An EOSC-enabled Data Space environment for the climate community
crossref(2023)
摘要
<p>The exponential increase in data volumes and complexities is causing a radical change in the scientific discovery process in several domains, including climate science. This affects the different stages of the data lifecycle, thus posing significant data management challenges in terms of data archiving, access, analysis, visualization, and sharing. The data space concept can support scientists' workflow and simplify the process towards a more FAIR use of data.</p> <p>In the context of the European Open Science Cloud (EOSC) initiative launched by the European Commission, the ENES Data Space (EDS) represents a domain-specific implementation of the data space concept. The service, developed in the frame of the EGI-ACE project, aims to provide an open, scalable, cloud-enabled data science environment for climate data analysis on top of the EOSC Compute Platform. It is accessible in the European Open Science Cloud (EOSC) through the EOSC Catalogue and Marketplace (https://marketplace.eosc-portal.eu/services/enes-data-space) and it also provides a web portal (https://enesdataspace.vm.fedcloud.eu) including information, tutorials and training materials on how to get started with its main features. </p> <p>The EDS integrates into a single environment ready-to-use climate datasets, compute resources and tools, all made available through the Jupyter interface, with the aim of supporting the overall scientific data processing workflow.  Specifically, the data store linked to the ENES Data Space provides access to a multi-terabyte set of variable-centric collections from large-scale global climate experiments.  The data pool consists of a mirrored subset of CMIP (Coupled Model Intercomparison Project) datasets from the ESGF (Earth System Grid Federation) federated data archive, collected and kept synchronized with the remote copies by using the Synda tool developed within the scope of the IS-ENES3 H2020 project. Community-based, open source frameworks (e.g., Ophidia) and libraries from the Python ecosystem provide the capabilities for data access, analysis and visualisation. Results  and experiment definitions (i.e., Jupyter Notebooks) can be easily shared among users promoting data sharing and application re-use towards a more Open Science approach. </p> <p>An overview of the data space capabilities along with the key aspects in terms of data management will be presented in this work.</p>
更多查看译文
AI 理解论文
溯源树
样例
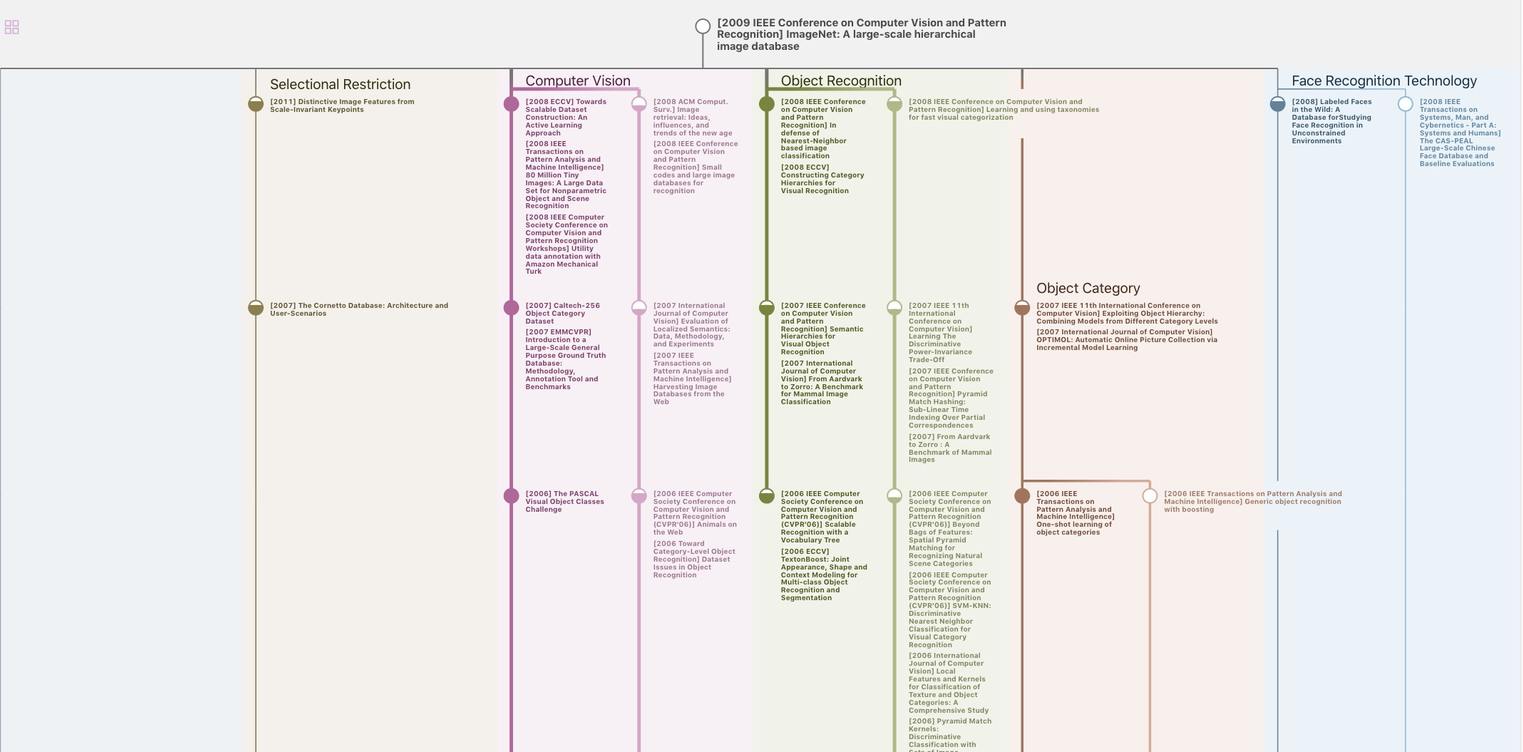
生成溯源树,研究论文发展脉络
Chat Paper
正在生成论文摘要