A Trend of AI Conference Convergence in Similarity: An Empirical Study Through Trans-Temporal Heterogeneous Graph
IEEE Transactions on Knowledge and Data Engineering(2023)
摘要
Publishing the research works on academic publications is an important part of the scientific process. Since the development of computer science research is very fast, researchers tend to publish the research works in a fast way, such as conferences whose review processes are faster than the journals. In the past decades, one conference usually focuses on a specific research field and the topic or method overlap between conferences is low. We have noticed that, in recent years, some topics or methods which were once studied in a small number of specific research fields have become popular in many other fields. Naturally, we come up with two research questions: (1) Do the conferences indeed become similar? and (2) How do conferences become similar? In this paper, we first use a trans-temporal heterogeneous graph network to model academic conferences in recent 20 years. Due to the large number of conferences, we categorize these conferences into 6 research fields for brevity. Then, we first quantitatively and qualitatively assess “Do the research fields become similar?” and then focus on exploring “How do research fields become similar?”. From the result, we find the reason for the research fields in computer science become similar is that AI becomes pervasive and researchers tend to apply the machine learning methods to different application fields. Since the methods become universal between different research fields, researchers should pay more attention to advanced information in other fields to motivate more interdisciplinary works. To assist the researchers to explore related interdisciplinary advanced information, it is crucial to measure the cross-field impact of papers using the citation information and recommend the paper which has a high cross-field impact on the related researchers. As for the newly published papers which do not have any citations, we also propose a cross-field impact prediction model to recommend the cutting-edge research works to related researchers accurately. Experiments conducted on real-world datasets verify the effectiveness of the proposed method.
更多查看译文
关键词
Academic conference,heterogeneous graph,trans-temporal
AI 理解论文
溯源树
样例
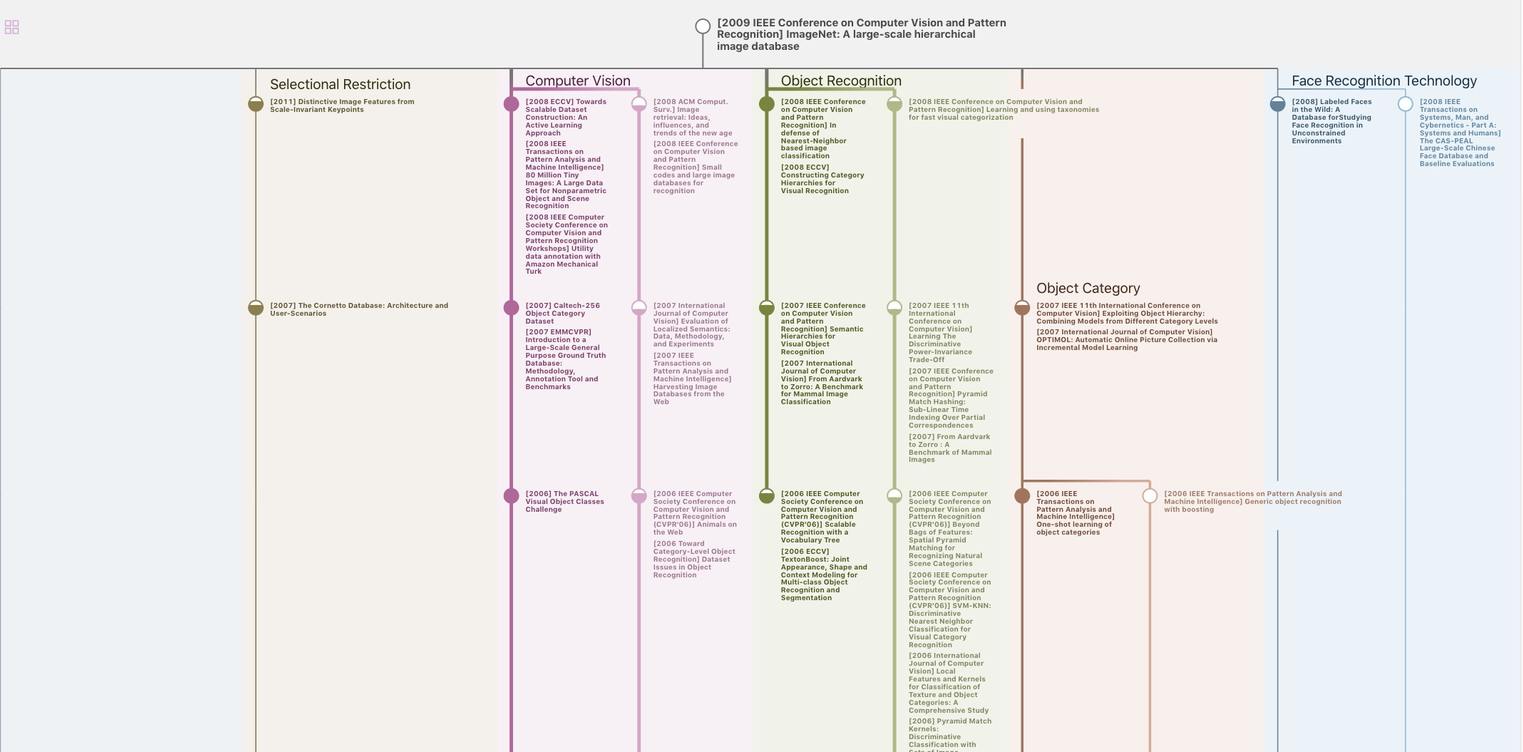
生成溯源树,研究论文发展脉络
Chat Paper
正在生成论文摘要