Prediction of ENSO using multivariable deep learning
Atmospheric and Oceanic Science Letters(2023)
摘要
A novel multivariable prediction system based on a deep learning (DL) algorithm, i.e., the residual neural network and pure observations, was developed to improve the prediction of the El Niño–Southern Oscillation (ENSO). Optimal predictors are automatically determined using the maximal information for spatial filtering and the Taylor diagram criteria, enabling the best prediction skills at lead times of eight months compared with most operational prediction models. The hindcast skill for the most challenging decade (2011–18) outperforms the multi-model ensemble operational forecasts. At the six-month lead, the correlation (COEF) skill of the DL model reaches 0.82 with a normalized root-mean-square error (RMSE) of 0.58 °C, which is significantly better than the average multi-model performance (COEF = 0.70 and RMSE = 0.73°C). DL prediction can effectively alleviate the long-standing spring predictability barrier problem. The automatically selected optimal precursors can explain well the typical ENSO evolution driven by both tropical dynamics and extratropical impacts.
更多查看译文
关键词
enso,deep learning,prediction
AI 理解论文
溯源树
样例
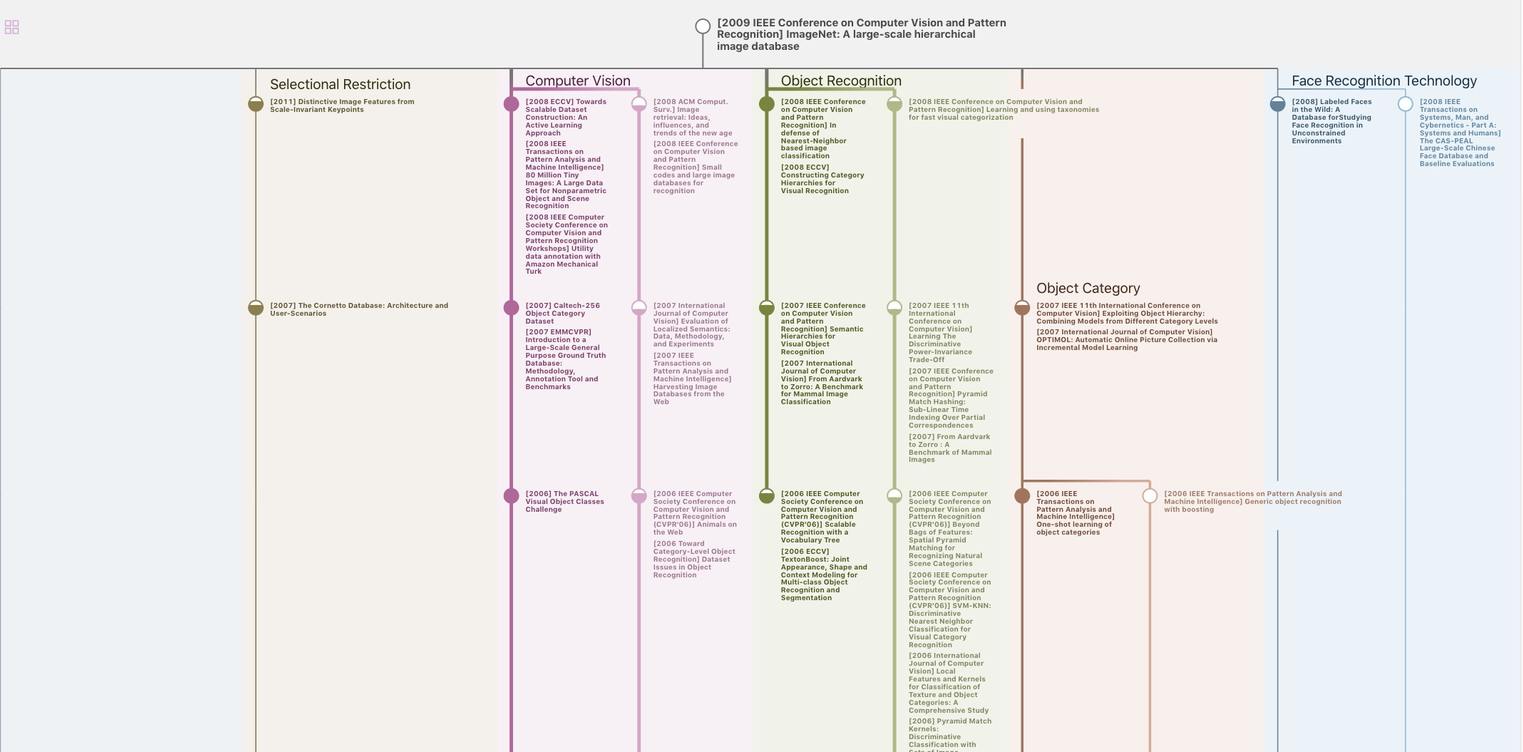
生成溯源树,研究论文发展脉络
Chat Paper
正在生成论文摘要