Towards Unconstrained Vocabulary Eavesdropping With mmWave Radar Using GAN
IEEE TRANSACTIONS ON MOBILE COMPUTING(2024)
摘要
As acoustic communication systems become increasingly common in our daily life, eavesdropping brings severe security and privacy risks. Current methods of acoustic eavesdropping either provide low resolution due to the use of sub-6 GHz frequencies, work only for limited words based on classification approaches, or cannot work through-wall because of the use of optical sensors. In this article, we present milliEar, a mmWave acoustic eavesdropping system that leverages the high-resolution of mmWave FMCW ranging and generative machine learning models to not only extract vibrations but to reconstruct the audio. milliEar combines speaker vibration estimation with conditional generative adversarial networks to eavesdrop and recover high-quality audios (i.e., with no vocabulary constraints). We implement and evaluate milliEar using off-the-shelf mmWave radars deployed in different scenarios and settings. Evaluation results clearly show that milliEar can accurately reconstruct the audio even at different distances, angles, and through the wall with different insulator materials. In addition, our subjective and objective evaluations demonstrate that the reconstructed audio has a strong similarity with the original audio.
更多查看译文
关键词
Acoustic eavesdropping,mmWave radar,vibration sensing,generative adversarial network
AI 理解论文
溯源树
样例
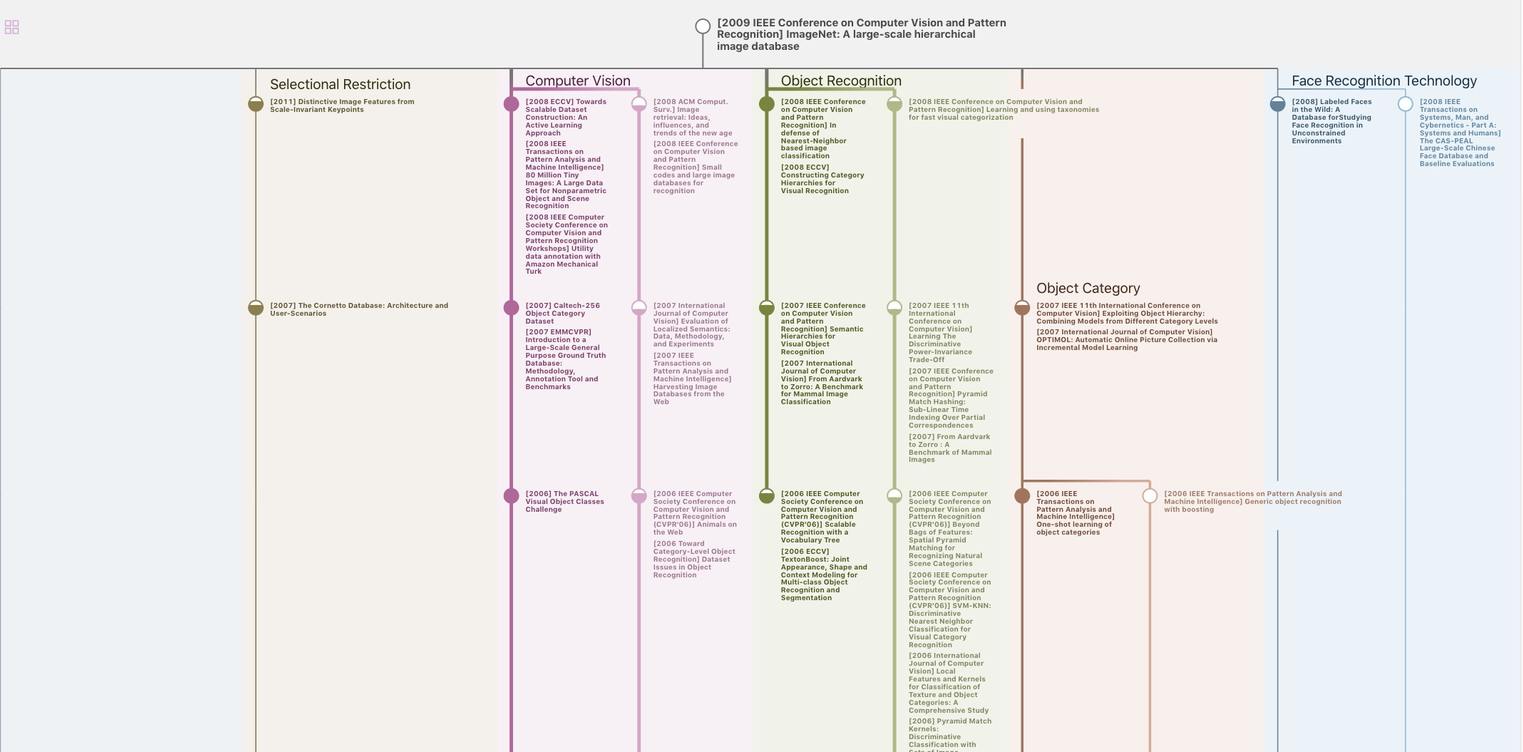
生成溯源树,研究论文发展脉络
Chat Paper
正在生成论文摘要