Dynamic Dispatching of Converter Gas in Steel Industry based on Dual Critic Proximal Policy Optimization
2022 China Automation Congress (CAC)(2022)
摘要
An optimal scheduling for converter gas is the key to realize energy savings and intelligent manufacturing in steel industry. Considering the traditional predictive optimization approaches are usually affected by prediction accuracy, reinforcement learning (RL) technology is adopted here to obtain dynamic dispatching strategies of the converter gas system. In view of the data of steelmaking process are with intermittent fluctuation characteristics, it would be a difficult task to establish a learning model simply using time domain data, which may thereby affect the RL process. In this study, a feature extraction method is employed to construct the state space of RL using frequency domain information. In order to improve the convergence of the policy network in RL, an improved dual critic proximal policy optimization (PPO) is further proposed, which combines the dual critic mechanism into the PPO algorithms. In order to verify the effectiveness of the proposed method, practical data come from the converter gas system of a domestic steel company are utilized to conduct our experiments. The results show that the proposed method achieves a better convergence performance and a lower economic cost compared with expert experience and other classic RL approaches.
更多查看译文
关键词
converter gas scheduling,proximal policy optimization,dual critic network,feature extraction
AI 理解论文
溯源树
样例
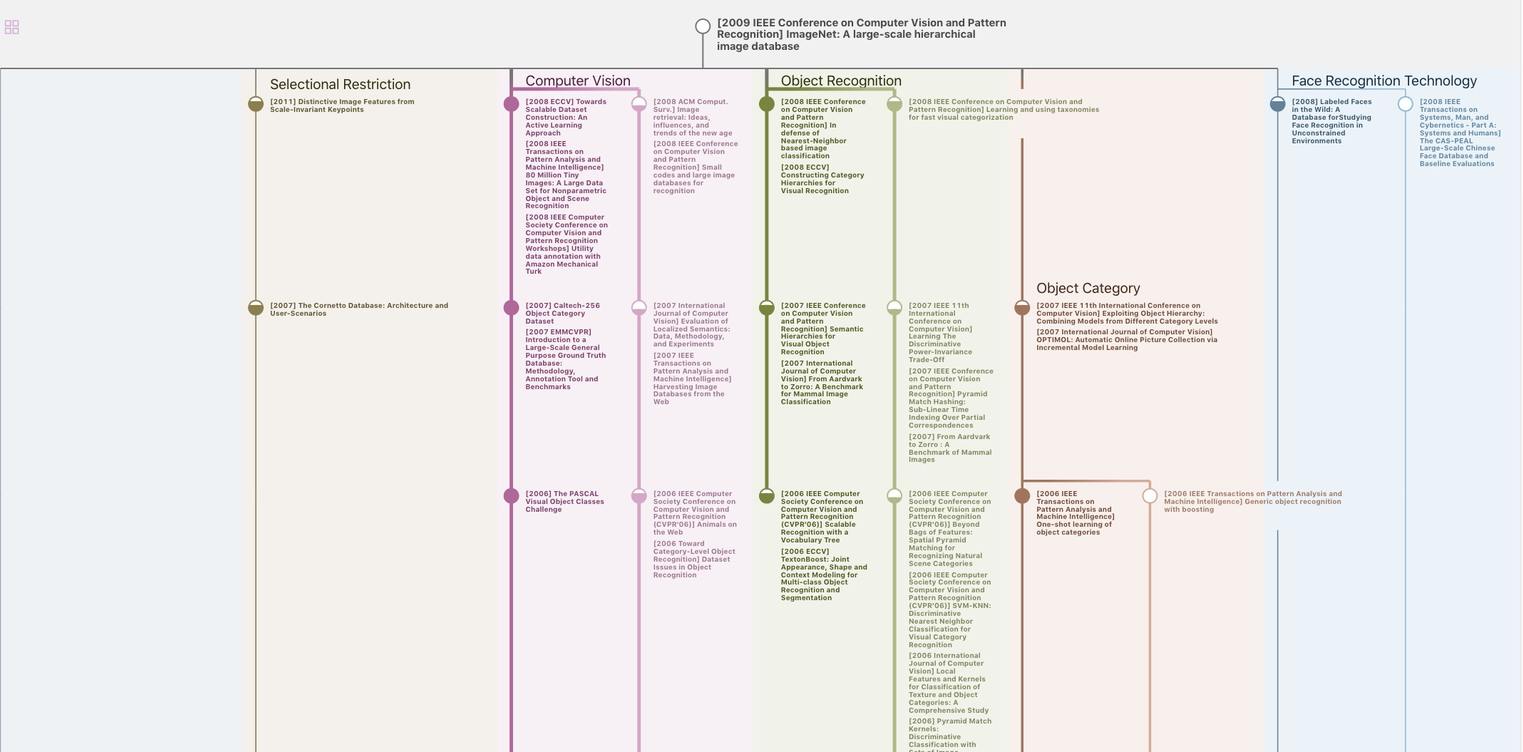
生成溯源树,研究论文发展脉络
Chat Paper
正在生成论文摘要