Focusing Fine-Grained Action by Self-Attention-Enhanced Graph Neural Networks With Contrastive Learning
IEEE Transactions on Circuits and Systems for Video Technology(2023)
摘要
With the aid of graph convolution neural network and transformer model, human action recognition has achieved significant performance based on skeleton data. However, the majority of existing works rarely focus on identifying fine-grained motion information (i.e., “read”, “write”, etc.). Furthermore, they tend to explore correlations between joints and bones ignoring the angular information. Consequently, the recognition accuracy for fine-grained actions with most models is still less desired. To address this issue, we first attempt to bring angular information as a complement to familiar joint and bone information, while learning the potential dependencies of the three kinds of information using graph neural networks. Based on this, we propose a self-attention-enhanced graph neural network (SAE-GNN), which consists of a kernel-unified graph convolution (KUGC) module and an enhanced attention graph convolution (EAGC) module. The KUGC module is devised to effectively extract rich features in the skeleton information. The EAGC consisting of a multi-scale enhanced graph convolution block and a multi-headed self-attention block is designed to learn the potential high-level semantic information in the features. Besides, we introduce contrastive learning in the two blocks to enhance feature representation by maximizing their mutual information. We conduct extensive experiments on four publicly available datasets, and results show that our model outperforms state-of-the-art methods in recognizing fine-grained actions.
更多查看译文
关键词
graph neural networks,contrastive learning,action,fine-grained,self-attention-enhanced
AI 理解论文
溯源树
样例
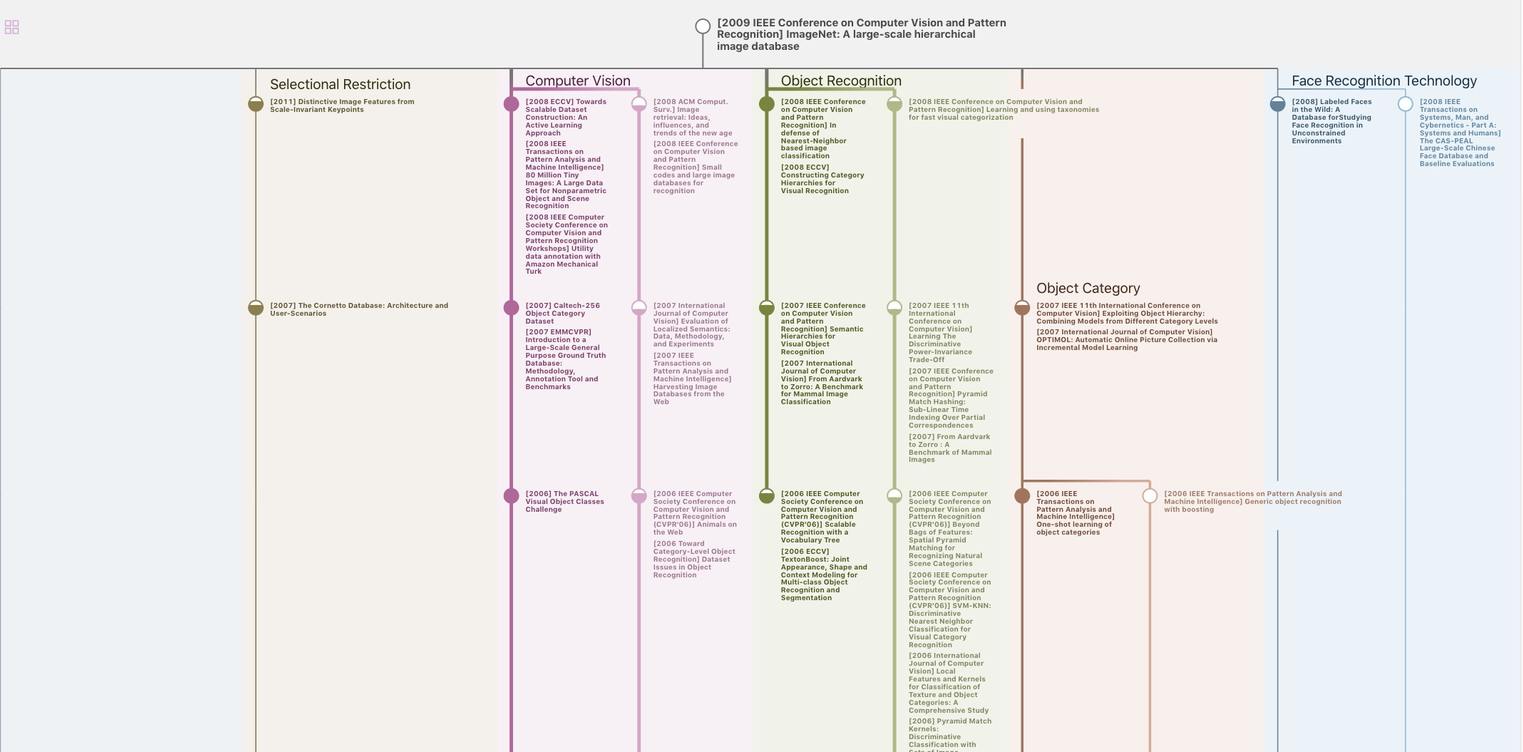
生成溯源树,研究论文发展脉络
Chat Paper
正在生成论文摘要