Graph Convolution Based Efficient Re-Ranking for Visual Retrieval
IEEE TRANSACTIONS ON MULTIMEDIA(2024)
摘要
Visual retrieval tasks such as image retrieval and person re-identification (Re-ID) aim at effectively and thoroughly searching images with similar content or the same identity. After obtaining retrieved examples, re-ranking is a widely adopted post-processing step to reorder and improve the initial retrieval results by making use of the contextual information from semantically neighboring samples. Prevailing re-ranking approaches update distance metrics and mostly rely on inefficient crosscheck set comparison operations while computing expanded neighbors based distances. In this work, we present an efficient re-ranking method which refines initial retrieval results by updating features. Specifically, we reformulate re-ranking based on Graph Convolution Networks (GCN) and propose a novel Graph Convolution based Re-ranking (GCR) for visual retrieval tasks via feature propagation. To accelerate computation for large-scale retrieval, a decentralized and synchronous feature propagation algorithm which supports parallel or distributed computing is introduced. In particular, the plain GCR is extended for cross-camera retrieval and an improved feature propagation formulation is presented to leverage affinity relationships across different cameras. It is also extended for video-based retrieval, and Graph Convolution based Re-ranking for Video (GCRV) is proposed by mathematically deriving a novel profile vector generation method for the tracklet. Without bells and whistles, the proposed approaches achieve state-of-the-art performances on seven benchmark datasets from three different tasks, i.e., image retrieval, person Re-ID and video-based person Re-ID.
更多查看译文
关键词
Task analysis,Visualization,Image retrieval,Representation learning,Convolution,Costs,Training,Visual retrieval,re-ranking,person re-identification,video-based person re-identification
AI 理解论文
溯源树
样例
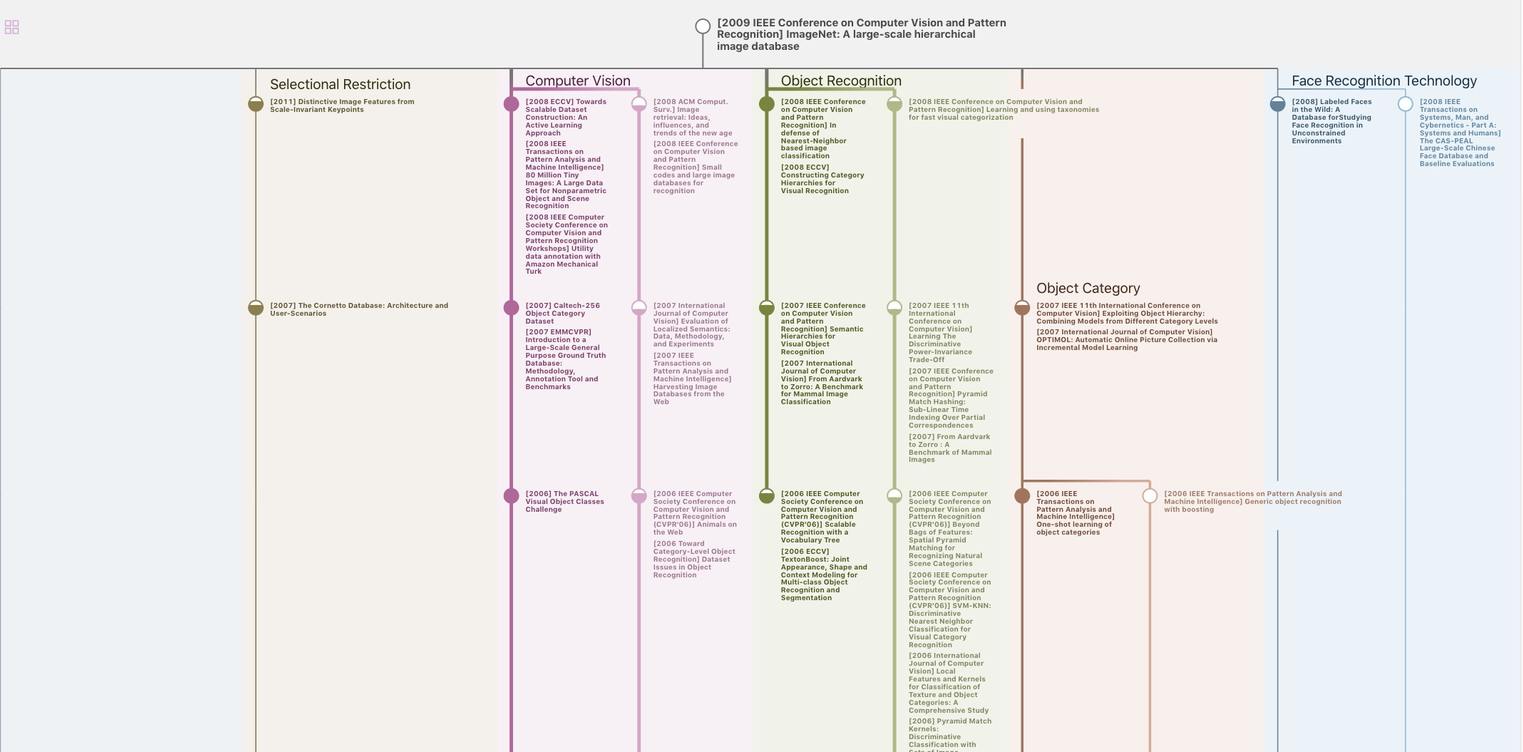
生成溯源树,研究论文发展脉络
Chat Paper
正在生成论文摘要