Deep Learning Depends on Segregated Dendritic Compartments and Spike Frequency Adaptation
IEEE Transactions on Instrumentation and Measurement(2023)
摘要
Deep learning mimics the function of the biological nervous system via adopting strategies motivated by neurophysiology. However, integrating biophysical characteristics to make deep learning more brain-like remains challenging. To investigate whether the more realistic simulated neurons could make deep learning more realistic from the biology perspective, we propose a three-layer multicompartment deep learning network with spike frequency adaptation (SFA). We utilize the segregated apical dendrites in the hidden layer to implement the credit assignment and coordinate the distribution of information flow. The introduction of SFA serves as the teaching signal. The results show that the apical dendrites separate the feedforward and feedback signals and instruct the credit assignment problem. The apical dendrites diverse the firing types of target neurons and further decrease the firing rate of nontarget neurons via switching their firing types from the tonic firing type to predominantly burst firing. Furthermore, the expression of SFA in the nontarget neurons further amplifies the difference between the firing rate of target and nontarget signals. This work provides new insight into a deep learning network with more biophysical neurons and is a meaningful supplementary for deep learning.
更多查看译文
关键词
Apical dendrite,deep learning,multicompartment,spike frequency adaptation (SFA),teaching signal
AI 理解论文
溯源树
样例
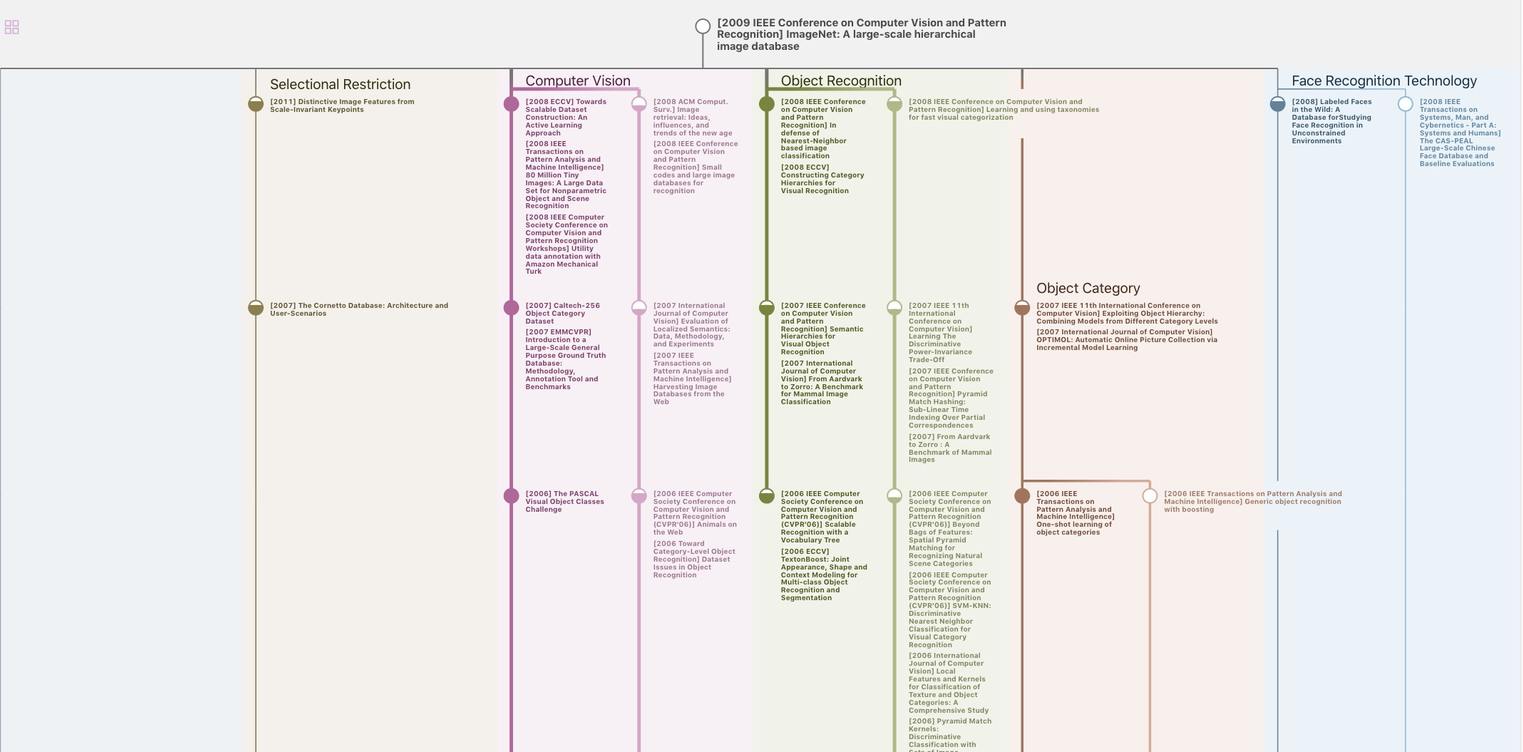
生成溯源树,研究论文发展脉络
Chat Paper
正在生成论文摘要