Data mining for prediction and interpretation of bacterial population behavior in food
Frontiers in Food Science and Technology(2022)
摘要
Although bacterial population behavior has been investigated in a variety of foods in the past 40 years, it is difficult to obtain desired information from the mere juxtaposition of experimental data. We predicted the changes in the number of bacteria and visualize the effects of pH, aw, and temperature using a data mining approach. Population growth and inactivation data on eight pathogenic and food spoilage bacteria under 5,025 environmental conditions were obtained from the ComBase database (www.combase.cc), including 15 food categories, and temperatures ranging from 0°C to 25°C. The eXtreme gradient boosting tree was used to predict population behavior. The root mean square error of the observed and predicted values was 1.23 log CFU/g. The data mining model extracted the growth inhibition for the investigated bacteria against aw, temperature, and pH using the SHapley Additive eXplanations value. A data mining approach provides information concerning bacterial population behavior and how food ecosystems affect bacterial growth and inactivation.
更多查看译文
关键词
databases,predictive microbiology,data-driven methods,SHapley Additive,population behavior
AI 理解论文
溯源树
样例
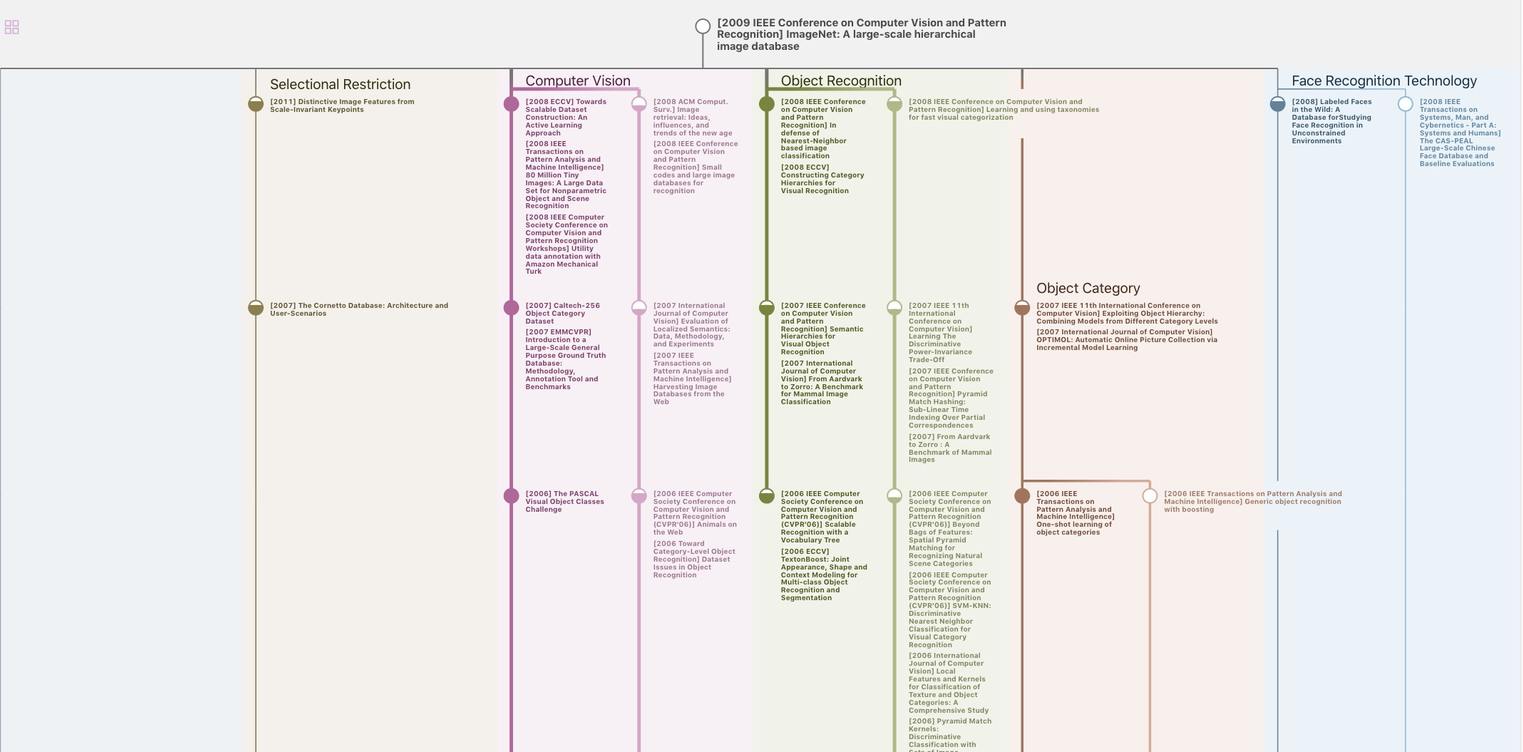
生成溯源树,研究论文发展脉络
Chat Paper
正在生成论文摘要