Improving predictions of land-atmosphere interactions based on a hybrid data assimilation and machine learning method
crossref(2022)
摘要
Abstract. The energy and moisture exchange between the land surface and atmospheric boundary layer plays a critical role in regional climate simulations. This paper implemented a hybrid data assimilation and machine learning framework (DA-ML method) into the Weather Research and Forecasting (WRF) model to optimize surface soil and vegetation conditions. The hybrid method can integrate remotely sensed leaf area index (LAI), multi-source soil moisture (SM) observations, and land surface models (LSMs) to accurately describe land surface states and fluxes. The performance of the hybrid method on the regional climate was evaluated in the Heihe River Basin (HRB), the second largest endorheic river basin in Northwest China. The findings indicate that the DA-ML method improved the estimation of evapotranspiration (ET) and generated a spatial distribution consistent with the ML-based watershed ET (ETMap). The WRF simulations overestimated (underestimated) the air temperature (specific humidity) in the vegetated areas of the HRB. In contrast, the estimated air temperature and specific humidity from WRF (DA-ML) agree well with the observations, especially in the midstream oasis. The DA-ML framework enhanced oasis-desert interactions by improving the soil and vegetation characteristics. The wetting and cooling effects and wind shield effects of the oasis were enhanced by the DA-ML. The wetting and cooling effect of the oasis can transfer water vapor to the surrounding desert, which benefits the oasis-desert ecosystem. The results show that the wetting and cooling effects only negligibly changed the local precipitation in the midstream oasis. However, upstream of the HRB, the integration of LAI and SM will induce water vapor intensification and promote precipitation, particularly on windward slopes.
更多查看译文
AI 理解论文
溯源树
样例
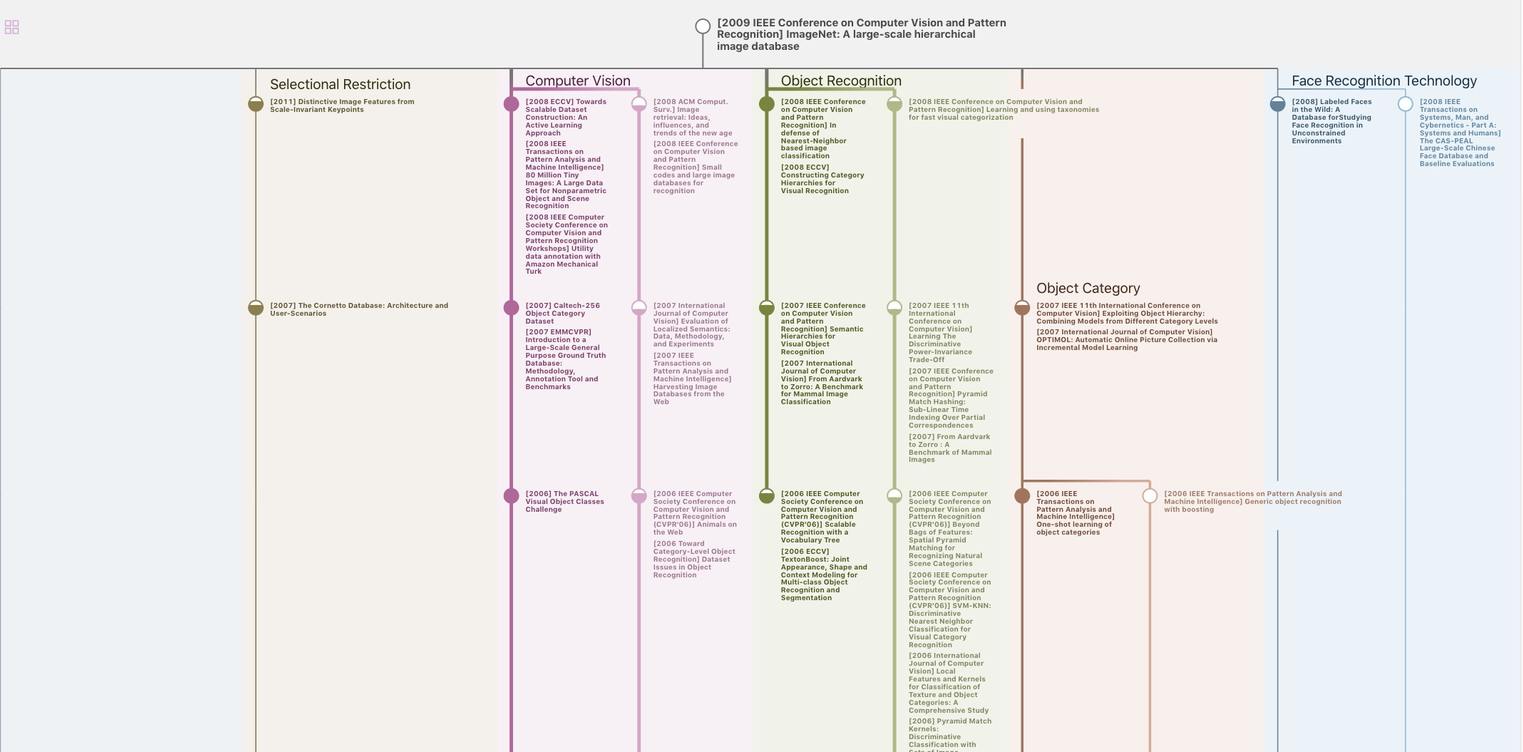
生成溯源树,研究论文发展脉络
Chat Paper
正在生成论文摘要