Machine learning-based prediction of diabetes risk by combining exposome and electrocardiographic predictors
18th International Symposium on Medical Information Processing and Analysis(2023)
摘要
Diabetes is a high-burden non-communicable disease affecting more than 532 million people worldwide and resulting in a range of life-threatening comorbidities. Pre-identifying high-risk individuals and applying preventive actions will likely reduce the prevalence and health consequences of diabetes. Under this context, we developed and evaluated the first predictive model of diabetes risk that combines both electrocardiography (ECG) and exposome predictors. A comprehensive list of ECG signals and exposome variables were extracted from the UK Biobank, then used to build and compare a set of machine learning models for diabetes risk prediction. Random Forest combining ECGs and exposome variables achieved an 0.82 +/- 0.03 AUC when predicting diabetes risk. This integrative model outperformed separate models based on exposome factors or ECG signals alone. These preliminary results indicate the potential of low-cost machine learning models trained from ECG and exposome data to predict diabetes years before its onset.
更多查看译文
关键词
diabetes, risk prediction, ECG signals, exposome factors, machine learning
AI 理解论文
溯源树
样例
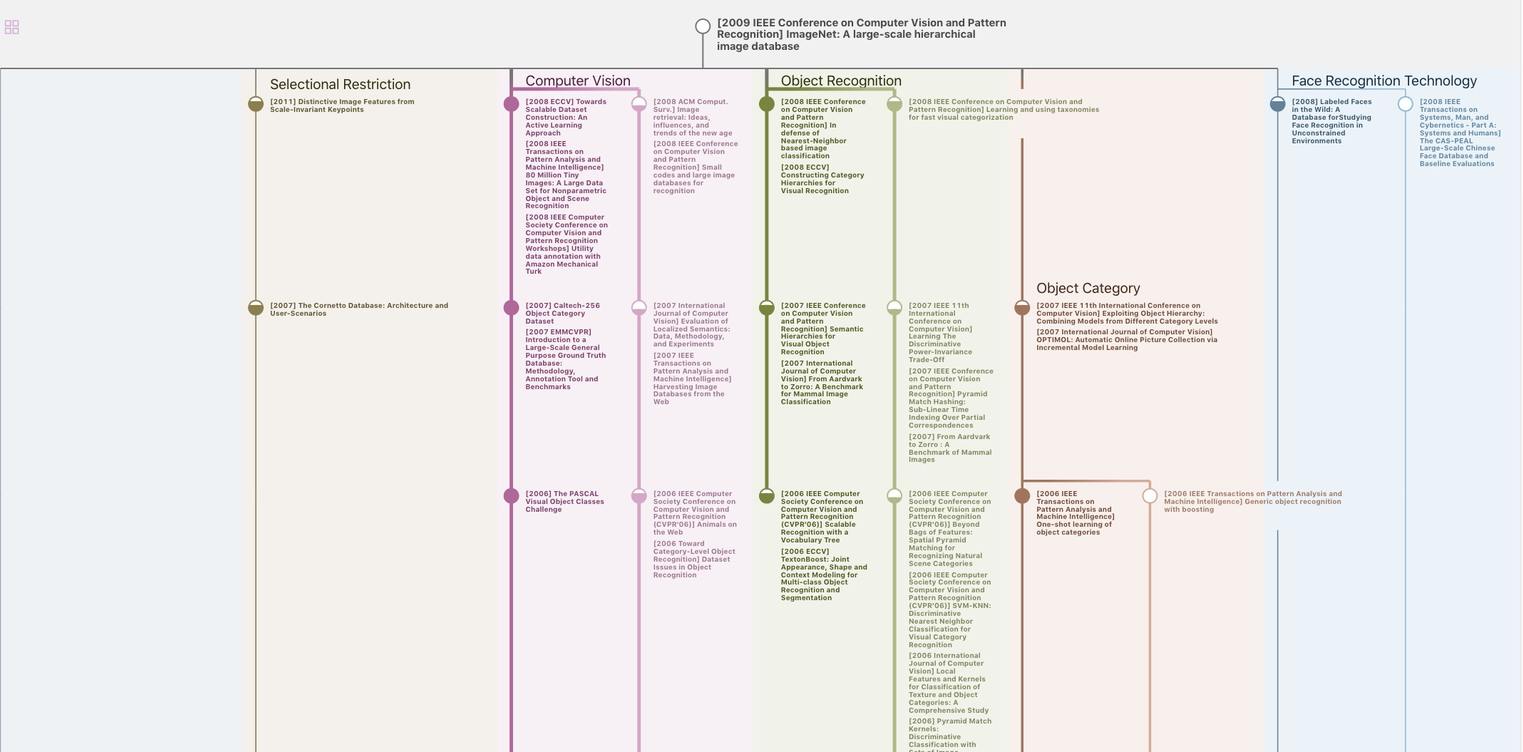
生成溯源树,研究论文发展脉络
Chat Paper
正在生成论文摘要