Batches Stabilize the Minimum Norm Risk in High Dimensional Overparameterized Linear Regression
CoRR(2023)
摘要
Learning algorithms that divide the data into batches are prevalent in many machine-learning applications, typically offering useful trade-offs between computational efficiency and performance. In this paper, we examine the benefits of batch-partitioning through the lens of a minimum-norm overparameterized linear regression model with isotropic Gaussian features. We suggest a natural small-batch version of the minimum-norm estimator, and derive an upper bound on its quadratic risk, showing it is inversely proportional to the noise level as well as to the overparameterization ratio, for the optimal choice of batch size. In contrast to minimum-norm, our estimator admits a stable risk behavior that is monotonically increasing in the overparameterization ratio, eliminating both the blowup at the interpolation point and the double-descent phenomenon. Interestingly, we observe that this implicit regularization offered by the batch partition is partially explained by feature overlap between the batches. Our bound is derived via a novel combination of techniques, in particular normal approximation in the Wasserstein metric of noisy projections over random subspaces.
更多查看译文
关键词
minimum norm risk
AI 理解论文
溯源树
样例
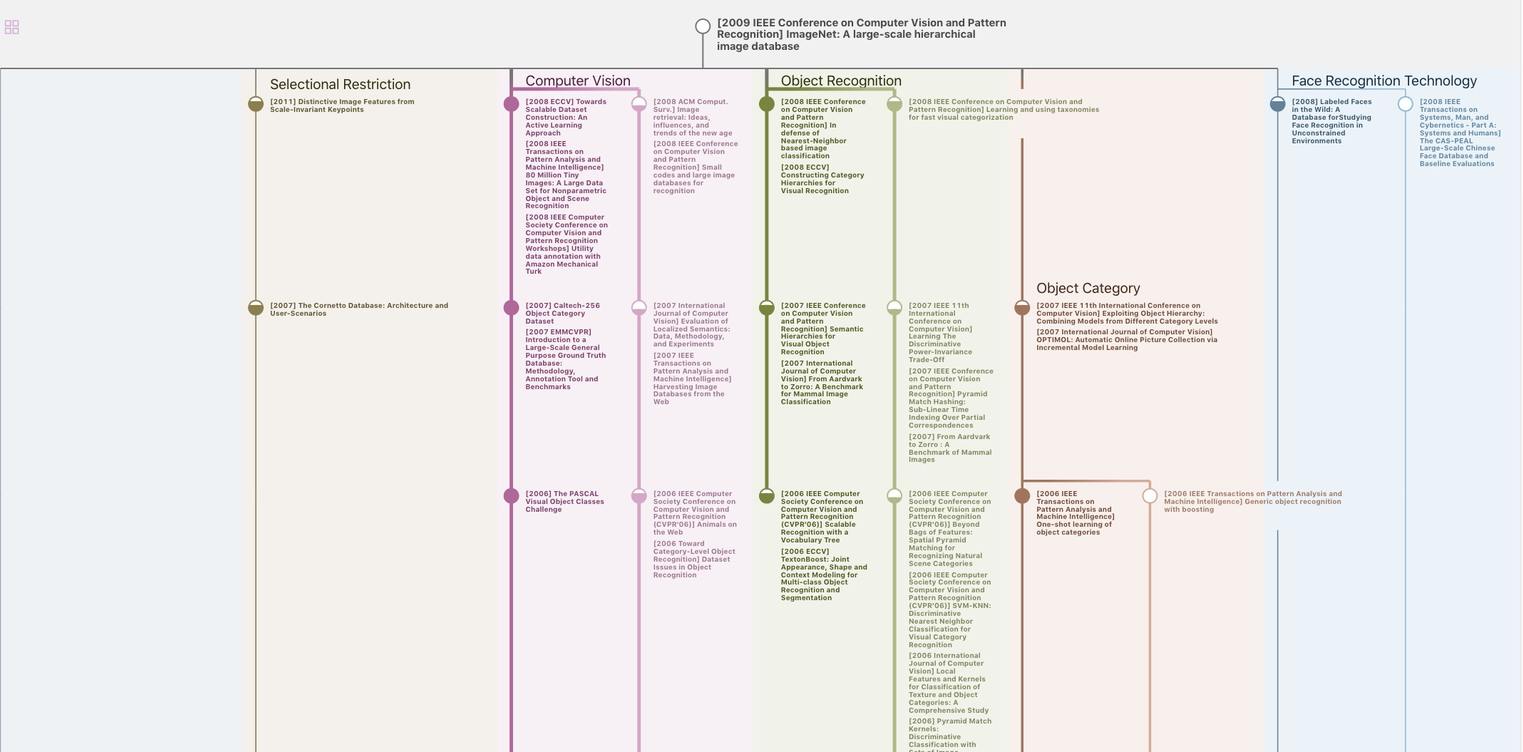
生成溯源树,研究论文发展脉络
Chat Paper
正在生成论文摘要