Simulation of Arctic sea ice within the Eocene Deep-Time Model Intercomparison Project: thresholds, seasonality and factors controlling sea ice development
crossref(2023)
摘要
<p>The early Eocene greenhouse climate driven by high atmospheric CO<sub>2</sub> concentrations serves as a testbed for future climate changes dominated by increasing CO<sub>2</sub> forcing. Especially, the early Eocene Arctic region is important in light of future CO<sub>2</sub>-driven climate warming in the northern polar region. Here, we present early Eocene Arctic sea ice simulations carried out by coupled climate models within the framework of the Deep-Time Model Intercomparison Project. We find differences in sea ice responses to CO<sub>2</sub> changes across the ensemble and compare the results with existing proxy-based sea ice reconstructions from the Arctic Ocean. Most of the models simulate winter sea ice presence at high CO<sub>2</sub> levels (≥ 840 ppmv = 3x pre-industrial (PI) level of 280 ppmv). However, the threshold when sea ice permanently disappears from the ocean varies significantly between the models (from < 840 ppmv to > 1680 ppmv). Based on a one-dimensional energy balance model analysis we find that the greenhouse effect plays an important role in the inter-model spread in Arctic winter surface temperature changes in response to a CO<sub>2</sub> rise from 1x to 3x the PI level. We link this greenhouse effect to increased atmospheric water vapour concentration. Furthermore, differences in simulated surface salinity in the Arctic Ocean play an important role in controlling local sea ice formation. These inter-model differences result from differences in the exchange of waters between a brackish Arctic and a more saline North Atlantic Ocean that are controlled by the width of the gateway between both basins. As there is no geological evidence for Arctic sea ice in the early Eocene, its presence in most of the simulations with 3x PI CO<sub>2</sub> level indicates either a higher CO<sub>2</sub> level and/or models miss important mechanism/feedback.</p>
更多查看译文
AI 理解论文
溯源树
样例
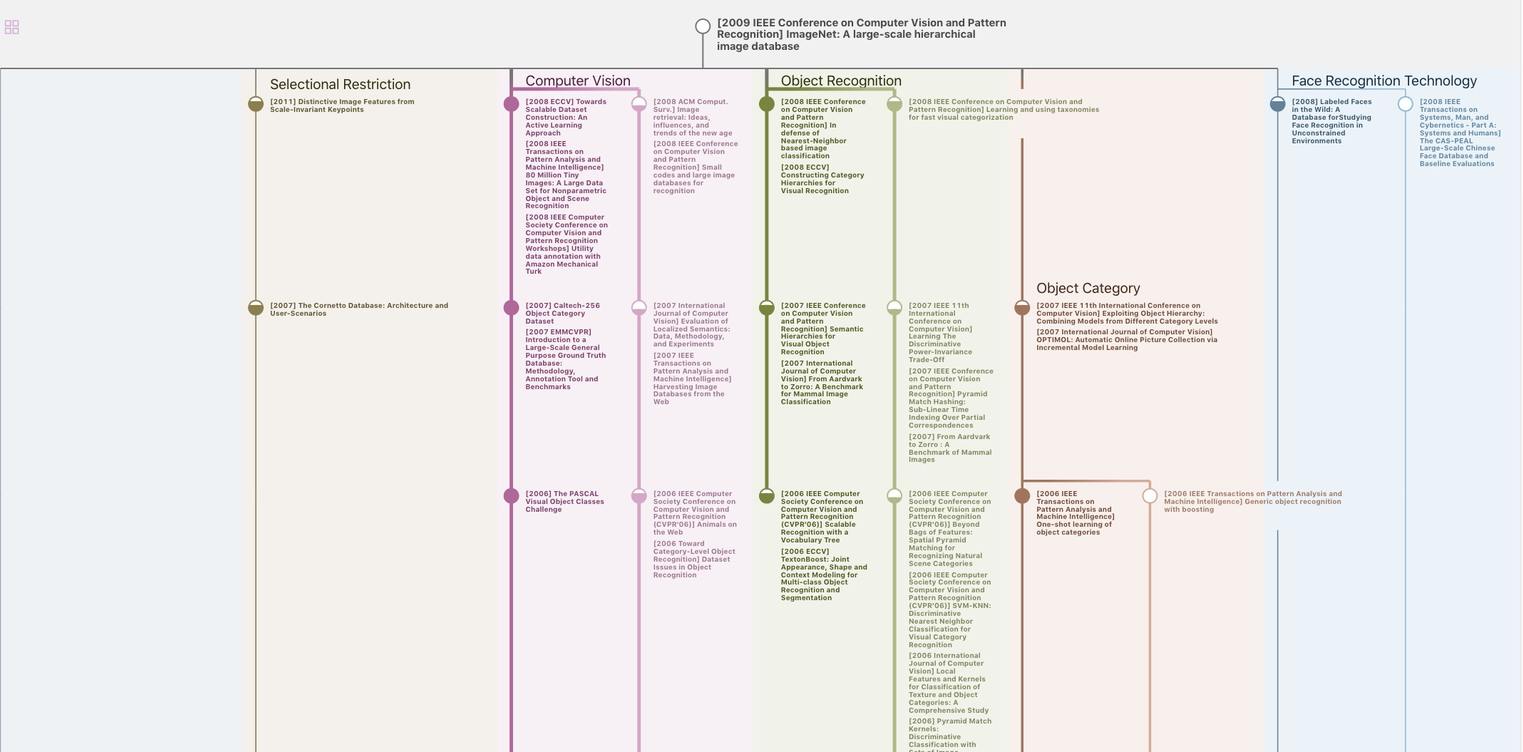
生成溯源树,研究论文发展脉络
Chat Paper
正在生成论文摘要