Knowledge Guided Representation Learning and Causal Structure Learning in Soil Science
CoRR(2023)
摘要
An improved understanding of soil can enable more sustainable land-use practices. Nevertheless, soil is called a complex, living medium due to the complex interaction of different soil processes that limit our understanding of soil. Process-based models and analyzing observed data provide two avenues for improving our understanding of soil processes. Collecting observed data is cost-prohibitive but reflects real-world behavior, while process-based models can be used to generate ample synthetic data which may not be representative of reality. We propose a framework, knowledge-guided representation learning, and causal structure learning (KGRCL), to accelerate scientific discoveries in soil science. The framework improves representation learning for simulated soil processes via conditional distribution matching with observed soil processes. Simultaneously, the framework leverages both observed and simulated data to learn a causal structure among the soil processes. The learned causal graph is more representative of ground truth than other graphs generated from other causal discovery methods. Furthermore, the learned causal graph is leveraged in a supervised learning setup to predict the impact of fertilizer use and changing weather on soil carbon. We present the results in five different locations to show the improvement in the prediction performance in out-of-sample and few-shots setting.
更多查看译文
关键词
causal structure learning,soil science,knowledge
AI 理解论文
溯源树
样例
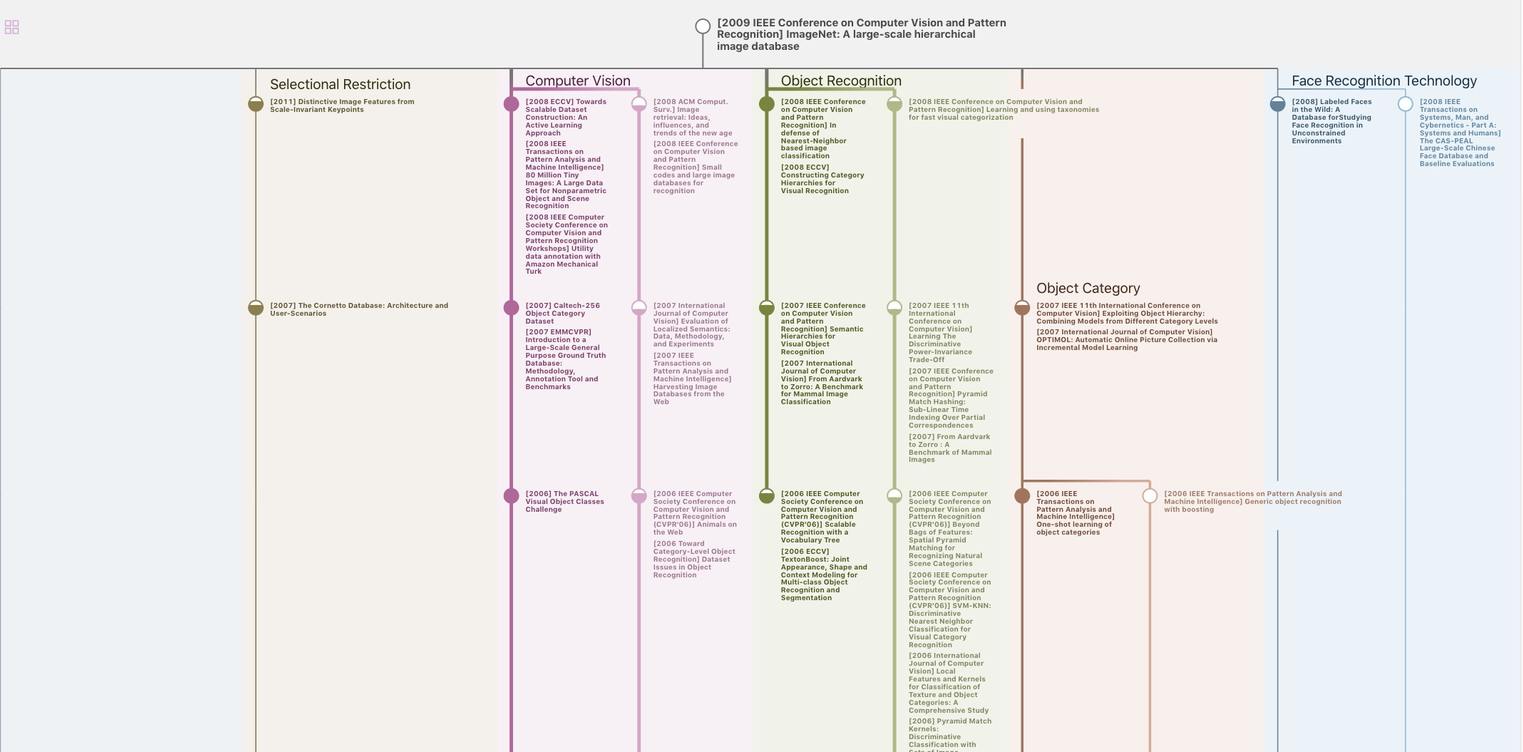
生成溯源树,研究论文发展脉络
Chat Paper
正在生成论文摘要