Learning Sparse Kernel CCA With Graph Priors for Nonlinear Process Monitoring
IEEE Sensors Journal(2023)
摘要
Process monitoring (PM) is important for improving product quality and ensuring plant safety in industrial systems. Recently, canonical correlation analysis (CCA)-based PM has shown excellent performance. The core idea is to first seek the relationship between two sets of process variables via CCA, then construct a residual generator and determine test statistics, and finally achieve the best monitoring. However, the global and local structure of process variables is not fully exploited. In this article, we propose a new nonlinear PM approach called joint sparse constrained kernel CCA and graph learning (JSKCCA-GL) by incorporating joint sparse kernel CCA (JSKCCA) and graph priors. Technically, imposing the
$\ell _{{2},{0}}$
-norm joint sparse constraints can capture the global row-wise structure, and embedding the graph Laplacian priors can preserve the local structured variable correlation information. Furthermore, an efficient alternating minimization algorithm is developed by integrating least squares and iterative hard thresholding (IHT). The superiority of the proposed JSKCCA-GL is extensively verified on the benchmark Tennessee Eastman process (TEP) and a practical cylinder-piston assembly (CPA) of marine diesel engines. In particular, the fault detection rate (FDR) values for unknown-type faults in the TEP have been improved by around 16.10% on average.
更多查看译文
关键词
Graph priors,kernel canonical correlation analysis (KCCA),process monitoring (PM),sparse optimization
AI 理解论文
溯源树
样例
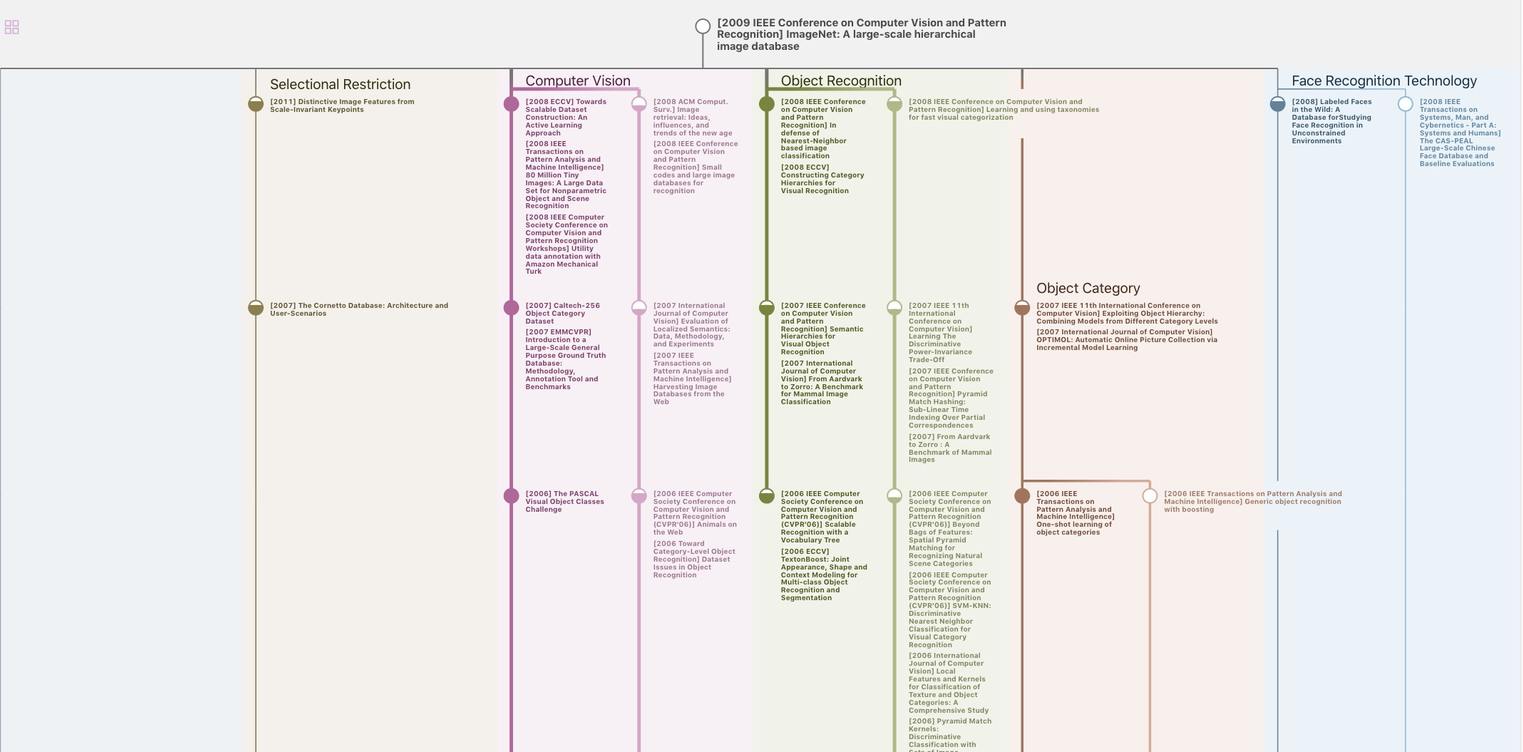
生成溯源树,研究论文发展脉络
Chat Paper
正在生成论文摘要