Marine Floating Plastic Detection using Open Source Earth Observation resources
crossref(2023)
摘要
<p>Marine plastic pollution is one of the major environmental problems that human civilization is currently experiencing. Every year more than 300 million tons of plastic are produced; surprisingly, half of them are single-use items such as shopping bags, cups and straws. Every year, around 14 million tons of plastic enter the ocean, and due to the extended life span, this plastic remains in the ocean for about 400-500 years until the degradation starts. Plastic debris is currently the most abundant type of litter in the ocean, contributing to 80% of all marine debris found in the ocean. Plastic waste in the ocean harms a wide variety of marine life, including fish, sea turtles, and marine mammals, as well as birds and other animals that feed on the ocean. In this work, an effort has been made to explore the potential application of open-source earth-observation resources and machine learning algorithms for detecting marine floating plastic (MFP). Different sites were considered for implementing the developed model to see the performance and accuracy of the classifiers. Among the chosen sites, two sites, i.e. Skala Loutron  (Greece), and Limassol (Cyprus), were considered for training the models and two sites, i.e. Calabria (Italy), and Beirut (Lebanon) were used for testing the model. In addition, two machine learning classifiers, i.e. support vector machine (SVM) and random forest (RF), were also used to classify the objects into categories and discriminate floating plastic from other objects.  In-situ ground truth data collected from the controlled experiments conducted in Mytilene, Greece (in 2018 and 2019), Skala Loutron, Greece (2021), and Limassol, Cyprus (2018) were used for training the machine learning classifiers. The trained models were then tested on unseen real-world data collected from Calabria (Italy), and Beirut (Lebanon). The train and test were carried out in five train and test case scenarios to explore the sensitivity of the models in varied datasets. Several Sentinel 2A/B spectral bands and remote sensing spectral indices were used as training inputs for constructing the models.   A newly developed index, kernel Normalized Difference Vegetation Index (kNDVI), was also used in this study to examine its contribution to model performances. Both the classifiers, i.e. SVM and RF performed well for all five models and test case combinations. Among the two ML models, the RF model was found superior in terms of the highest accuracy and precision score. The inclusion of kNDVI was crucial as the same has substantially increased the detection accuracy. Amongst the remote sensing indices used in this study, the Floating Debris Index (FDI) was the most important predictor for detecting marine floating plastic. The outcome of this study collectively suggests that open-source earth observation resources can be an effective alternative for the cost-effective detection of MFP.</p>
更多查看译文
AI 理解论文
溯源树
样例
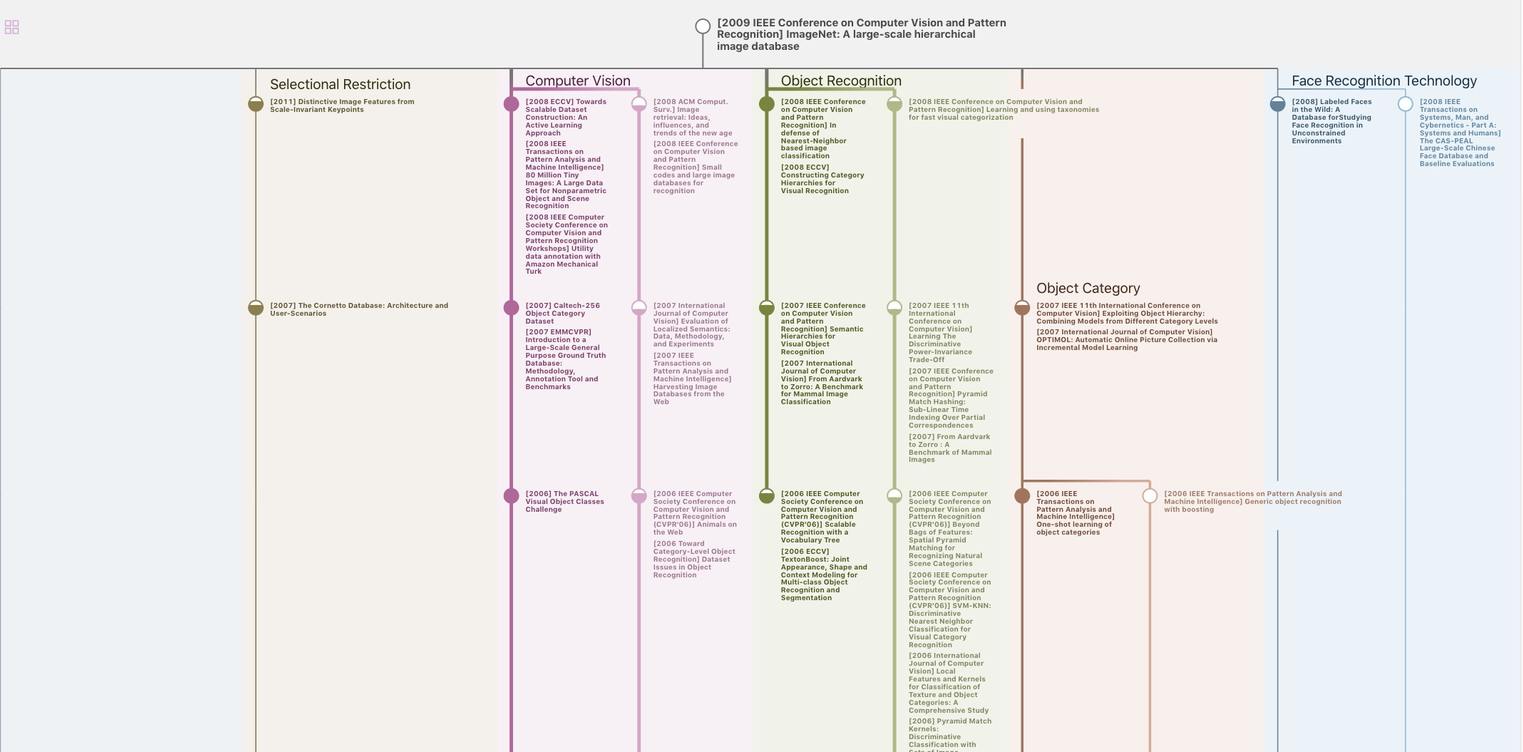
生成溯源树,研究论文发展脉络
Chat Paper
正在生成论文摘要