CDAnet: A Physics‐Informed Deep Neural Network for Downscaling Fluid Flows
Journal of Advances in Modeling Earth Systems(2022)
摘要
Generating high-resolution flow fields is of paramount importance for various applications in engineering and climate sciences. This is typically achieved by solving the governing dynamical equations on high-resolution meshes, suitably nudged toward available coarse-scale data. To alleviate the computational cost of such downscaling process, we develop a physics-informed deep neural network (PI-DNN) that mimics the mapping of coarse-scale information into their fine-scale counterparts of continuous data assimilation (CDA). Specifically, the PI-DNN is trained within the theoretical framework described by Foias et al. (2014, ) to generate a surrogate of the theorized determining form map from the coarse-resolution data to the fine-resolution solution. We demonstrate the PI-DNN methodology through application to 2D Rayleigh-Benard convection, and assess its performance by contrasting its predictions against those obtained by dynamical downscaling using CDA. The analysis suggests that the surrogate is constrained by similar conditions, in terms of spatio-temporal resolution of the input, as the ones required by the theoretical determining form map. The numerical results also suggest that the surrogate's downscaled fields are of comparable accuracy to those obtained by dynamically downscaling using CDA. Consistent with the analysis of Farhat, Jolly, and Titi (2015, ), temperature observations are not needed for the PI-DNN to predict the fine-scale velocity, pressure and temperature fields. Plain Language Summary Detailed descriptions of the solution of dynamical systems are essential for a comprehensive understanding of their behavior. Obtaining sufficiently fine solutions is, however, computationally expensive. Mathematical tools have been developed to drive the solution of dynamical systems toward the reference solution, from which only coarse-scale observations are available. These techniques either rely on statistical correlations between the low-resolution observations and the higher-resolution reference solution, or alternatively on solving the governing equation on a high-resolution mesh with an additional forcing term that depends on the discrepancy between the observations and solution. Recently, a continuous map, called determining form, from the coarse-scale data to the high-resolution solution fields was proven to exist, subject to reasonable assumptions on the resolution of the coarse data. We propose a physics-informed deep neural network that serves as a surrogate of this theoretical map, to predict the higher-resolution solution fields from a history of coarse-scale data. The proposed network is shown to mimic the performance of dynamical downscaling, and is governed by similar constraints on the spatial and temporal resolutions of the observations.
更多查看译文
关键词
deep neural network,neural network,fluid,physics‐informed
AI 理解论文
溯源树
样例
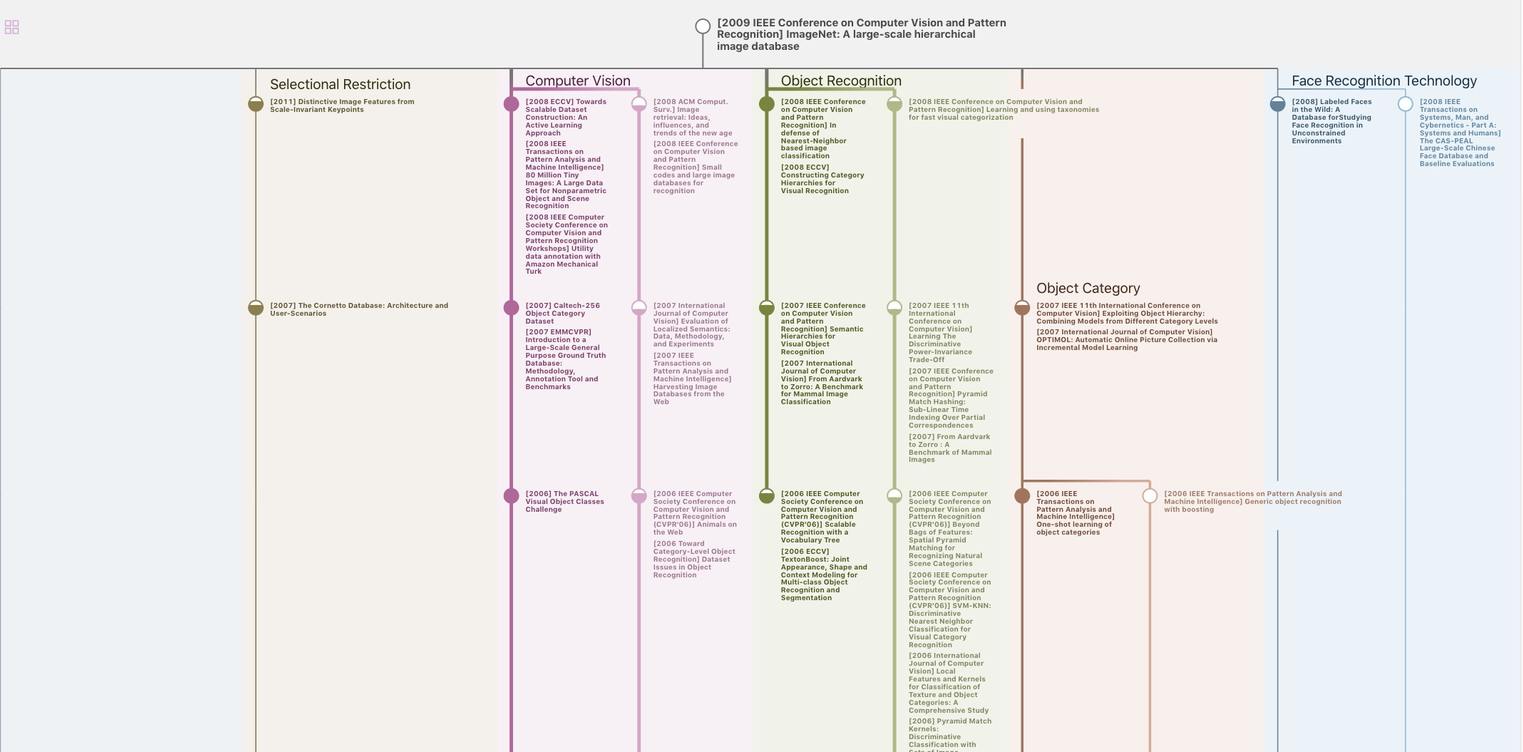
生成溯源树,研究论文发展脉络
Chat Paper
正在生成论文摘要