Abstract P6-04-10: Recurrence Prediction in Ductal Carcinoma In Situ (DCIS) Patients from Tissue Microarrays (TMAs)
Cancer Research(2023)
摘要
Abstract Recurrence Prediction in Ductal Carcinoma In Situ (DCIS) Patients Using Generative Adversarial Network (GAN) Augmented Deep Learning Model Background: DCIS patients have an excellent overall survival rate and over-treatment is always a cause for concern due to potential side-effects. Standard clinicopathological factors (age, growth pattern, tumor size, margin status and grade) have been shown to have limited value in predicting recurrence and segregation of high and low risk patients. Early and accurate recurrence prediction would facilitate a more aggressive treatment policy for high-risk patients (mastectomy or adjuvant radiation therapy), and simultaneously reduce over-treatment of low-risk patients. In this work, we have developed a deep learning (DL) classification framework that predicts recurrence in DCIS patients from Tissue microarrays (TMAs) hematoxylin and eosin (H&E) images using a generative adversarial network (GAN) augmented deep learning (DL) classification model. A GAN is a class of DL models, in which two adversarial neural networks, generator and discriminator contest among each other to generate high quality images. During the adversarial training process, the generator learns to synthesize realistic images similar to those in the training set while the discriminator learns to distinguish between real and generated images. In recent years, high quality medical images have been generated by GAN models. To the best of our knowledge, this is the first time a GAN model has been used to generate H&E images to train a DL classification model to predict recurrence in DCIS patients. Materials and methods: The cohort was comprised of 68 DCIS patients, aged between 35-89 years, lesion size of 5-90 mm, with a mix of low (15%), intermediate (35%) and 50% high grade cases. Patients were treated with mastectomy and/or a combination of lumpectomy, radiation and hormone therapy. TMAs were constructed from 2mm cores (1-3 cores per patient) in consultation with a breast pathologist to create hematoxylin and eosin (H&E) images for further analysis. The cohort was split into independent training (n=50 patients, 10 with recurrences at 5years) and validation groups (n=18 patients, 6 with recurrences at 5years). TMA (H&E) images were divided into smaller image patches of size 256x256 to train a GAN to generate image patches. A DL classification network (Resnet-Inception v2) was trained using TMA image patches and aggressive image patches generated by GAN to predict recurrence. The ability to generate synthetic image patches of aggressive lesions permitted training of a large DL classification network and predict recurrence in DCIS patients. Importantly, manual annotation was not necessary for the process. Results: The DL classification model trained with both TMA and GAN generated image patches predicted recurrence with an AUC of 0.87, sensitivity of 0.83 and specificity of 0.91 in the validation dataset. The DL classification model trained with image patches from TMAs only predicted recurrence with an AUC of 0.81. Conclusions: The use of a GAN model to generate H&E images circumvents the needs for a large cohort and accurate labor-intensive manual annotation of histopathological images, which is often required for training a large DL classification model. The use of GAN generated aggressive image patches during training significantly improves recurrence prediction accuracy of the DL classification model. Validation in independent larger cohorts is ongoing, and if successful, could provide a novel assay for risk prediction that does not waste precious tissue samples. Citation Format: Ghose Soumya, Yesim Gokmen-Polar, Sanghee Cho, Elizabeth McDonough, Cynthia Davis, Jhimli Mitra, Zhanpan Zhang, Fiona Ginty, Sunil Badve. Recurrence Prediction in Ductal Carcinoma In Situ (DCIS) Patients from Tissue Microarrays (TMAs) [abstract]. In: Proceedings of the 2022 San Antonio Breast Cancer Symposium; 2022 Dec 6-10; San Antonio, TX. Philadelphia (PA): AACR; Cancer Res 2023;83(5 Suppl):Abstract nr P6-04-10.
更多查看译文
关键词
ductal carcinoma,tissue microarrays,recurrence prediction
AI 理解论文
溯源树
样例
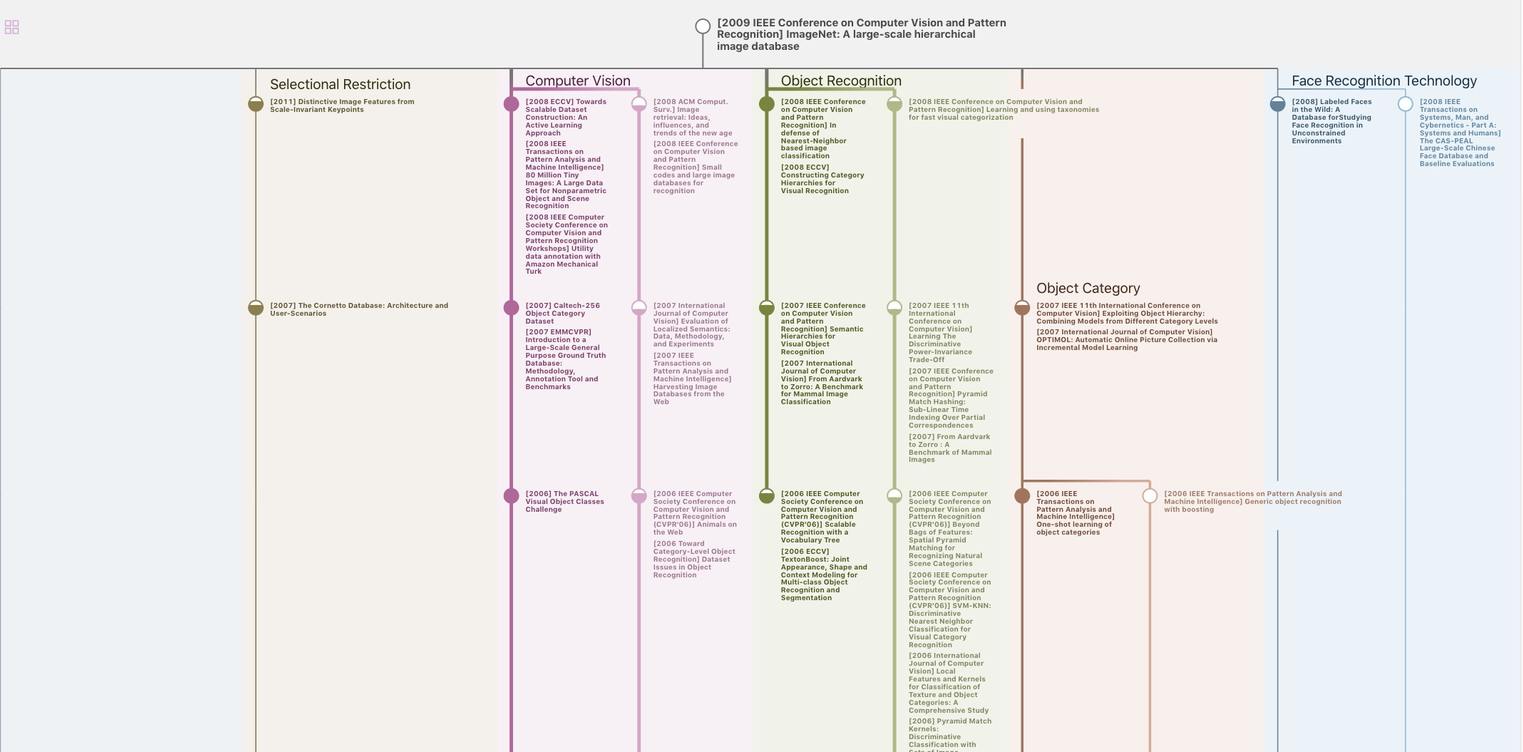
生成溯源树,研究论文发展脉络
Chat Paper
正在生成论文摘要