The NEON Ecological Forecasting Challenge
Authorea (Authorea)(2023)
摘要
The 21st century continues to be characterized by major changes to the environment and the ecosystem services upon which society depends. Anticipating and responding to these changes requires that scientists explicitly forecast future conditions in real time (Dietze et al. 2018). Ecological forecasting, like weather and epidemiological forecasting, involves integrating data and models to generate quantitative predictions of the future state of ecological systems before observations are collected. The iterative cycle of creating forecasts, evaluating them with new observations, updating the models, and then making new forecasts has the potential to accelerate learning across many ecological subdisciplines. This cycle builds on openly available data, often published soon after collection, as is increasingly common in ecological observatory networks, such as the National Ecological Observatory Network (NEON). To accelerate improvements in ecological forecasting, we designed and launched the NEON Ecological Forecasting Challenge (hereafter, “Challenge”) (Figure 1), an open platform for the ecological and data science communities to forecast NEON data before they are collected. The ecological forecasting community is interested in using forecasts to advance theory (Lewis et al. 2023) and in translating forecasts for natural resource management (Enquist et al. 2017). By analyzing a catalog of forecasts developed for a range of ecological systems, spatiotemporal scales, and environmental gradients, scientists can begin to address fundamental questions in ecology. The Ecological Forecasting Initiative Research Coordination Network (EFI-RCN) – funded by the US National Science Foundation (NSF) – invites the broad ecology community to help build this catalog by forecasting NEON data. NEON is a powerful platform to support such a challenge because it provides standardized data with reported uncertainties that span a range of environmental conditions and levels of biological organization across terrestrial and freshwater systems in the US. The Challenge was designed on input from academic, government, and private sectors through workshops and working groups. We call it a “Challenge” because, despite its similarities to data science competitions (Makridakis et al. 2021), we are empowering the community to do more than just submit forecasts – we are also collaboratively developing software, training materials, and best practices. In May 2020, we launched the Challenge's design at a virtual conference with over 200 attendees (Peters and Thomas 2021). Attendees prioritized five forecasting “themes” that draw on NEON data, address open science questions, and have potential to support decision making for resource management: (1) freshwater temperature, dissolved oxygen, and chlorophyll-a; (2) terrestrial carbon fluxes and evapotranspiration; (3) plant canopy phenology; (4) tick populations; and (5) beetle communities. Themes were identified at the virtual meeting, after which smaller design teams developed detailed theme-specific protocols. The protocols defined the timing of forecast submissions (when and how often forecasts are due) and forecast horizons (how far forecasts extend into the future). With these protocols in place, team participants developed code to convert NEON data products into standardized time-series that are ready for modeling and evaluation. Simultaneously, the EFI-RCN standards working group was assembled to define the format of forecast submissions and metadata across themes (Dietze et al. 2023). Likewise, the EFI-RCN steering committee worked with each design team to ensure the developed protocols were consistent across themes (eg all forecasts quantify uncertainty in predictions). To support the Challenge, we created software and workflows for provisioning model inputs and processing model outputs that leverage modern cloud storage and computing (Figure 1). We also developed software to improve the efficiency of downloading NEON data while facilitating the analysis of data that exceed computer memory (Boettiger et al. 2021). Other end-user tools convert NEON data into easy-to-use time-series, process submitted forecasts, score probabilistic forecasts, and visualize submissions. Every day we are automatically downloading, processing, and sharing NOAA numerical ensemble weather forecasts for all NEON sites, eliminating the need for users to have to do so themselves. All of these technologies are open source and generalized to be applicable beyond the Challenge. We hope everyone who is interested in participating in the Challenge feels empowered to submit forecasts as individuals or teams. To reduce barriers, we have curated resources (documentation, workflow code examples, videos) to train teams in the computational skills needed for model development and submission. Participants can contribute forecasts from any NEON site or theme, using any type of modeling framework (eg empirical, process-based, machine learning). We also created a set of simple forecasts to serve as benchmarks for submissions. The Challenge provides a foundation for training in ecological modeling and forecasting. Teaching ecological modeling in undergraduate classrooms improves students’ systems-level thinking skills and quantitative literacy (Carey et al. 2020). Similarly, integrating ecological forecasting into classrooms expands students’ understanding of complex ecological concepts (Moore et al. 2022). Challenge submissions are an ideal project for undergraduate and graduate students in courses and workshops. The rapid, iterative feedback inherent to the Challenge design inspires student engagement and improvement – submissions are evaluated daily as new submissions are accepted and as new data become available. The Challenge can help transform ecology into a more predictive science while providing feedback to NEON that improves data generation and delivery. Forecasting is part of NEON's mission, and the Challenge empowers the ecological forecasting community to lead the charge in accomplishing that mission. However, the Challenge extends well beyond NEON, engaging researchers who have not previously considered forecasting or who are seeking new data analysis and modeling approaches. It is a testing ground for developing novel forecasting techniques that can be rapidly applied across broad environmental gradients, with products and outcomes that have the potential to support decision making in environmental management and conservation. It fosters the creation of a more quantitative workforce and can be an inspiration and blueprint for other environmental observatory networks across the globe. In 2021, we launched a beta round of the Challenge that resulted in 2516 forecast submissions contributed by 54 different teams, ranging in composition from students to researchers at private companies. At that stage of the Challenge, contributions were critical to refining protocols and to identifying needed software and educational materials. Today, the Challenge is fully operational and actively seeking contributions. If you are interested in becoming involved or learning more, see www.neon4cast.org (Thomas et al. 2021). We aim for the Challenge to further enable new forecast innovations, provide valuable training, and spark broad engagement among ecologists. The EFI-RCN is supported by NSF (DEB-1926388) with computational support provided by NSF-funded Jetstream2 (OAC-2005506). NEON is a program sponsored by NSF and operated under cooperative agreement by Battelle. This material is based in part upon work supported by the NSF through NEON. Any use of trade, firm, or product names is for descriptive purposes only and does not imply endorsement by the US Government. WebPanel 1 Please note: The publisher is not responsible for the content or functionality of any supporting information supplied by the authors. Any queries (other than missing content) should be directed to the corresponding author for the article.
