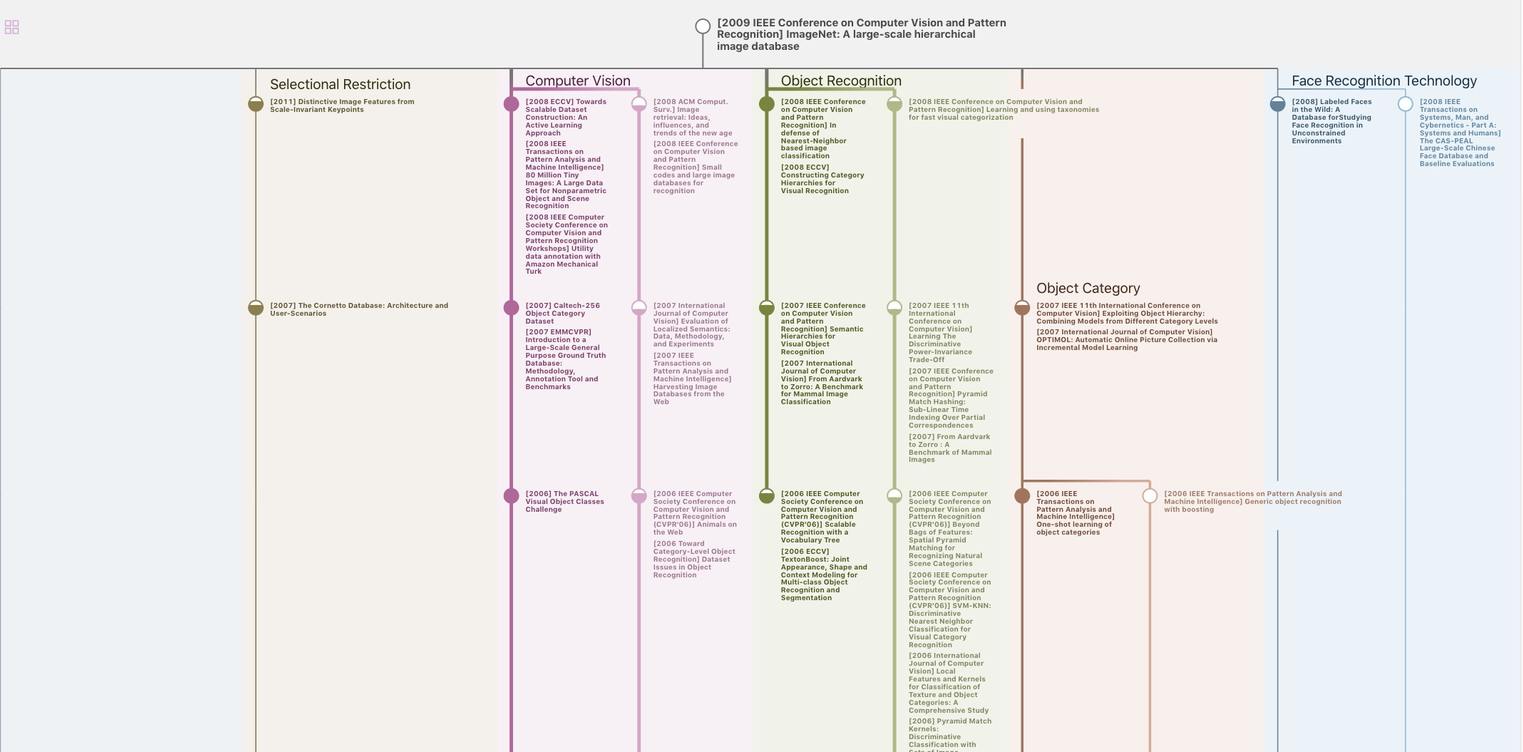
生成溯源树,研究论文发展脉络
Chat Paper
正在生成论文摘要