Novel extended NI-MWMOTE-based fault diagnosis method for data-limited and noise-imbalanced scenarios
EXPERT SYSTEMS WITH APPLICATIONS(2024)
摘要
Under real-world conditions, faulty samples of key components (e.g., bearings and cutting tools, etc.) are typi-cally limited and sparse. Additionally, their historical data is characterized by time-series and imbalance char-acteristics. In other words, the training samples are not only limited and noisy, but also exhibit both within-class and between-class imbalance. These factors present significant challenges in the realm of fault monitoring modeling. To tackle these challenges, this paper presents an innovative fault diagnosis method rooted in the extended NI-MWMOTE and LS-SVM. NI-MWMOTE stands as an advanced noise-immunity majority weighted minority oversampling technique, originally introduced in our prior research, and it has exhibited exceptional competitiveness in noisy imbalanced benchmark datasets. It champions an adaptive noise processing strategy leveraging the distribution characteristics of noisy imbalanced data and the essence of machine learning. Spe-cifically, it employs Euclidean distance and neighbor density to differentiate between spurious noise and true noise, and it determines the optimal processing strategy based on misclassification error and iteration. Furthermore, it employs unsupervised aggregative hierarchical clustering, misclassification error, and majority -weighted minority oversampling in a collaborative manner to address both within-class and between-class imbalanced problems. The primary contribution of our paper lies in the context of the monitoring scenario mentioned above. We have expanded the hyper-parameter range of NI-MWMOTE, corrected and optimized its built-in noise function to enhance the interpretability of the model, and successfully applied it in conjunction with LS-SVM to this particular setting. Notably, this marks the pioneering endeavor within our established knowledge sphere into the domain of tool wear state monitoring. The results suggest that, when compared to 11 well-known algorithms, our framework demonstrates significant competitiveness in real-world scenarios char-acterized under data-limited and noise-imbalanced scenarios for bearings and cutting tools fault diagnosis. This establishes a solid theoretical and practical foundation for similar scenarios.
更多查看译文
关键词
Extended NI-MWMOTE,Limited data,Noisy and imbalanced data,Bearings and cutting tools,Fault diagnosis
AI 理解论文
溯源树
样例
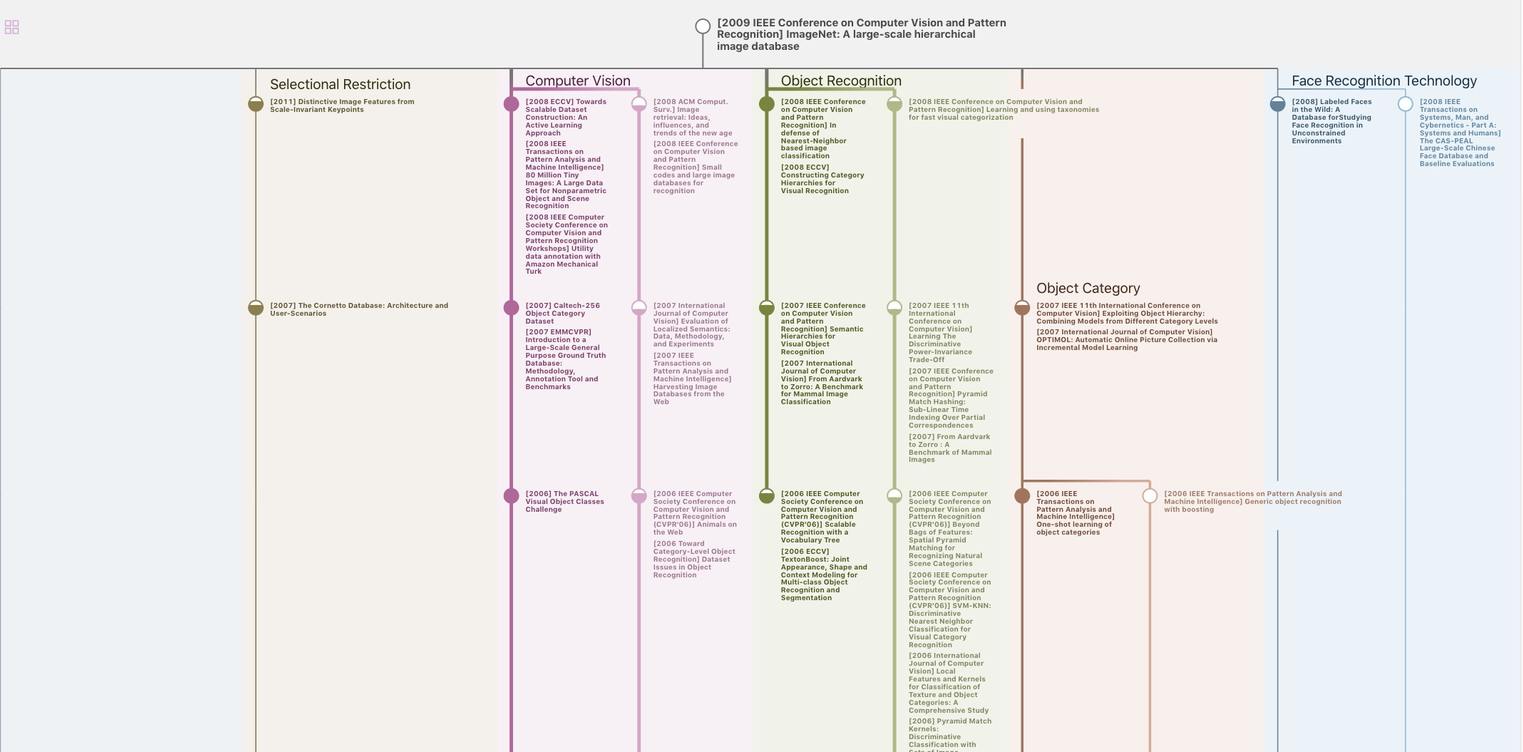
生成溯源树,研究论文发展脉络
Chat Paper
正在生成论文摘要