SQ-Swin: Siamese Quadratic Swin Transformer for Lettuce Browning Prediction
IEEE ACCESS(2023)
摘要
Enzymatic browning is a major quality defect of packaged "ready-to-eat" fresh-cut lettuce salads. While there have been many research and breeding efforts to counter this problem, progress is hindered by the lack of a technology to identify and quantify browning rapidly, objectively, and reliably. Here, we report a deep learning model for lettuce browning score prediction. To the best of our knowledge, it is the first-of-its-kind on deep learning for lettuce browning prediction using a Siamese Quadratic Swin (SQ-Swin) transformer with several highlights. First, our model includes quadratic features in the transformer model which is more powerful to incorporate real world representations than the linear transformer. Second, a multi-scale training strategy is employed to augment the data and explore more of the inherent self-similarity of the lettuce images. Third, the proposed model uses a siamese architecture which learns the inter-relations among the limited training samples. Fourth, the model is pretrained on the ImageNet and then trained with the reptile meta-learning algorithm to learn higher-order gradients than a regular one. Experiment results on the fresh-cut lettuce datasets show that the proposed SQ-Swin outperforms the traditional methods and other deep learning-based backbones.
更多查看译文
关键词
Lettuce,enzymatic browning,transformer,quadratic,siamese model,reptile
AI 理解论文
溯源树
样例
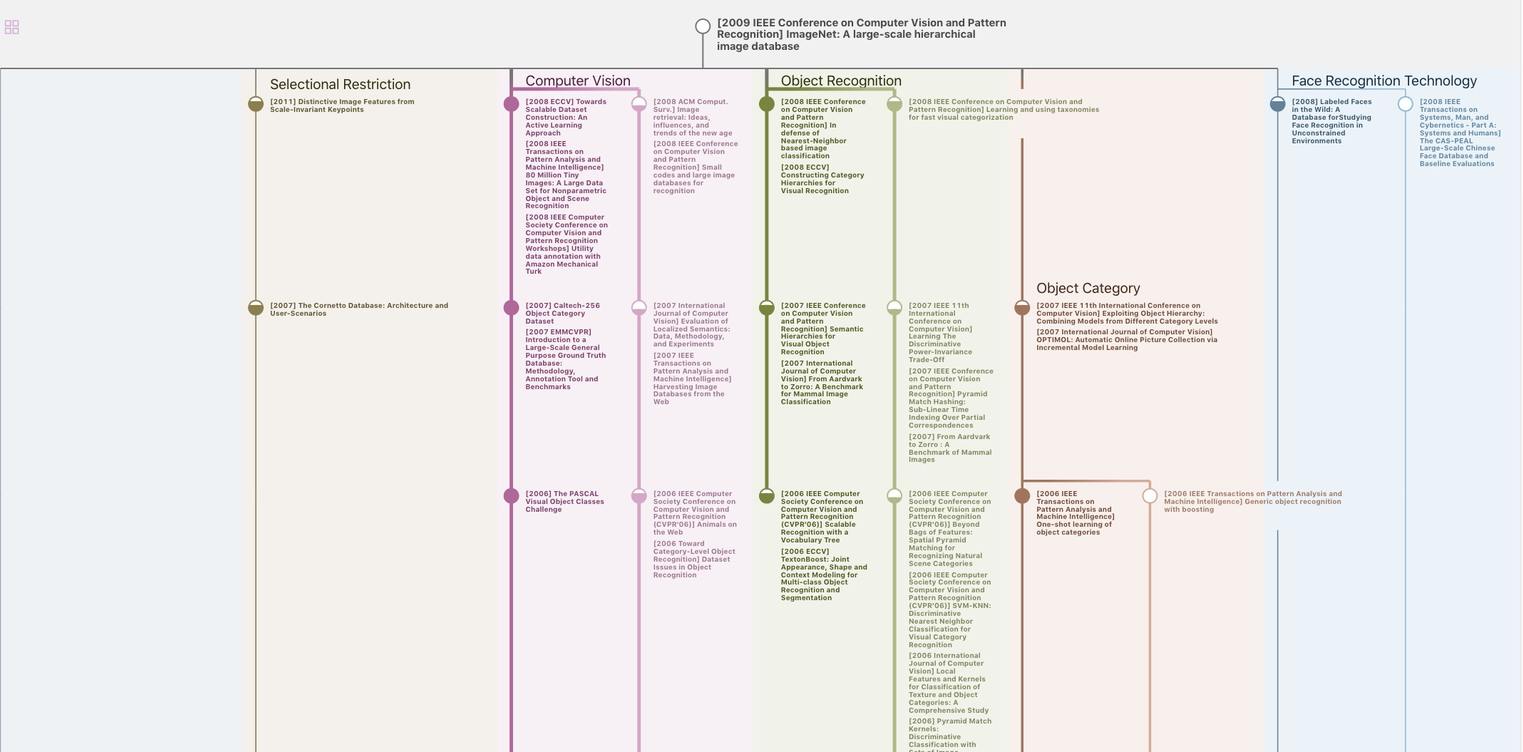
生成溯源树,研究论文发展脉络
Chat Paper
正在生成论文摘要