A deep-learning model of prescient ideas demonstrates that they emerge from the periphery
PNAS NEXUS(2023)
摘要
Where do prescient ideas-those that initially challenge conventional assumptions but later achieve widespread acceptance-come from? Although their outcomes in the form of technical innovation are readily observed, the underlying ideas that eventually change the world are often obscured. Here, we develop a novel method that uses deep learning to unearth the markers of prescient ideas from the language used by individuals and groups. Our language-based measure identifies prescient actors and documents that prevailing methods would fail to detect. Applying our model to corpora spanning the disparate worlds of politics, law, and business, we demonstrate that it reliably detects prescient ideas in each domain. Moreover, counter to many prevailing intuitions, prescient ideas emanate from each domain's periphery rather than its core. These findings suggest that the propensity to generate far-sighted ideas may be as much a property of contexts as of individuals. Significance Statement: Prescient ideas-those that initially challenge conventional assumptions but later achieve widespread acceptance-drive themost consequential changes in society. Yet such ideas are difficult to directly observe, leading researchers to focus on how they manifest in the form of domain-specific technical innovations. Here, we develop a novel, deep-learning method to identify prescient ideas in everyday language. We demonstrate that this common approach to quantifying prescience can be applied across such disparate domains as law, politics, and business and that prescient ideas arise from the periphery rather than the center of these fields. Although the capacity to generate prescient ideas is often attributed to individuals, its origins may have as much to do with place as with people.
更多查看译文
关键词
prescient ideas,deep-learning deep-learning,periphery
AI 理解论文
溯源树
样例
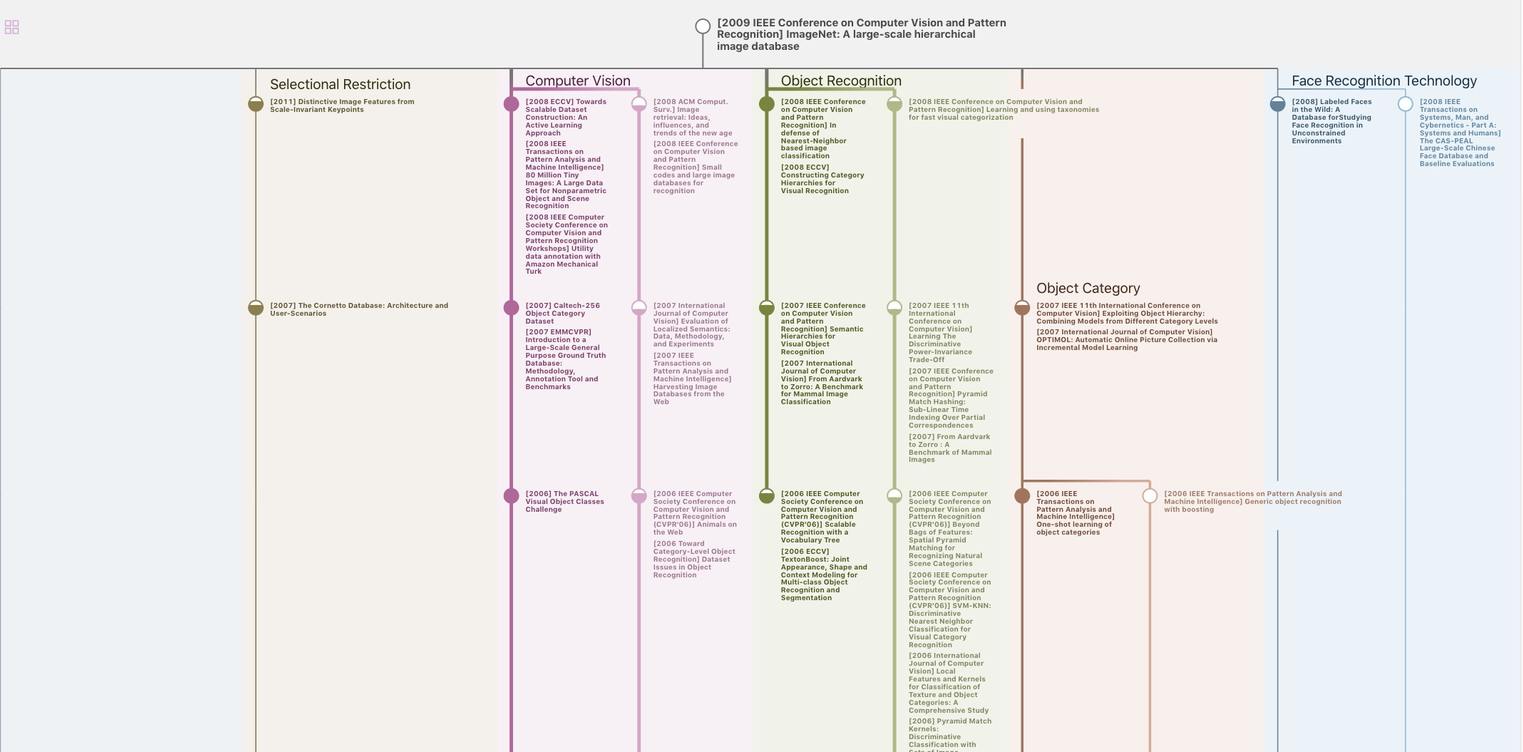
生成溯源树,研究论文发展脉络
Chat Paper
正在生成论文摘要