Shared communication for coordinated large-scale reinforcement learning control
SICE Journal of Control, Measurement, and System Integration(2023)
摘要
Deep Reinforcement Learning (DRL) recently emerged as a possibility to control complex systems without the need to model them mathematically. In contrast to classical controllers, DRL alleviates the need for constant parameter tuning, tedious design of control laws, and re-identification procedures in the event of performance degradation. However, the application of DRL algorithms remains fairly modest, and they have not yet established a significant position in process industries. One major obstacle has been their sample inefficiency when facing tasks featuring large state-action spaces. In this work, we show that it is possible to use DRL for plant-wide control by decentralizing and coordinating reinforcement learning. Namely, we express the global policy as a collection of local policies. Every local policy receives local observations and is responsible for controlling a different region of the environment. To enable coordination among local policies, we present a mechanism based on message passing. Messages are encoded by a shared communication channel, which is equipped with a model-based stream to capture the dynamics of the system and enable effective pre-training. The proposed method is evaluated on a set of robotic tasks and a large-scale vinyl acetate monomer (VAM) plant. Experimental results highlight that the proposed model exhibits drastic improvements over baselines in terms of mean scores and sample efficiency.
更多查看译文
关键词
shared communication,reinforcement,large-scale large-scale,control
AI 理解论文
溯源树
样例
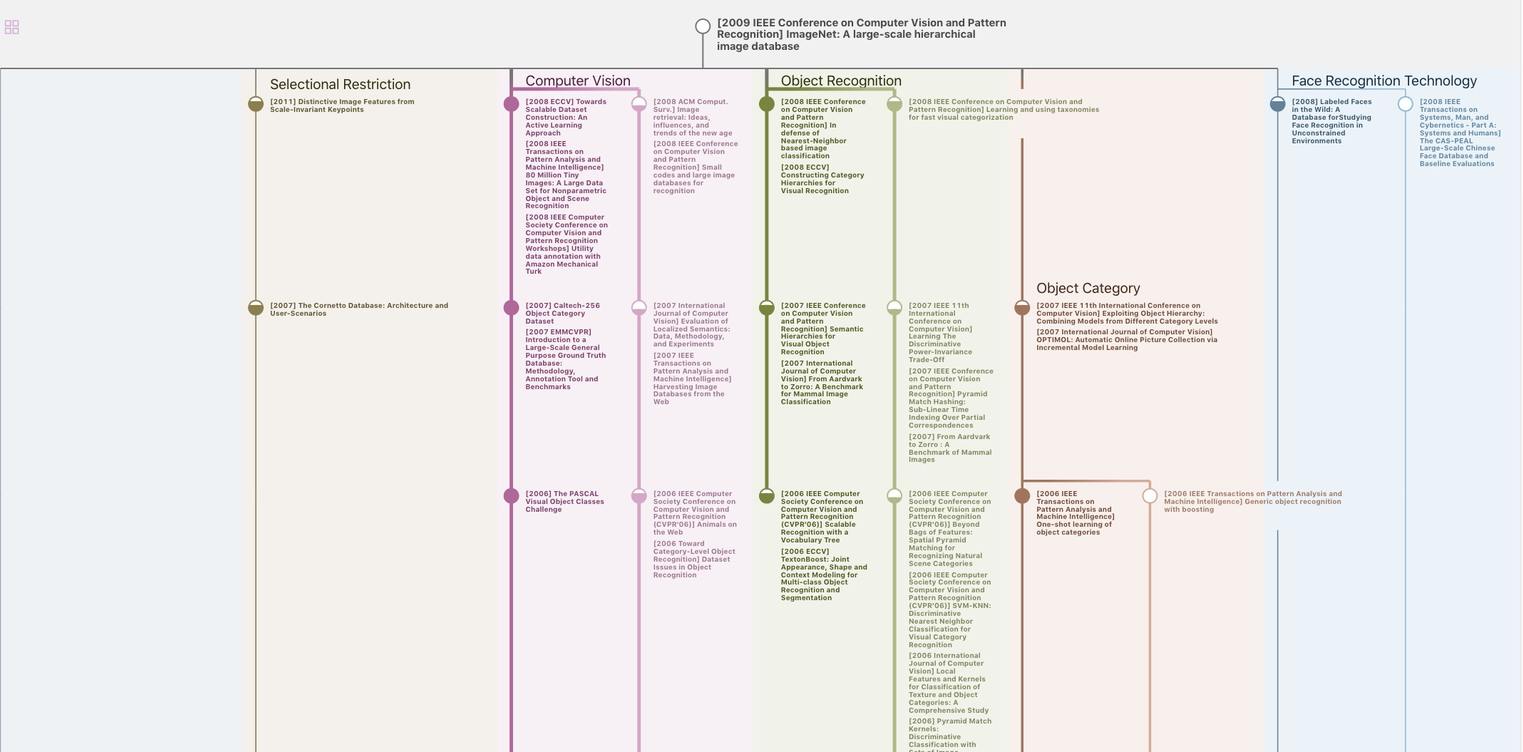
生成溯源树,研究论文发展脉络
Chat Paper
正在生成论文摘要