A New Cross-Domain Bearing Fault Diagnosis Framework Based on Transferable Features and Manifold Embedded Discriminative Distribution Adaption Under Class Imbalance
IEEE Sensors Journal(2023)
摘要
Cross-domain fault diagnosis based on transfer learning has been popularly developed to overcome inconsistent data distribution-caused degradation of diagnostic performance. However, the existing methods are typically suffering from a class imbalance of domains and lacking sufficient fault data because it is difficult to obtain the real industrial data of machinery under fault conditions. To address these problems, this work proposes a new cross-domain bearing diagnosis framework based on transferable features and manifold embedded discriminative distribution adaption. First, it applies the maximal overlap discrete wavelet packet transform to process the vibration data and extract different statistics-based features. Then, to enhance the domain adaptation performance, it designs a transferability evaluation based on the adjusted rand index and maximum mean discrepancy to quantify the fault discriminability and domain invariance of the features. After that, it proposes a novel manifold embedded discriminative joint distribution adaptation method to perform cross-domain feature discriminative joint distribution alignment in a Grassmann manifold subspace. Finally, it utilizes a random forest classifier to train the cross-domain fault diagnosis model. To verify the performances of the proposed methods, extensive experiments have been conducted on two real rolling bearing datasets. The results demonstrate that the proposed methods can achieve the desirable diagnosis results and significantly outperform comparative classical transfer learning-based models when there is the class imbalance between source and target domains.
更多查看译文
关键词
Cross-domain fault diagnosis,distribution alignment,domain adaptation (DA),imbalance data,manifold subspace learning (MSL)
AI 理解论文
溯源树
样例
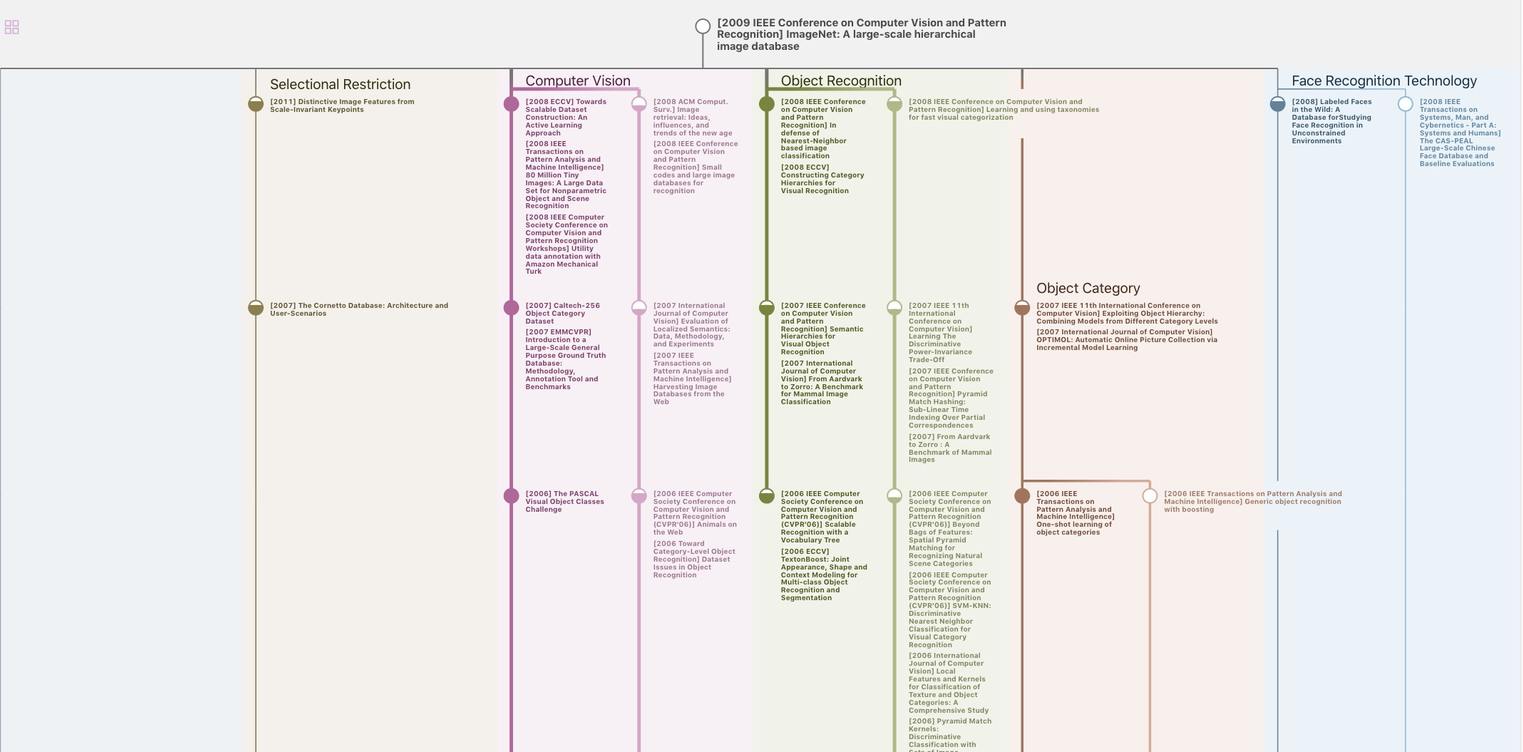
生成溯源树,研究论文发展脉络
Chat Paper
正在生成论文摘要