BANDT: A Border-Aware Network With Deformable Transformers for Visual Tracking
IEEE Transactions on Consumer Electronics(2023)
摘要
Siamese trackers that predict a tracking box over a regular grid of feature maps based on the sliding-window paradigm have recently drawn widespread attention in the tracking community. However, the sliding-window paradigm lacks the exploration to characterize the explicit border features of the tracking object for accurate box prediction. Furthermore, Siamese trackers do not use an online learning process to improve the model's discriminative power and a deformable transformer to explore the rich temporal context from the successive frames. To overcome these issues, we propose a border-aware network with deformable transformers (BANDT), which contains classification and regression branches for tracking. The proposed BANDT tracker introduces a border-alignment operation to capture the "border features" from the extreme points of the object's border to improve the point features of the sliding-window paradigm. The BANDT tracker implements a deformable transformer consisting of an encoder and a decoder to enhance the target representations within a discriminative online learning framework. Specifically, the introduced encoder of the deformable transformer enhances the target representations, while the decoder highlights the potential tracking location. The proposed tracker achieves state-of-the-art performance on seven public datasets. In particular, it achieves 69.6% overlap success and 90.0% precision scores on the UAV123 dataset.
更多查看译文
关键词
Visual tracking,border features,deformable transformers,online learning,anchor-free tracker
AI 理解论文
溯源树
样例
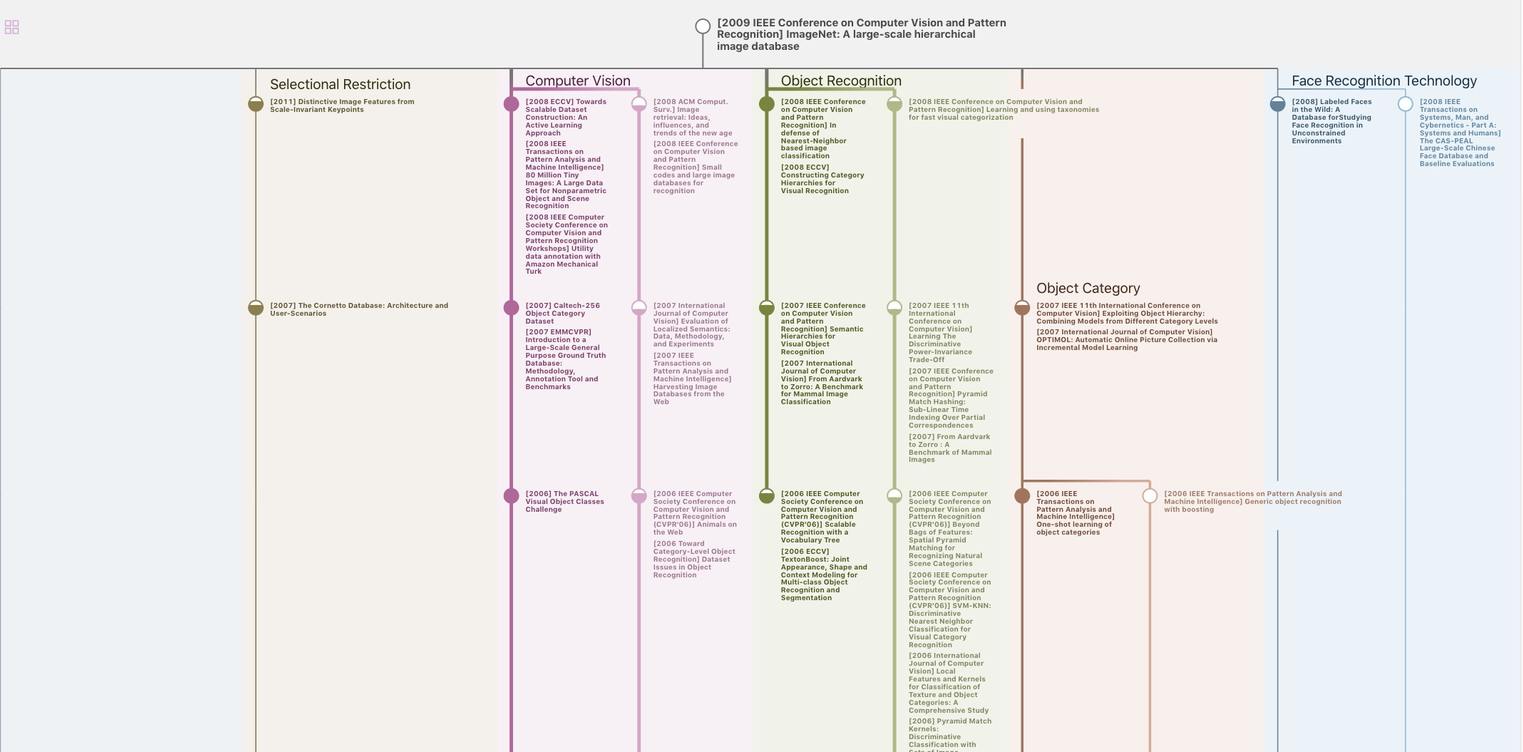
生成溯源树,研究论文发展脉络
Chat Paper
正在生成论文摘要