Neural Networks with the Correlative Layer for Multi-label Classification
Journal of Physics: Conference Series(2023)
摘要
Abstract Multi-label classification is a very significant but challenging task. Correlation between labels often exists, so recent works have paid much attention to using the correlation between labels to improve the classification performance. However, how to effectively learn the correlation is still a problem. In this paper, a general framework, i.e., the neural network with the correlative layer (CLNN), is proposed, where the correlative layer is used to express the dependencies between labels. Different from existing work, CLNN first trains a neural network without the correlative layer to obtain rough classification results and then trains the whole neural network to adaptively adjust all the weights including those of the correlation layer. Thus CLNN could learn both positive/negative and strong/weak relationships between labels. We test CLNN with three typical neural networks, and experimental results show that neural network can achieve better performance by adding the correlative layer, which demonstrates that the CLNN framework is very effective.
更多查看译文
关键词
correlative layer,classification,neural networks,multi-label
AI 理解论文
溯源树
样例
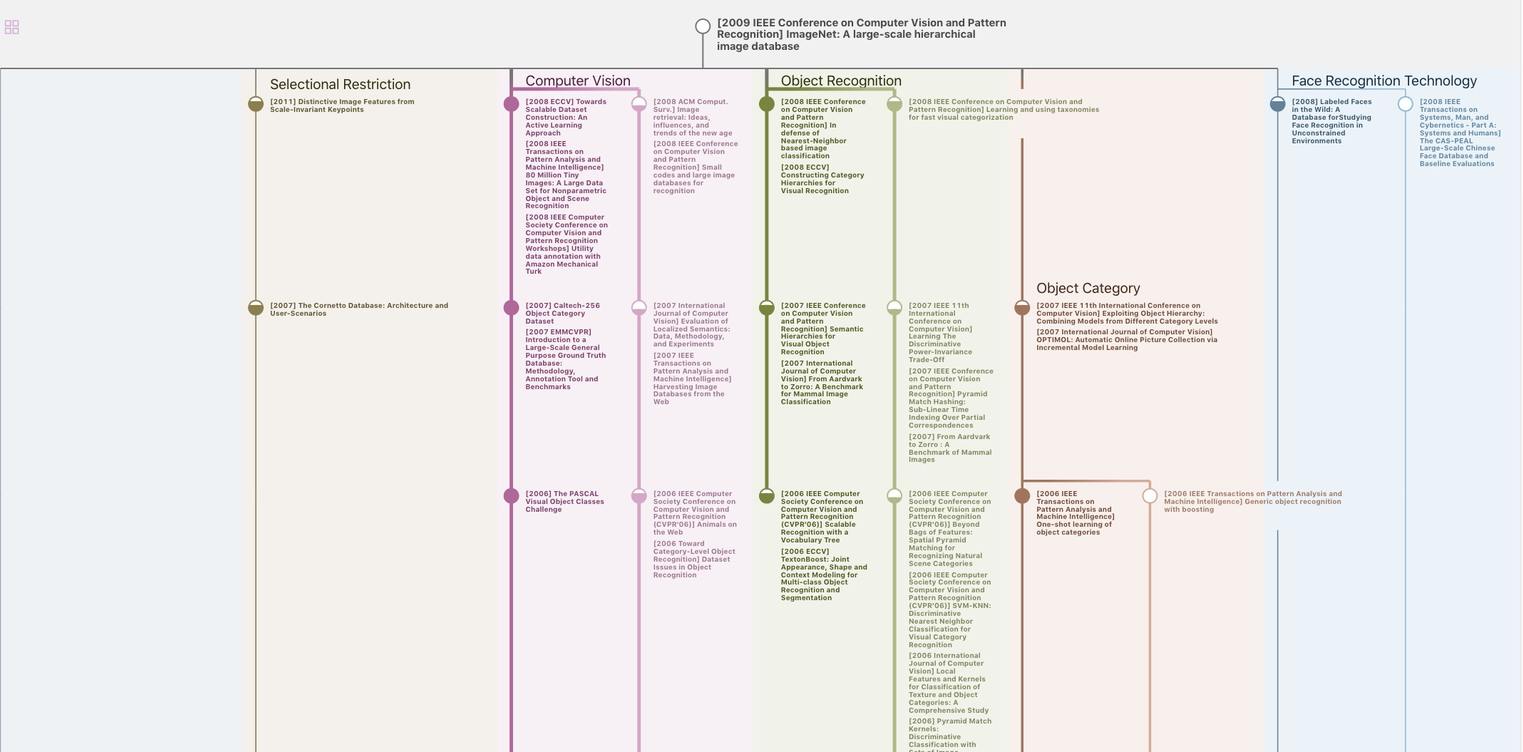
生成溯源树,研究论文发展脉络
Chat Paper
正在生成论文摘要