Learning self-target knowledge for few-shot segmentation
PATTERN RECOGNITION(2024)
摘要
Few-shot semantic segmentation uses a few annotated data of a specific class in the support set to segment the target of the same class in the query set. Most existing approaches fail to perform well when there are significant intra-class variances. This paper alleviates the problem by concentrating on mining the query image and using the support set as supplementary information. First, it proposes a Query Prototype Generation Module to generate a query foreground prototype from the query features. Specifically, we use both prototypelevel and pixel-level similarity matching to generate two complementary initial prototypes, which we then integrate to create a discriminative query foreground prototype. Second, we propose a Support Auxiliary Refinement Module to further guide the final precise prediction of the query image by leveraging the target category information of the support set through step -by-step mining. Specifically, we generate a query-support mixture prototype based on the support prototype representation obtained using the attention mechanism. Then we generate a support supplement prototype to complement the missing information by encoding over the foreground regions that the query-support mixture prototype fails to segment out. Extensive experiments on PASCAL-5 ' and COCO-20(iota). demonstrate that our model outperforms the prior works of few-shot segmentation.
更多查看译文
关键词
Few-shot segmentation,Two-level similarity matching,Step-by-step mining,Attention mechanism
AI 理解论文
溯源树
样例
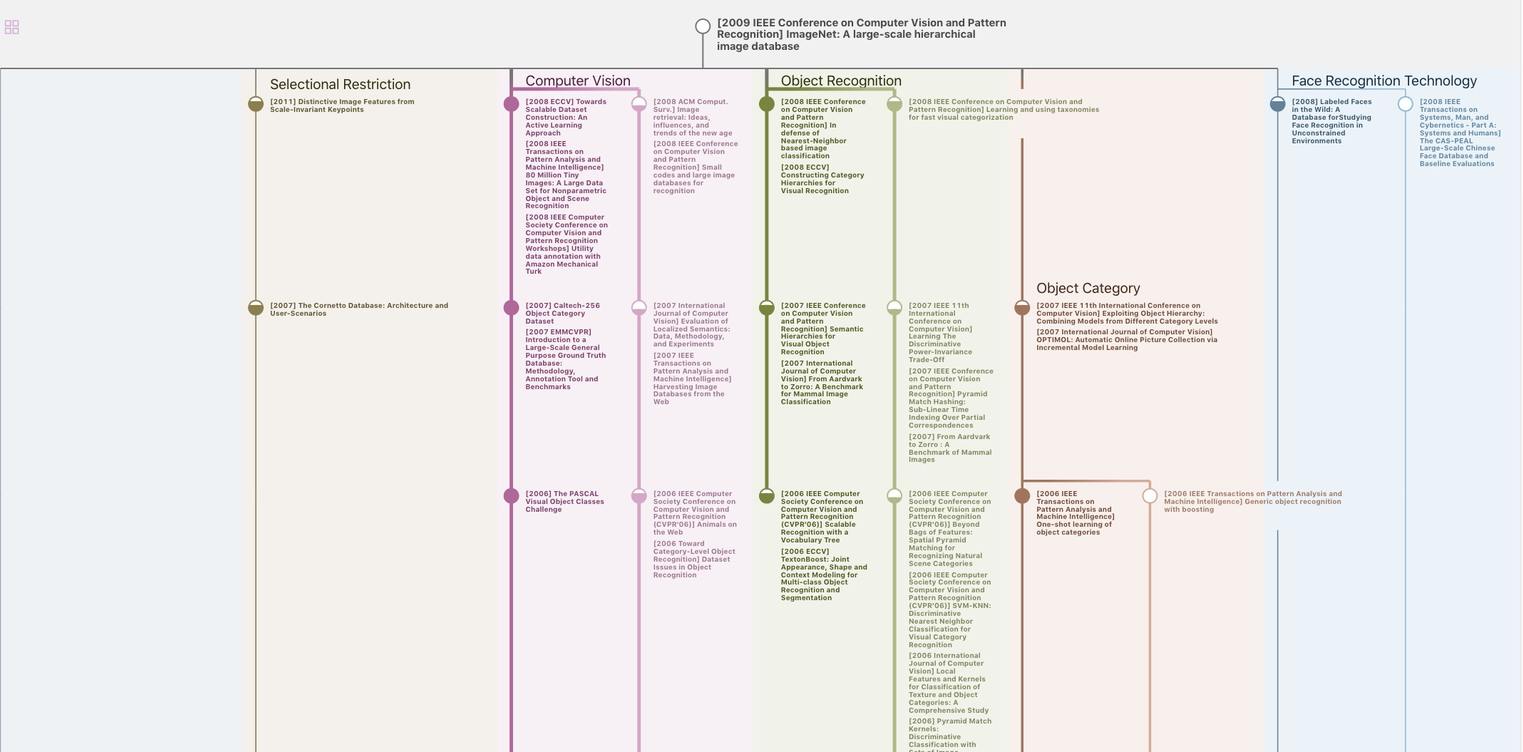
生成溯源树,研究论文发展脉络
Chat Paper
正在生成论文摘要