Bayesian risk prediction model for colorectal cancer mortality through integration of clinicopathologic and genomic data
npj Precision Oncology(2023)
摘要
Routine tumor-node-metastasis (TNM) staging of colorectal cancer is imperfect in predicting survival due to tumor pathobiological heterogeneity and imprecise assessment of tumor spread. We leveraged Bayesian additive regression trees (BART), a statistical learning technique, to comprehensively analyze patient-specific tumor characteristics for the improvement of prognostic prediction. Of 75 clinicopathologic, immune, microbial, and genomic variables in 815 stage II–III patients within two U.S.-wide prospective cohort studies, the BART risk model identified seven stable survival predictors. Risk stratifications (low risk, intermediate risk, and high risk) based on model-predicted survival were statistically significant (hazard ratios 0.19–0.45, vs. higher risk; P < 0.0001) and could be externally validated using The Cancer Genome Atlas (TCGA) data (P = 0.0004). BART demonstrated model flexibility, interpretability, and comparable or superior performance to other machine-learning models. Integrated bioinformatic analyses using BART with tumor-specific factors can robustly stratify colorectal cancer patients into prognostic groups and be readily applied to clinical oncology practice.
更多查看译文
关键词
colorectal cancer mortality,bayesian risk prediction model,colorectal cancer
AI 理解论文
溯源树
样例
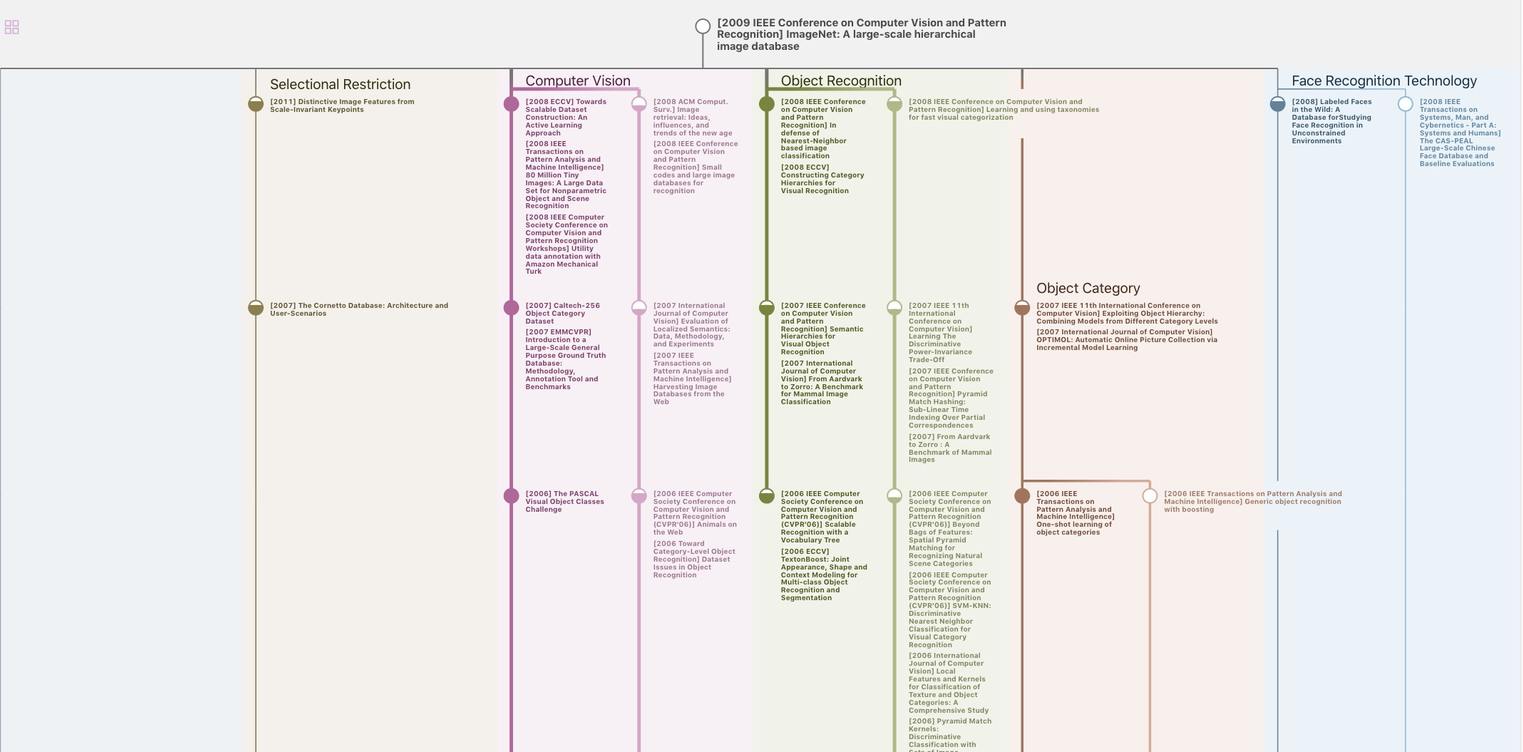
生成溯源树,研究论文发展脉络
Chat Paper
正在生成论文摘要