Biomarkers Identification of Hepatocellular Carcinoma Based on Multi-omics Data Integration and Graph-embedded Deep Neural Network
Current Bioinformatics(2023)
摘要
Abstract: Hepatocellular carcinoma (HCC) is one of the malignancies with a high mortality rate, and identifying relevant biomarkers of HCC is helpful for early diagnosis and patient care. Though some high-dimensional omic data contains intrinsic biomedical information about HCC, how to integrate analysis of them effectively and find promising biomarkers of HCC is still an important and difficult issue. Here, we present a novel biomarker identification approach, named GEDNN, based on multi-omic data and graph-embedded deep neural networks. To achieve a more comprehensive understanding of HCC, we first collected and normalized the three following types of HCC-related data: DNA methylation, copy number variation (CNV), and gene expression. The ANOVA was adopted to filter out redundant genes. Then, we measured the connectivity between gene pairs by Pearson correlation coefficient of gene pairs, and further construct gene graph. Next, graph-embedded feedforward neural network (DFN) and back-propagation of convolutional neural network (CNN) were combined to integrative analyze the three types of omics data and achieve the importance score of gene biomarkers. Extensive experimental results showed that the biomarkers screened by the proposed method were effective in classifying and predicting HCC. Furthermore, the gene analysis further showed that the biomarkers screened by our method were strongly associated with the development of HCC. background: Hepatocellular carcinoma (HCC) is one of the malignancies with high mortality rate, and identify relevant biomarkers of HCC is helpful for early diagnosis and patient care. Though some high-dimensional omic data contains intrinsic biomedical information about HCC, how to integrate analysis them effectively and find promising biomarkers of HCC is still an important and difficult issue. Background: Hepatocellular carcinoma (HCC) is one of the malignancies with high mortality rate, and identify relevant biomarkers of HCC is helpful for early diagnosis and patient care. Though some high-dimensional omic data contains intrinsic biomedical information about HCC, how to integrate analysis them effectively and find promising biomarkers of HCC is still an important and difficult issue. Method: We present a novel biomarker identification approach, named GEDNN, based on multi-omic data and graph-embedded deep neural network. To achieve a more comprehensive understanding of HCC, we first collected and normalized the three following types of HCC-related data: DNA methylation, copy number variation (CNV), and gene expression. The ANOVA was adopted to filter out redundant genes. Then, we measured the connectivity between gene pairs by Pearson correlation coefficient of gene pairs, and further construct gene graph. Next, graph-embedded feedforward neural network (DFN) and back-propagation of convolutional neural network (CNN) were combined to integratively analyze the three types of omics data and achieve the importance score of gene biomarkers. Result: Extensive experimental results showed that the biomarkers screened by the proposed method were effective in classifying and predicting HCC. Furthermore, the gene analysis further showed that the biomarkers screened by our method were strongly associated with the development of HCC. Conclusion: In this paper, we propose the GEDNN method to assess the importance of genes for more accurate identification of cancer biomarkers, which facilitates the effective classification of cancers. The proposed method is applied to multi-omics data of HCC, including RNASeq, DNAMeth and CNV, considering the complementary information between different types of data. We construct a gene graph by Pearson correlation coefficients as additional information for DFN, thus reducing the importance score of redundant genes. In addition, the proposed method also incorporates back-propagation of CNN to further obtain the importance of features.
更多查看译文
关键词
multiomics data integration,hepatocellular carcinoma,biomarkers,deep neural network,graph-embedded
AI 理解论文
溯源树
样例
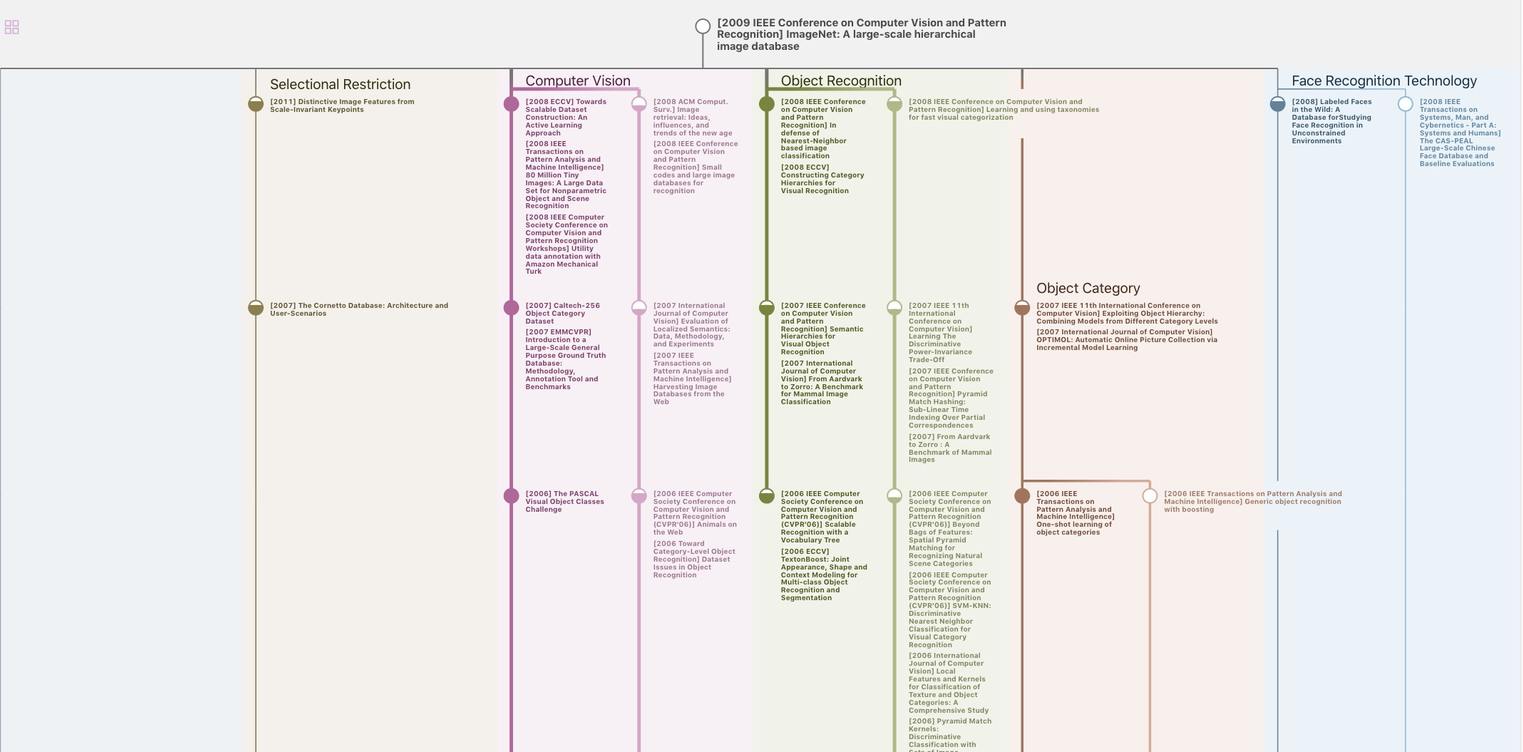
生成溯源树,研究论文发展脉络
Chat Paper
正在生成论文摘要