A lightweight deep learning approach to mouth segmentation in color images
Applied Computing and Informatics(2022)
摘要
PurposeMouth segmentation is one of the challenging tasks of development in lip reading applications due to illumination, low chromatic contrast and complex mouth appearance. Recently, deep learning methods effectively solved mouth segmentation problems with state-of-the-art performances. This study presents a modified Mobile DeepLabV3 based technique with a comprehensive evaluation based on mouth datasets.Design/methodology/approachThis paper presents a novel approach to mouth segmentation by Mobile DeepLabV3 technique with integrating decode and auxiliary heads. Extensive data augmentation, online hard example mining (OHEM) and transfer learning have been applied. CelebAMask-HQ and the mouth dataset from 15 healthy subjects in the department of rehabilitation medicine, Ramathibodi hospital, are used in validation for mouth segmentation performance.FindingsExtensive data augmentation, OHEM and transfer learning had been performed in this study. This technique achieved better performance on CelebAMask-HQ than existing segmentation techniques with a mean Jaccard similarity coefficient (JSC), mean classification accuracy and mean Dice similarity coefficient (DSC) of 0.8640, 93.34% and 0.9267, respectively. This technique also achieved better performance on the mouth dataset with a mean JSC, mean classification accuracy and mean DSC of 0.8834, 94.87% and 0.9367, respectively. The proposed technique achieved inference time usage per image of 48.12 ms.Originality/valueThe modified Mobile DeepLabV3 technique was developed with extensive data augmentation, OHEM and transfer learning. This technique gained better mouth segmentation performance than existing techniques. This makes it suitable for implementation in further lip-reading applications.
更多查看译文
关键词
mouth segmentation,lightweight deep learning approach,deep learning
AI 理解论文
溯源树
样例
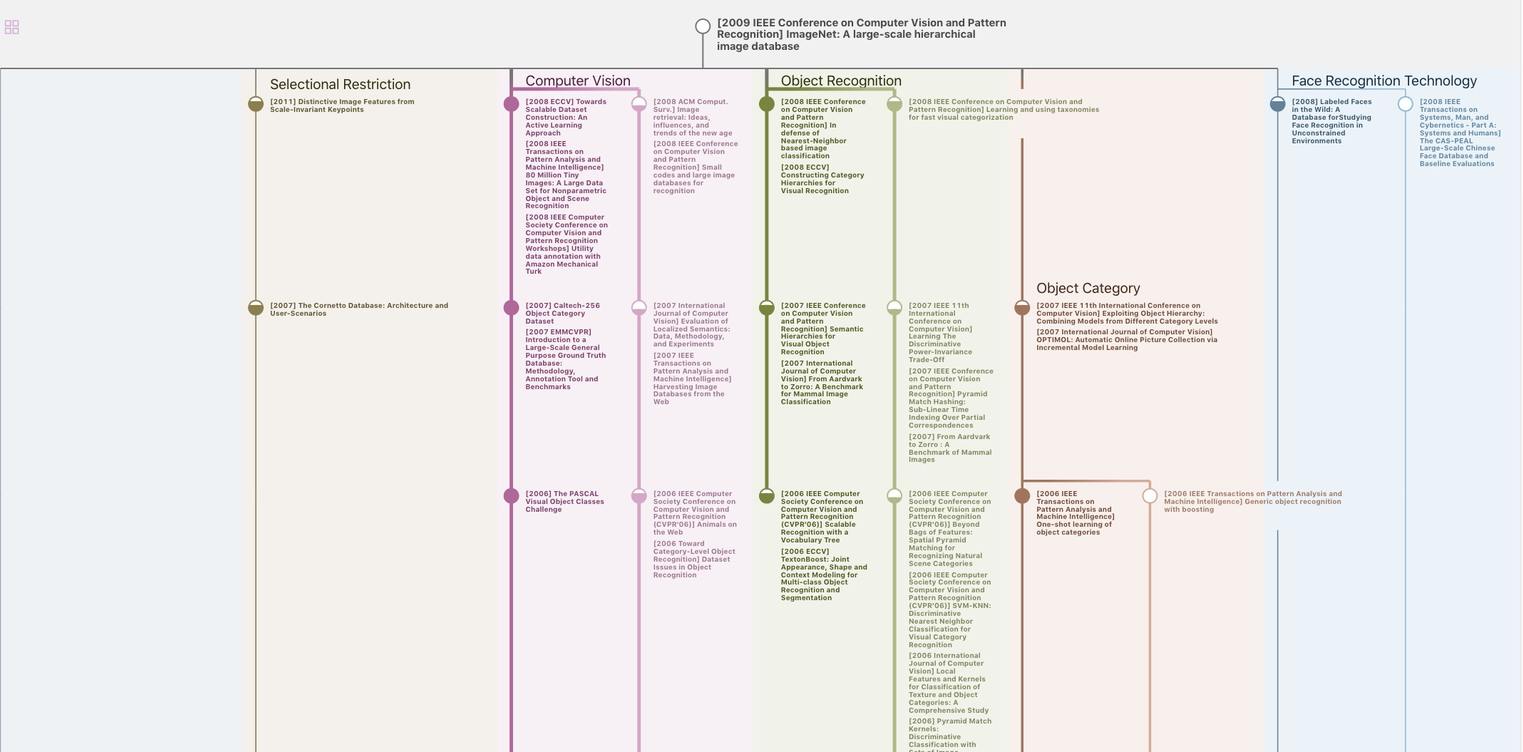
生成溯源树,研究论文发展脉络
Chat Paper
正在生成论文摘要