An adaptive and dynamical neural network for machine remaining useful life prediction
IEEE TRANSACTIONS ON INDUSTRIAL INFORMATICS(2023)
摘要
Recently, many neural networks have been proposed for machine remaining useful life (RUL) prediction. However, most network architectures of existing approaches are fixed. Since the sequential information depends on the input data and distributes differently, these fixed networks which cannot be dynamically adjusted according to the input data, may not be able to capture these sequential information well, resulting sub-optimal performances. To mitigate this issue, we propose an adaptive and dynamical neural network (AdaNet), which can dynamically adjust its architecture according to the input data. Neural network is generally determined by kernel size, depth and channel size. In this paper, we aim to enable our proposed AdaNet to adjust its kernel size and channel size dynamically. Firstly, we explore to adapt the deformable convolution to time series data, which allows the convolutional kernel to change according to the feature map. With this deformable convolution, the convolutional kernels in AdaNet become adjustable, which is beneficial to fully exploit the sequential information in time series data, leading to accurate RUL prediction. Additionally, a channel selection module is devised, which can selectively activate the feature channel according to the input, further improving the performance of our AdaNet. Extensive experiments have been carried out on the C-MAPSS dataset, demonstrating that our proposed AdaNet achieves state-of-the-art performances.
更多查看译文
关键词
Remaining useful life prediction,deep learning,neural network,time series
AI 理解论文
溯源树
样例
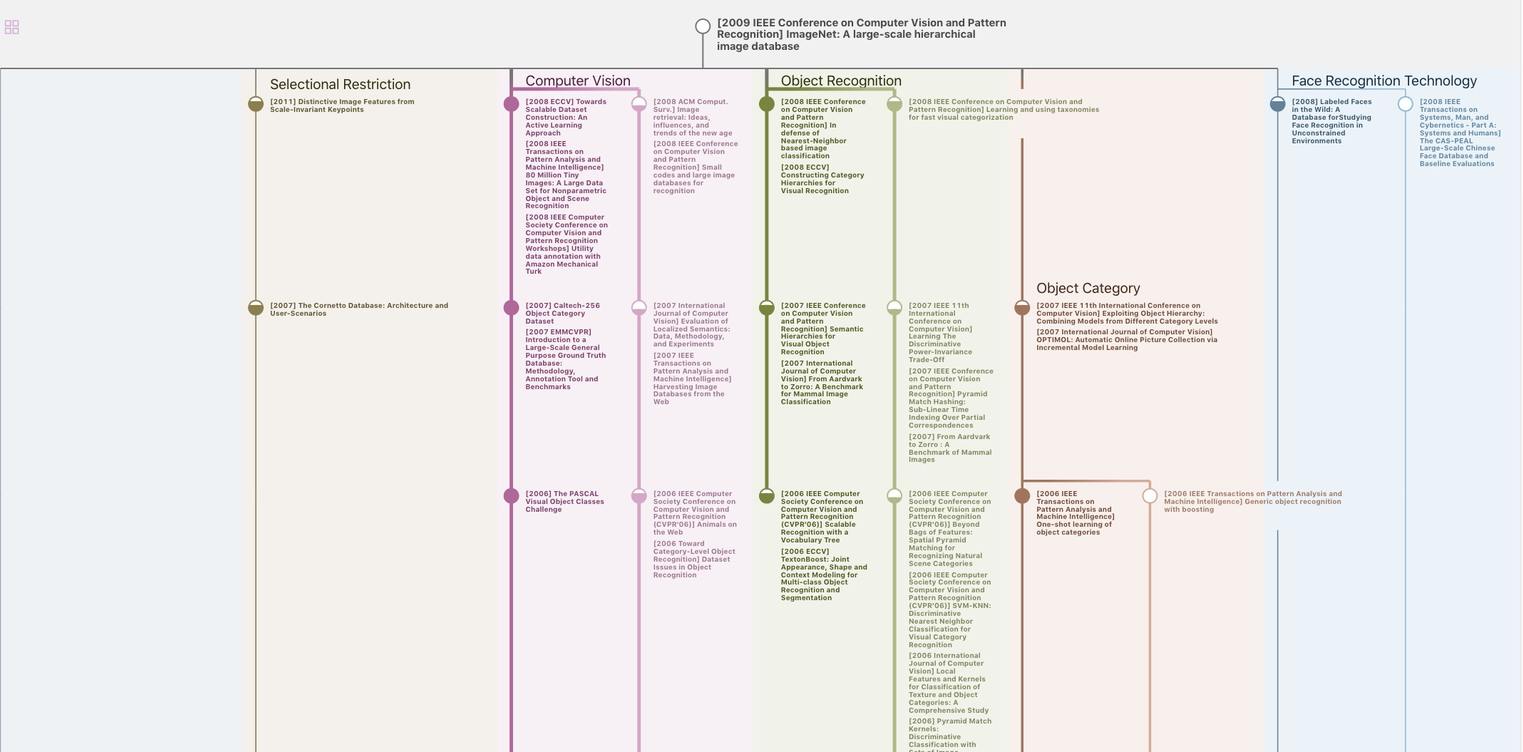
生成溯源树,研究论文发展脉络
Chat Paper
正在生成论文摘要