更多查看译文
关键词
ecological forecasting challenge
AI 理解论文
溯源树
样例
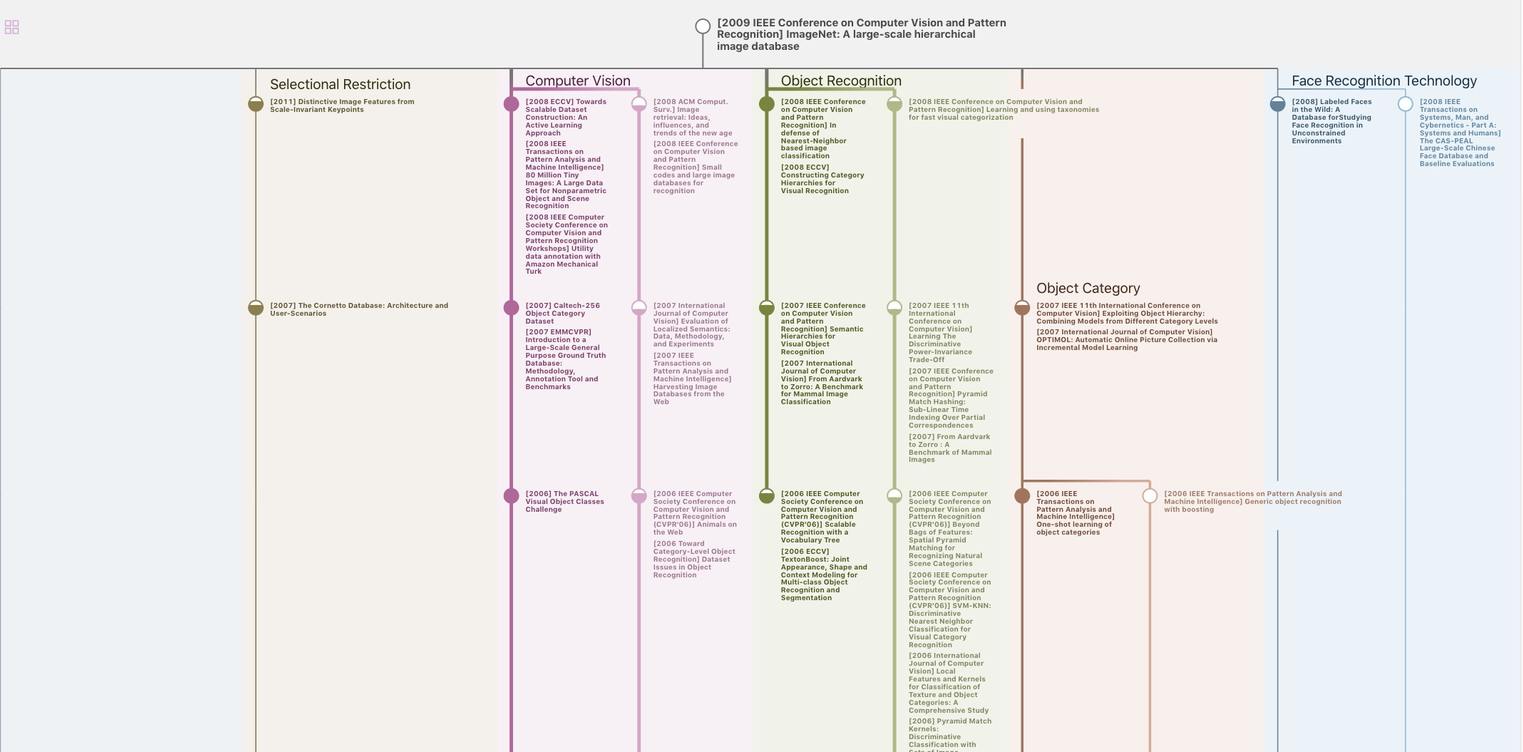
生成溯源树,研究论文发展脉络
Chat Paper
正在生成论文摘要