An in-memory computing architecture based on a duplex 2D material structure for in-situ machine learning
Research Square (Research Square)(2023)
摘要
Abstract The growing computational demand in artificial intelligence (AI) calls for hardware solutions that are capable of in-situ machine learning, where both training and inference are performed by edge computation. This not only requires extremely energy-efficient architecture (such as in-memory computing, IMC) but also memory hardware with tunable properties to simultaneously meet the demand for training and inference. Here, we report a duplex device structure based on ferroelectric field-effect transistor (FeFET) and atomically thin MoS2 channel and realize a universal IMC architecture for in-situ learning. By exploiting the tunability of ferroelectric energy landscape, the duplex building block demonstrates overall excellent performance in endurance (>1013), retention (>10 years), speed (4.8 ns) and energy consumption (22.7 fJ/(bit·μm2)). We implemented a hardware neural network using arrays of two-transistor-one-duplex-FeFET (2T1D) cells and achieved 99.86% accuracy in non-linear localization task with in-situ trained weights. Simulations show that the proposed device architecture could achieve the same level of performance as graphics processing unit under notably improved energy efficiency. Our device core can be combined with silicon circuitry through three-dimensional heterogeneous integration to give a hardware solution toward general edge intelligence (EI).
更多查看译文
关键词
duplex 2d material structure,computing,architecture,in-memory,in-situ
AI 理解论文
溯源树
样例
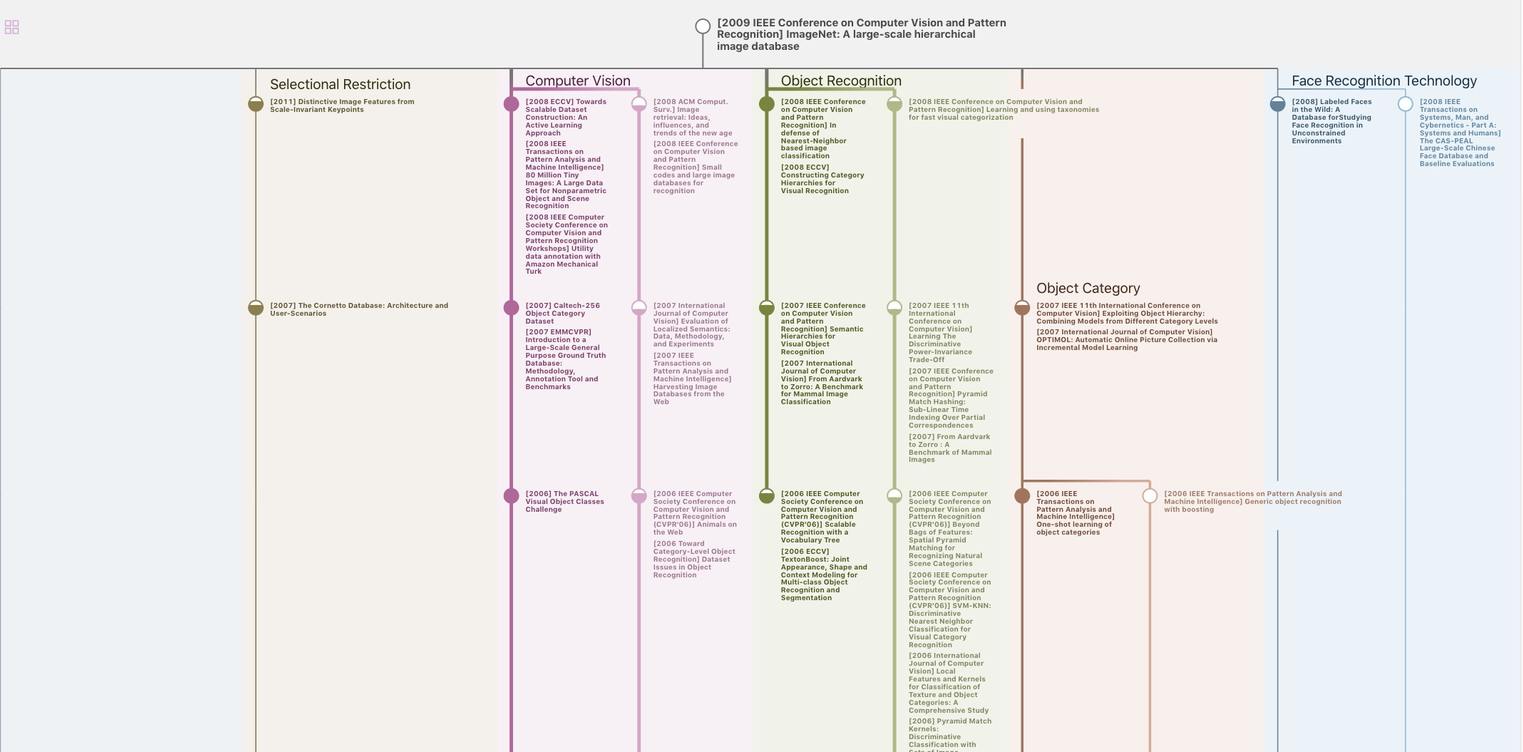
生成溯源树,研究论文发展脉络
Chat Paper
正在生成论文摘要