Reward-Free Curricula for Training Robust World Models
ICLR 2024(2023)
摘要
There has been a recent surge of interest in developing generally-capable agents that can adapt to new tasks without additional training in the environment. Learning world models from reward-free exploration is a promising approach, and enables policies to be trained using imagined experience for new tasks. Achieving a general agent requires robustness across different environments. However, different environments may require different amounts of data to learn a suitable world model. In this work, we address the problem of efficiently learning robust world models in the reward-free setting. As a measure of robustness, we consider the minimax regret objective. We show that the minimax regret objective can be connected to minimising the maximum error in the world model across environments. This informs our algorithm, WAKER: Weighted Acquisition of Knowledge across Environments for Robustness. WAKER selects environments for data collection based on the estimated error of the world model for each environment. Our experiments demonstrate that WAKER outperforms naive domain randomisation, resulting in improved robustness, efficiency, and generalisation.
更多查看译文
关键词
curricula,model-based reinforcement learning,world models,reward-free reinforcement learning,robustness,unsupervised environment design
AI 理解论文
溯源树
样例
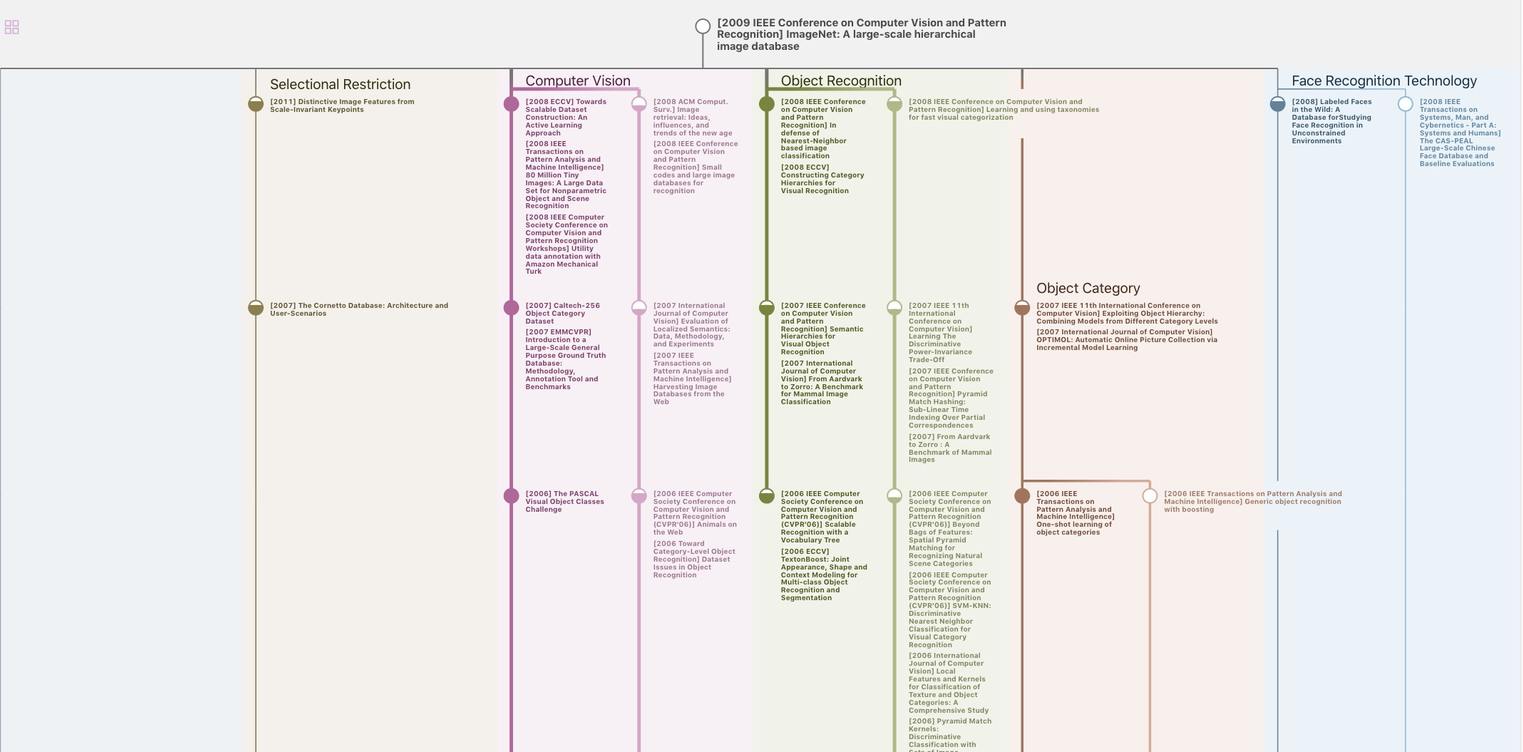
生成溯源树,研究论文发展脉络
Chat Paper
正在生成论文摘要