iGROWL: Improved Group Detection With Link Prediction
IEEE Transactions on Biometrics, Behavior, and Identity Science(2023)
摘要
One of the main challenges robots need to overcome is crowd analysis. Crowd analysis deals with the detection of individuals and interaction groups as well as the recognition of their activities. This paper focuses on the detection of conversational groups, where there have been a number of approaches addressing this problem in both supervised and unsupervised ways. Supervised bottom-up approaches primarily relied on pairwise affinity matrices and were limited to static, third-person views. In this work, we present our approach based on Graph Neural Networks (GNNs) to the problem of interaction group detection, called improved Group Detection With Link Prediction (iGROWL). iGROWL utilises the fact that interaction groups exist in certain inherent spatial configurations and improves its predecessor, GROWL, by introducing an ensemble learning-based sample balancing technique to the algorithm. Our results show that iGROWL outperforms other state-of-the-art methods by 16.7% and 26.4% in terms of
$F_{1}$
-score when evaluated on the Salsa Poster Session and Cocktail Party datasets, respectively. Moreover, we show that sample balancing with GNNs is not trivial, but consistent results can be achieved by employing ensemble learning.
更多查看译文
关键词
Biometrics council,IEEE,IEEEtran,journal,face recognition,gesture recognition,graph neural networks
AI 理解论文
溯源树
样例
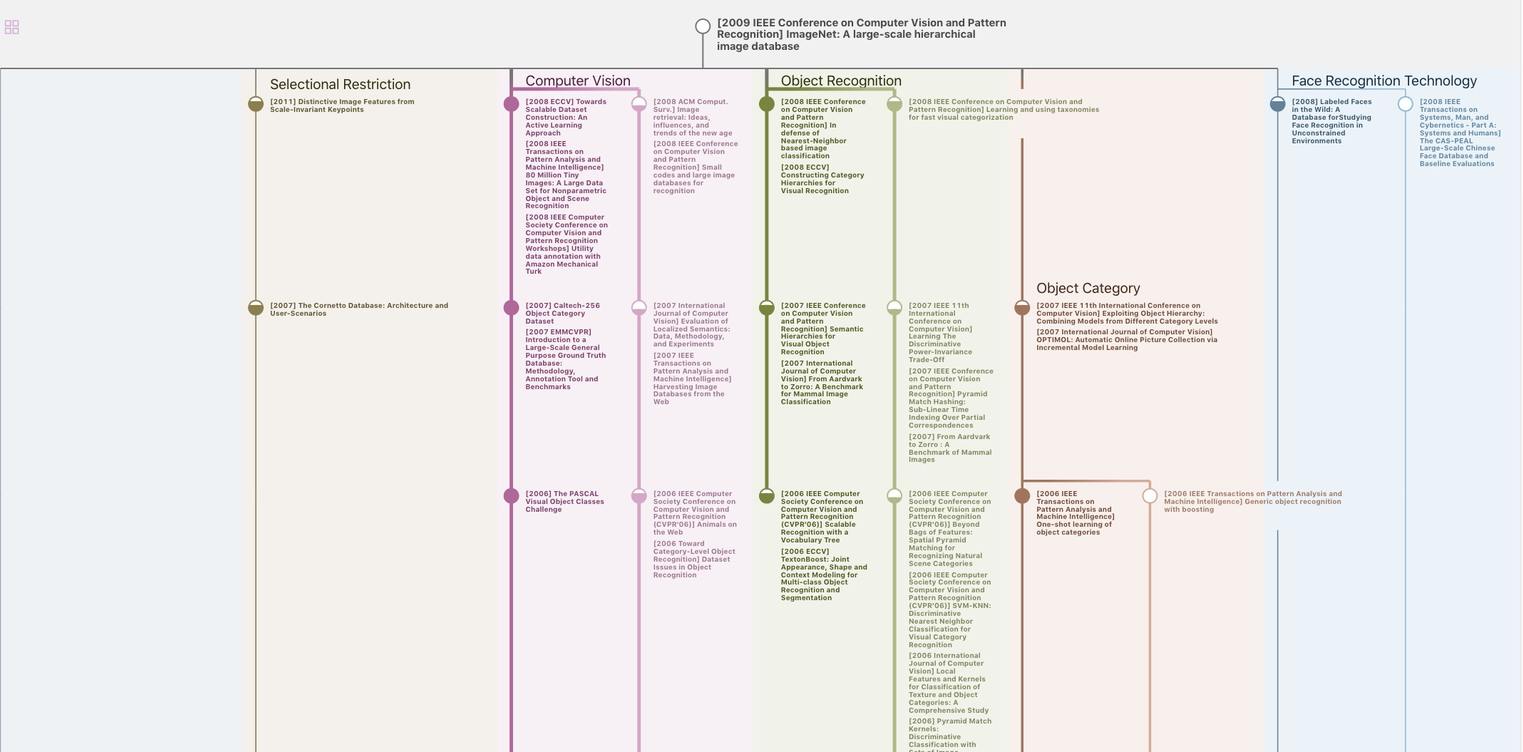
生成溯源树,研究论文发展脉络
Chat Paper
正在生成论文摘要