Introduction to Information Theory
Stochastic Methods for Modeling and Predicting Complex Dynamical SystemsSynthesis Lectures on Mathematics & Statistics(2023)
摘要
One major challenge in modeling, understanding, and predicting complex systems is quantifying the associated uncertainty due to the intrinsic turbulent nature or the external stochastic forcing. Information theory is a powerful tool to assess the uncertainty, model error, and prediction skill of the underlying complex systems. It also facilitates the development of useful criteria for building statistically accurate reduced-order models and measuring the predictability limit of nature. This chapter includes an introduction to information theory. The main focus will be on the properties and the applications of three widely used information measurements that are directly applicable to quantifying the uncertainty and model error. They are Shannon’s entropy, relative entropy, and mutual information. The maximum entropy principle, which provides an unbiased criterion for developing approximate PDFs and coarse-grained statistical models, will also be presented with concrete examples. In addition, the relationship between the information criteria and the path-wise measurements will be developed, which illustrates the necessity of adopting the former in studying many complex turbulent systems.
更多查看译文
关键词
information theory,introduction
AI 理解论文
溯源树
样例
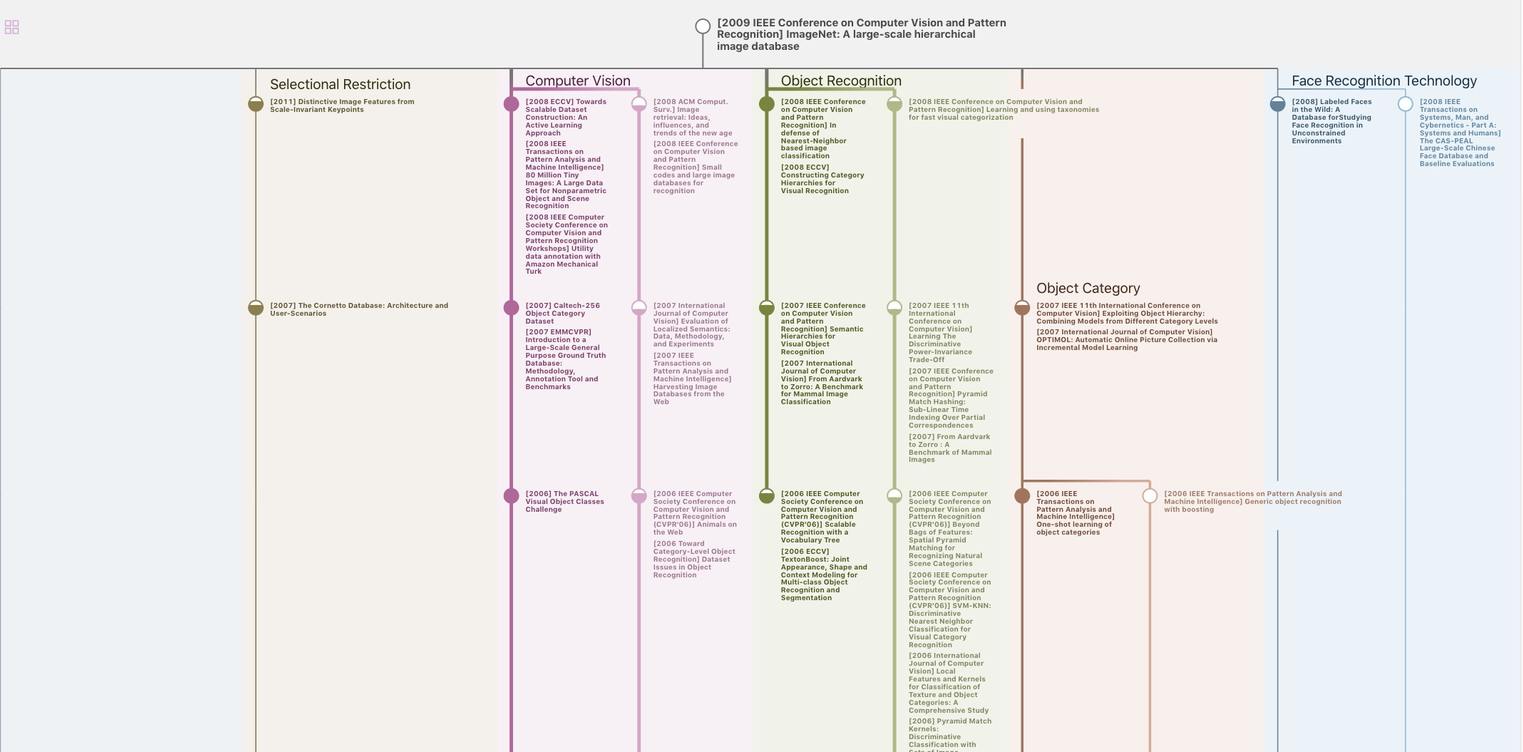
生成溯源树,研究论文发展脉络
Chat Paper
正在生成论文摘要