Generalized Roughness Bearing Fault Diagnosis Using Time Series Analysis and Gradient Boosted Tree
2022 IEEE Energy Conversion Congress and Exposition (ECCE)(2022)
摘要
Generalized roughness bearing faults are the most commonly reported ones in rotating machine failures. Early detection of bearing faults plays a vital role in minimizing operation losses. This paper aims to develop a real-time condition monitoring for bearing faults and for this purpose a sensory system to measure 3 axes vibration is developed. A time series model is proposed for vibration signals to extract features accurately. These features are classified using gradient boosted trees (GBT). GBT is an ensemble of trees and is optimal for parallel evaluation with relatively low cost. The proposed feature extraction algorithm also has a low computational cost. Overall, the proposed algorithm has very low latency and computational cost which enables fault diagnosis through embedded processors. This algorithm can diagnose five different faults with a promising accuracy of 98.5%, which is calculated for different load and speed conditions.
更多查看译文
关键词
bearing fault detection,generalized roughness fault,Gradient Boosted Tree
AI 理解论文
溯源树
样例
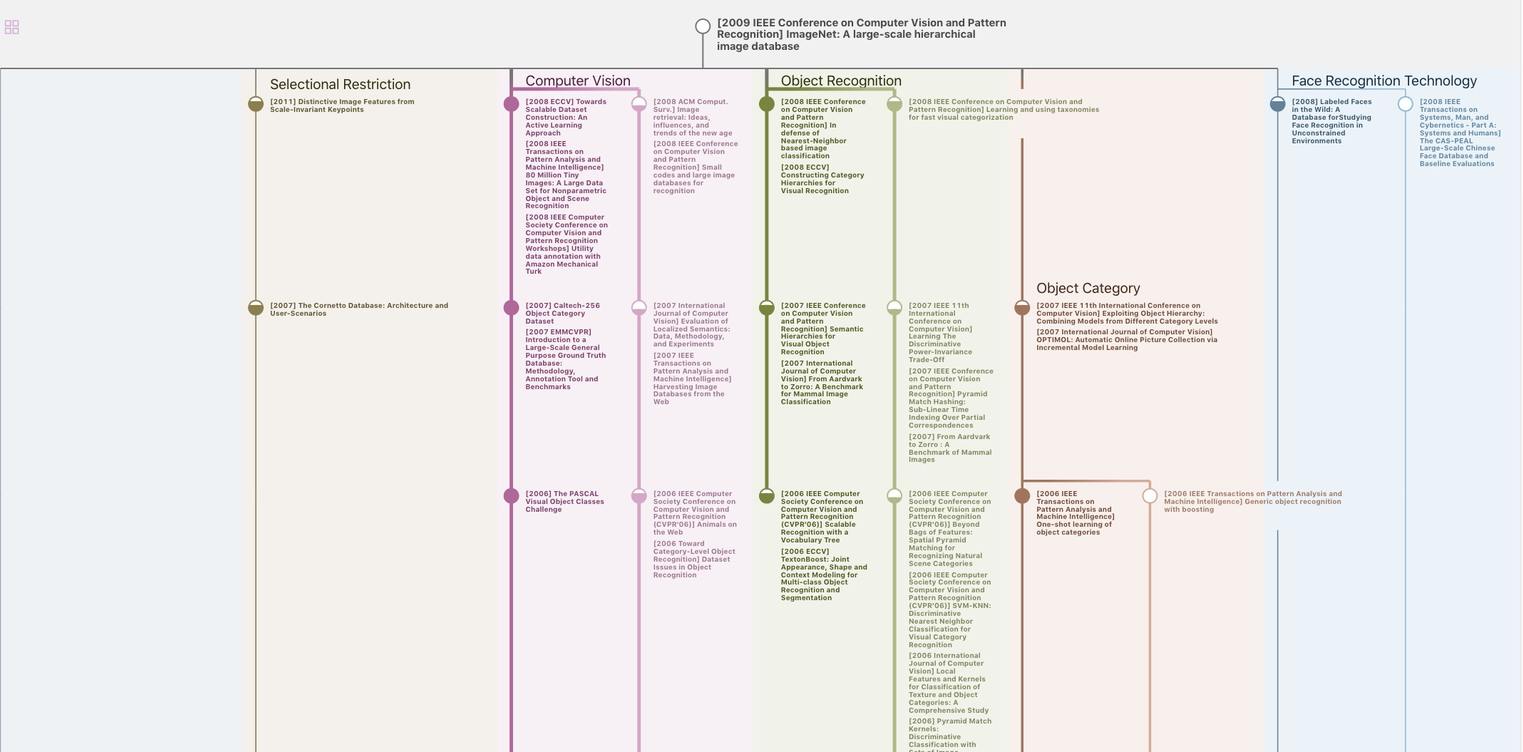
生成溯源树,研究论文发展脉络
Chat Paper
正在生成论文摘要