Dis-AE: Multi-domain & Multi-task Generalisation on Real-World Clinical Data
CoRR(2023)
摘要
Clinical data is often affected by clinically irrelevant factors such as discrepancies between measurement devices or differing processing methods between sites. In the field of machine learning (ML), these factors are known as domains and the distribution differences they cause in the data are known as domain shifts. ML models trained using data from one domain often perform poorly when applied to data from another domain, potentially leading to wrong predictions. As such, developing machine learning models that can generalise well across multiple domains is a challenging yet essential task in the successful application of ML in clinical practice. In this paper, we propose a novel disentangled autoencoder (Dis-AE) neural network architecture that can learn domain-invariant data representations for multi-label classification of medical measurements even when the data is influenced by multiple interacting domain shifts at once. The model utilises adversarial training to produce data representations from which the domain can no longer be determined. We evaluate the model's domain generalisation capabilities on synthetic datasets and full blood count (FBC) data from blood donors as well as primary and secondary care patients, showing that Dis-AE improves model generalisation on multiple domains simultaneously while preserving clinically relevant information.
更多查看译文
关键词
clinical
AI 理解论文
溯源树
样例
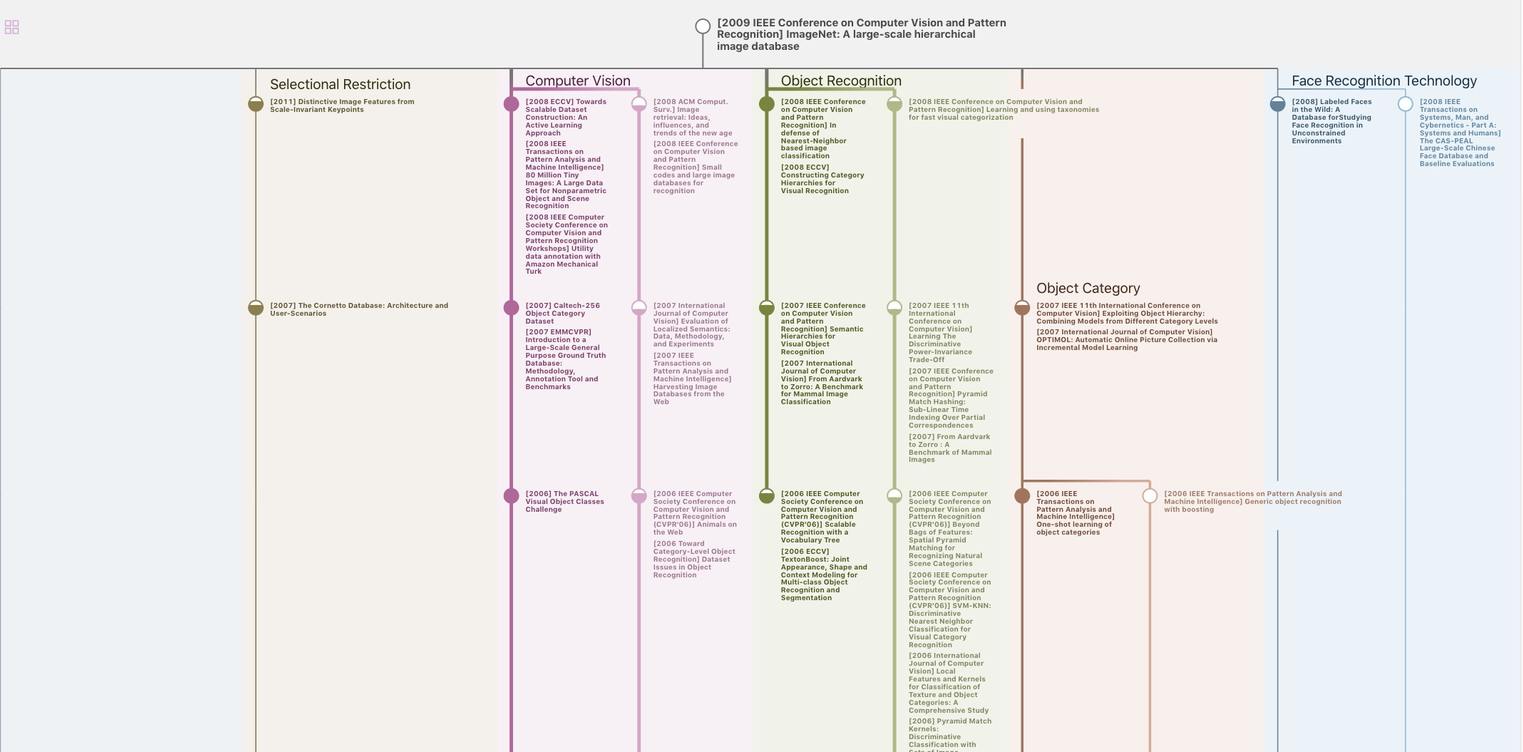
生成溯源树,研究论文发展脉络
Chat Paper
正在生成论文摘要