CoverHunter: Cover Song Identification with Refined Attention and Alignments
CoRR(2023)
摘要
Abstract: Cover song identification (CSI) focuses on finding the same music with different versions in reference anchors given a query track. In this paper, we propose a novel system named CoverHunter that overcomes the shortcomings of existing detection schemes by exploring richer features with refined attention and alignments. CoverHunter contains three key modules: 1) A convolution-augmented transformer (i.e., Conformer) structure that captures both local and global feature interactions in contrast to previous methods mainly relying on convolutional neural networks; 2) An attention-based time pooling module that further exploits the attention in the time dimension; 3) A novel coarse-to-fine training scheme that first trains a network to roughly align the song chunks and then refines the network by training on the aligned chunks. At the same time, we also summarize some important training tricks used in our system that help achieve better results. Experiments on several standard CSI datasets show that our method significantly improves over state-of-the-art methods with an embedding size of 128 (2.3% on SHS100K-TEST and 17.7% on DaTacos).
更多查看译文
关键词
Cover Song Identification, Contrastive Learning, Chunk Alignment, Conformer, Coarse-to-Fine Training
AI 理解论文
溯源树
样例
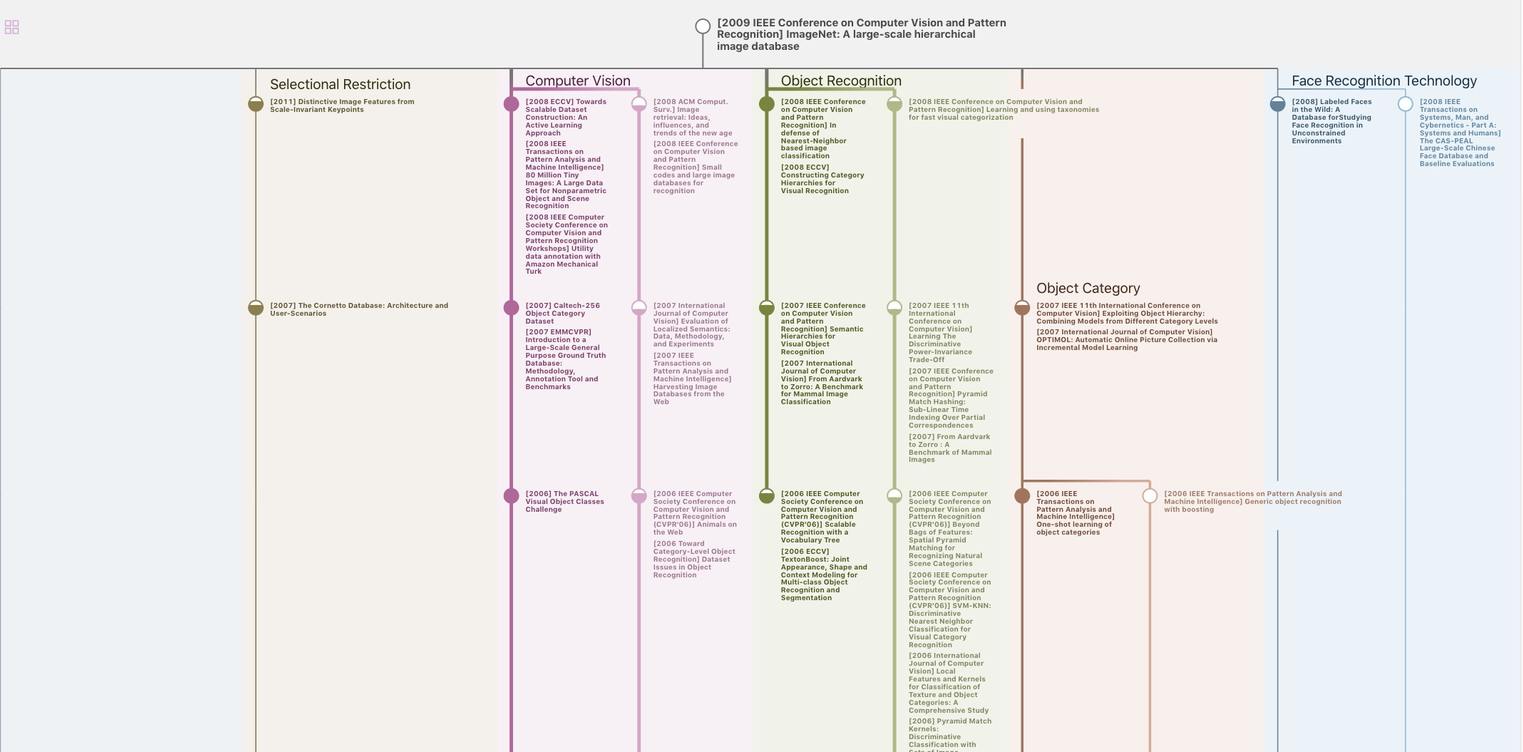
生成溯源树,研究论文发展脉络
Chat Paper
正在生成论文摘要