Inference of astrophysical parameters with a conditional invertible neural network
Journal of Physics: Conference Series(2023)
摘要
Conditional Invertible Neural Networks (cINNs) provide a new technique for the inference of free model parameters by enabling the creation of posterior distributions. With these distributions, the mean parameter values, their uncertainties and the correlations between the parameters can be estimated. In this contribution, we summarize the functionality of cINNs, which are based on normalizing flows, and present the application of this new method to a scenario from astroparticle physics. We show that it is possible to constrain properties of the currently unknown sources of ultra-high-energy cosmic rays and compare the posterior distributions obtained with the network to those acquired using the classic Markov Chain Monte Carlo method.
更多查看译文
关键词
conditional invertible neural network,astrophysical parameters,neural network,inference
AI 理解论文
溯源树
样例
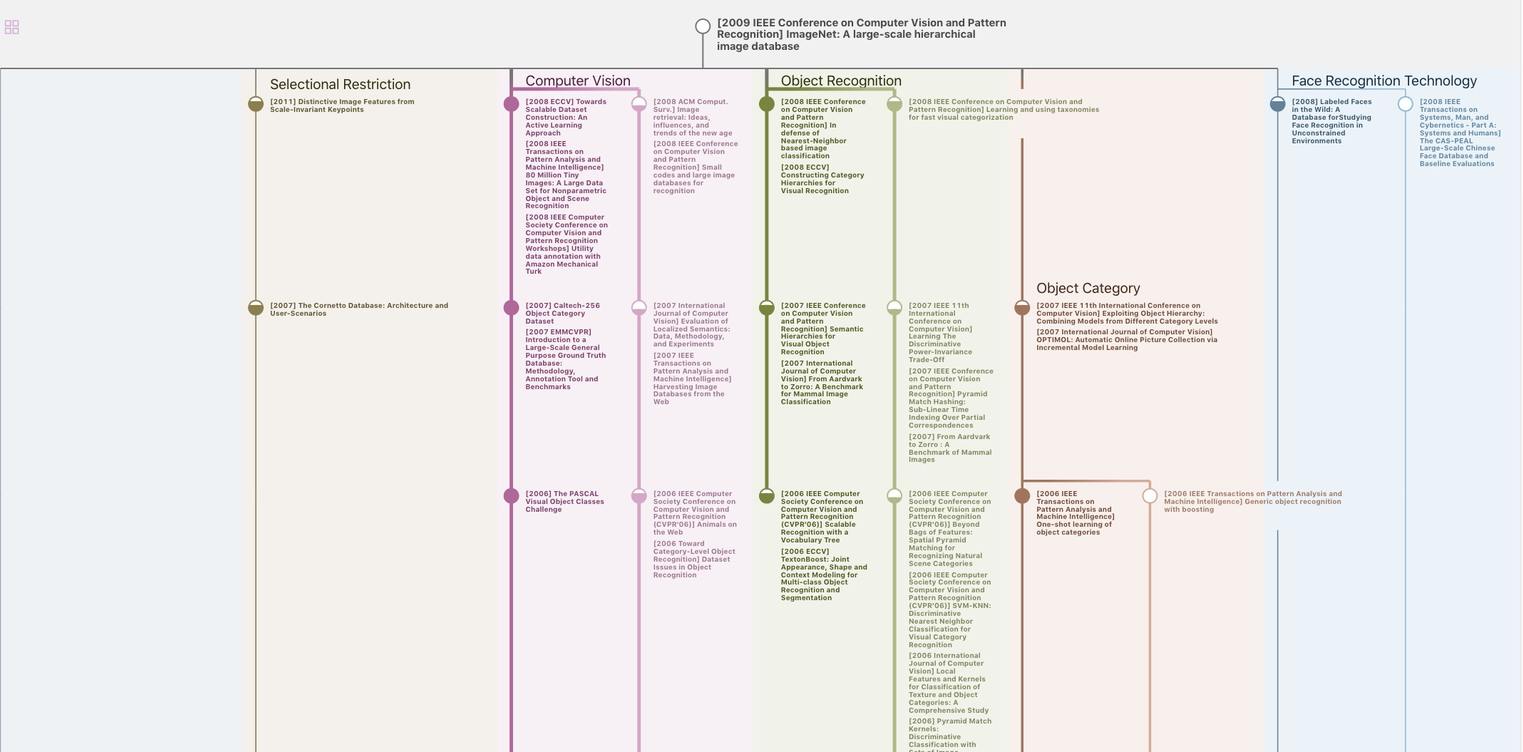
生成溯源树,研究论文发展脉络
Chat Paper
正在生成论文摘要